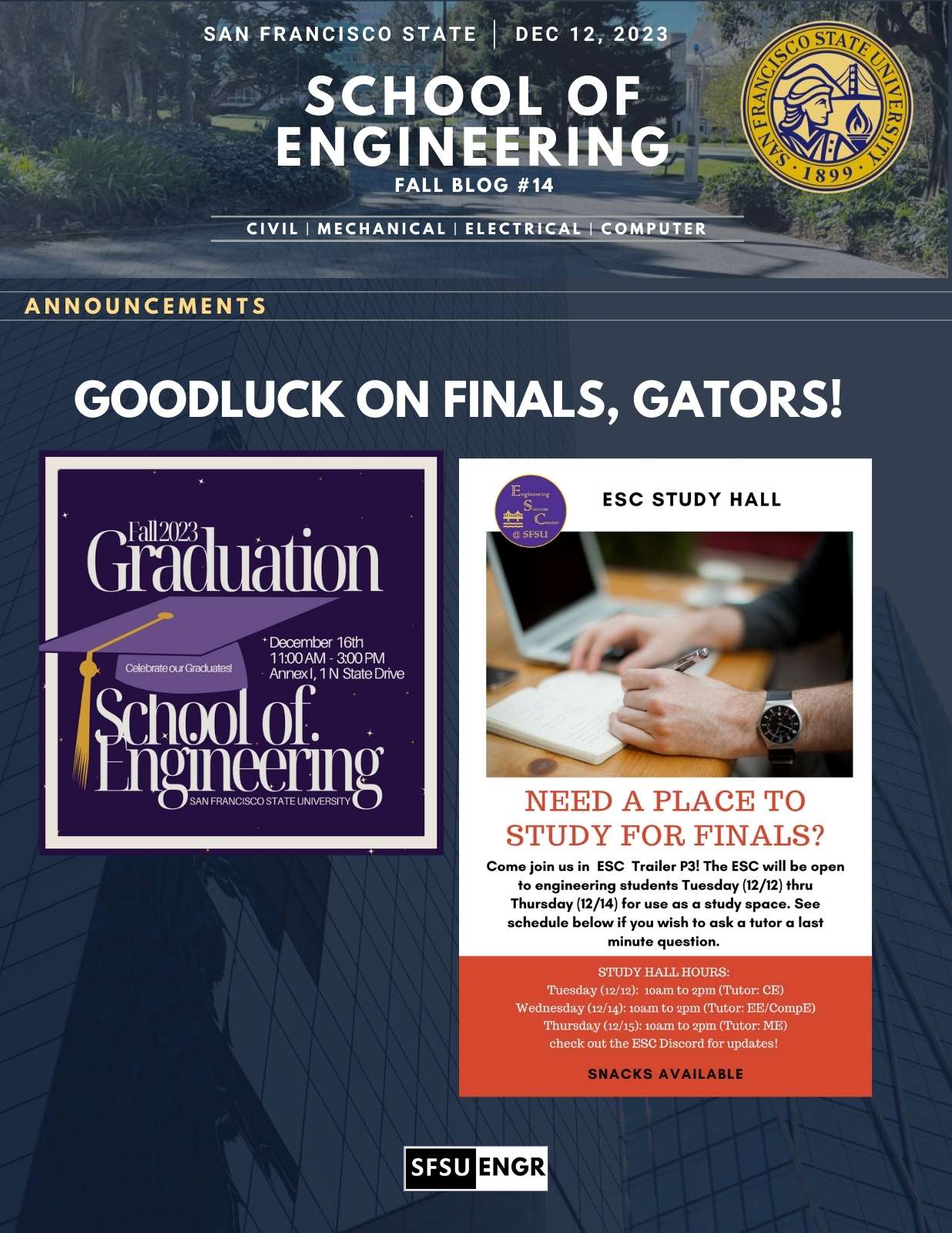
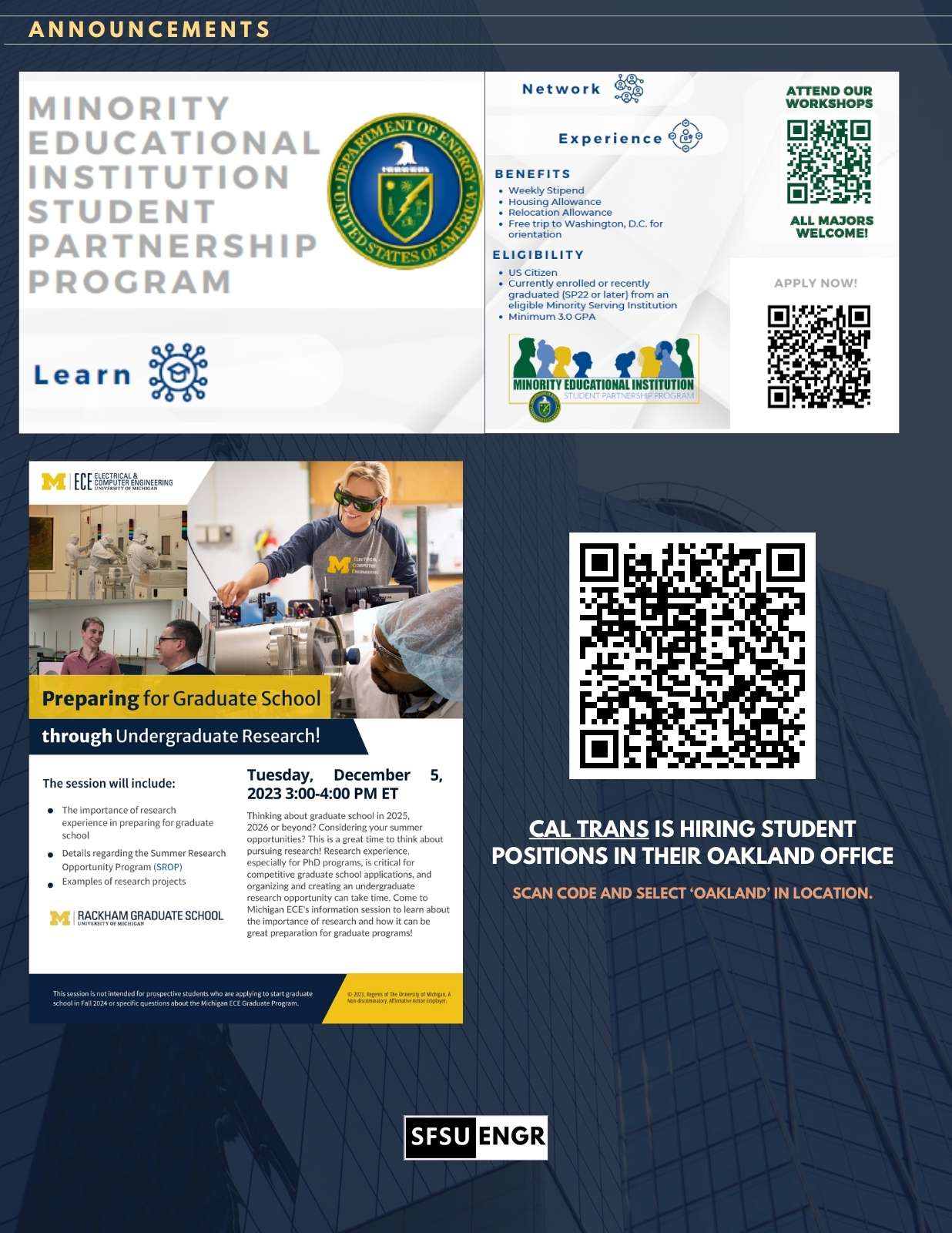
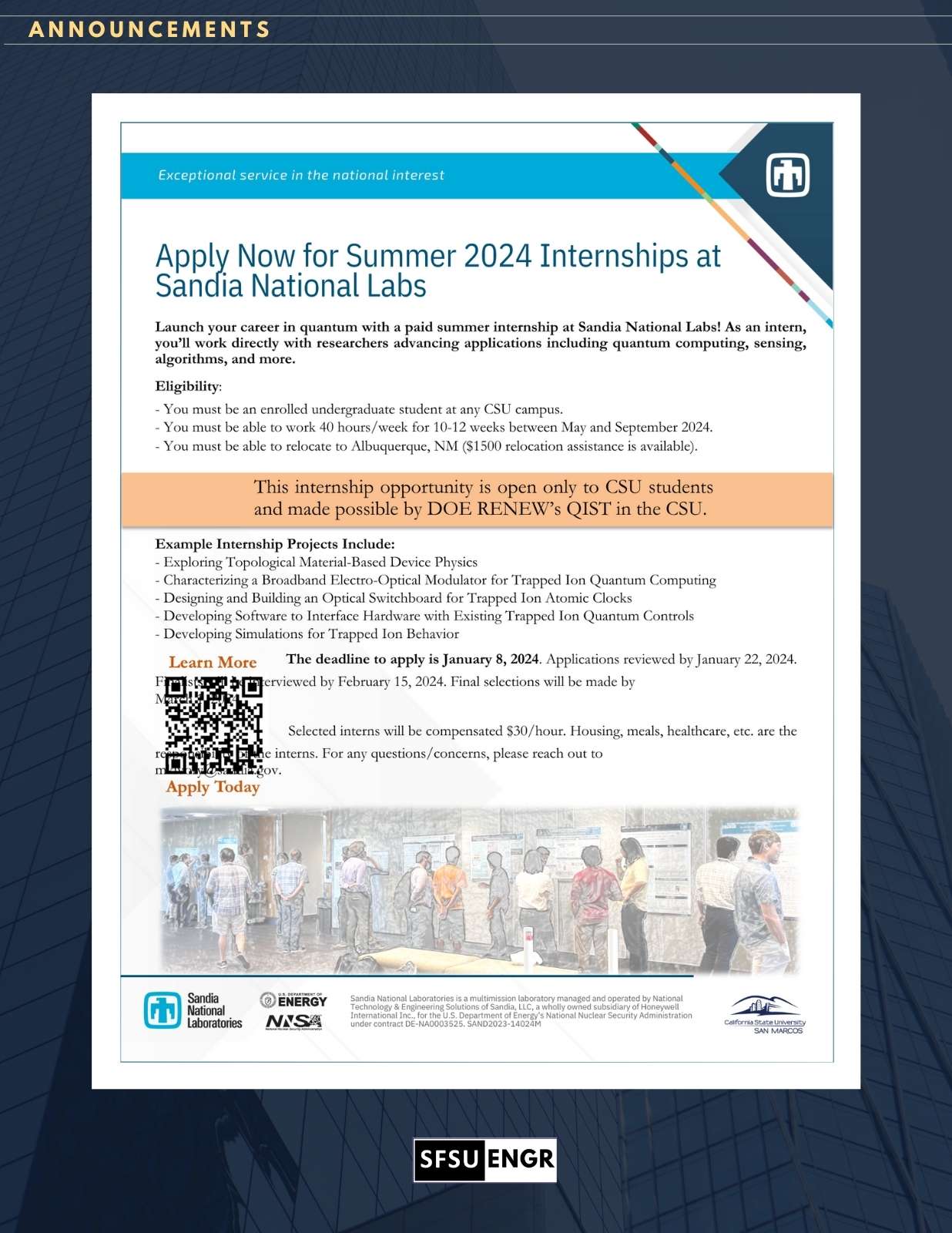
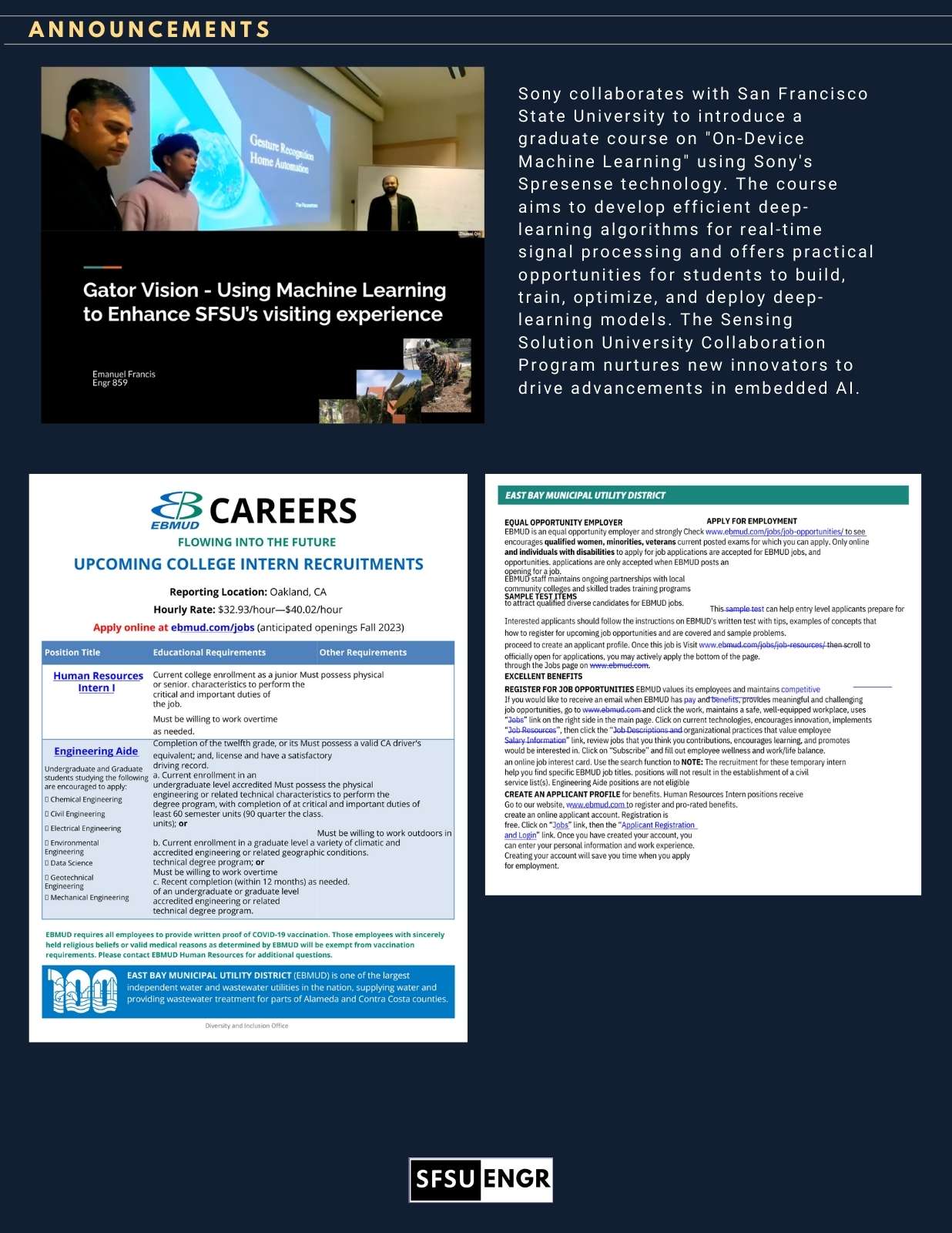
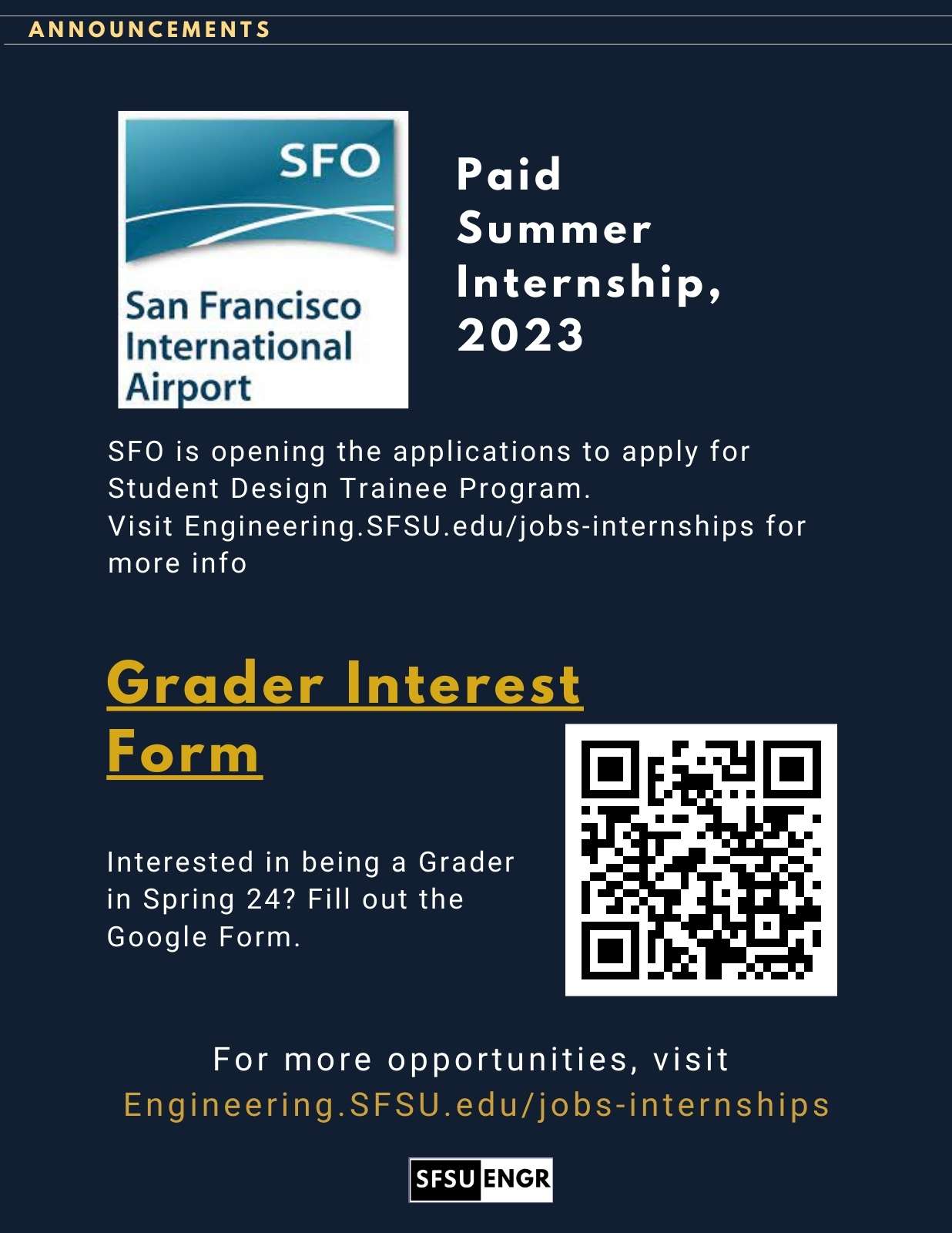
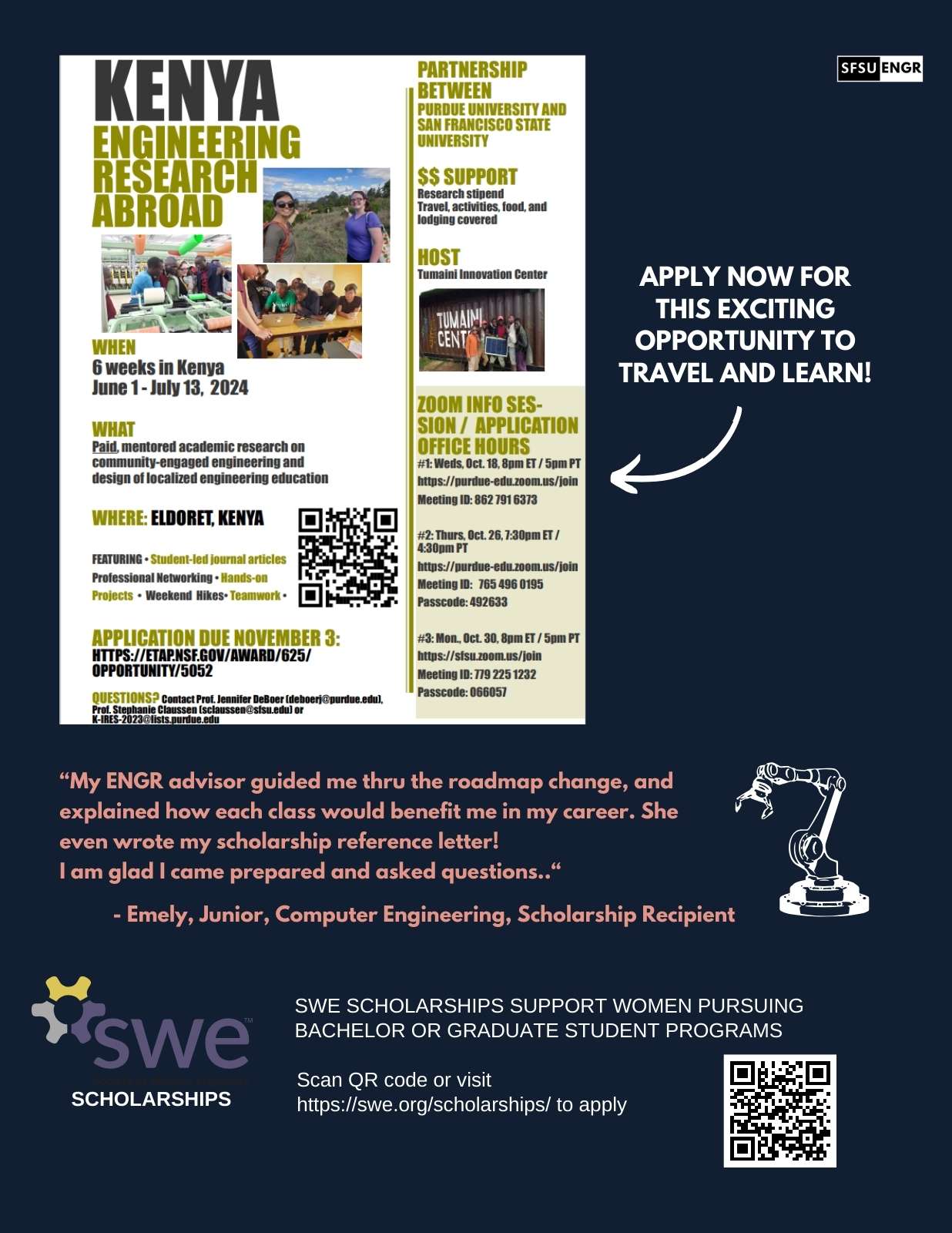
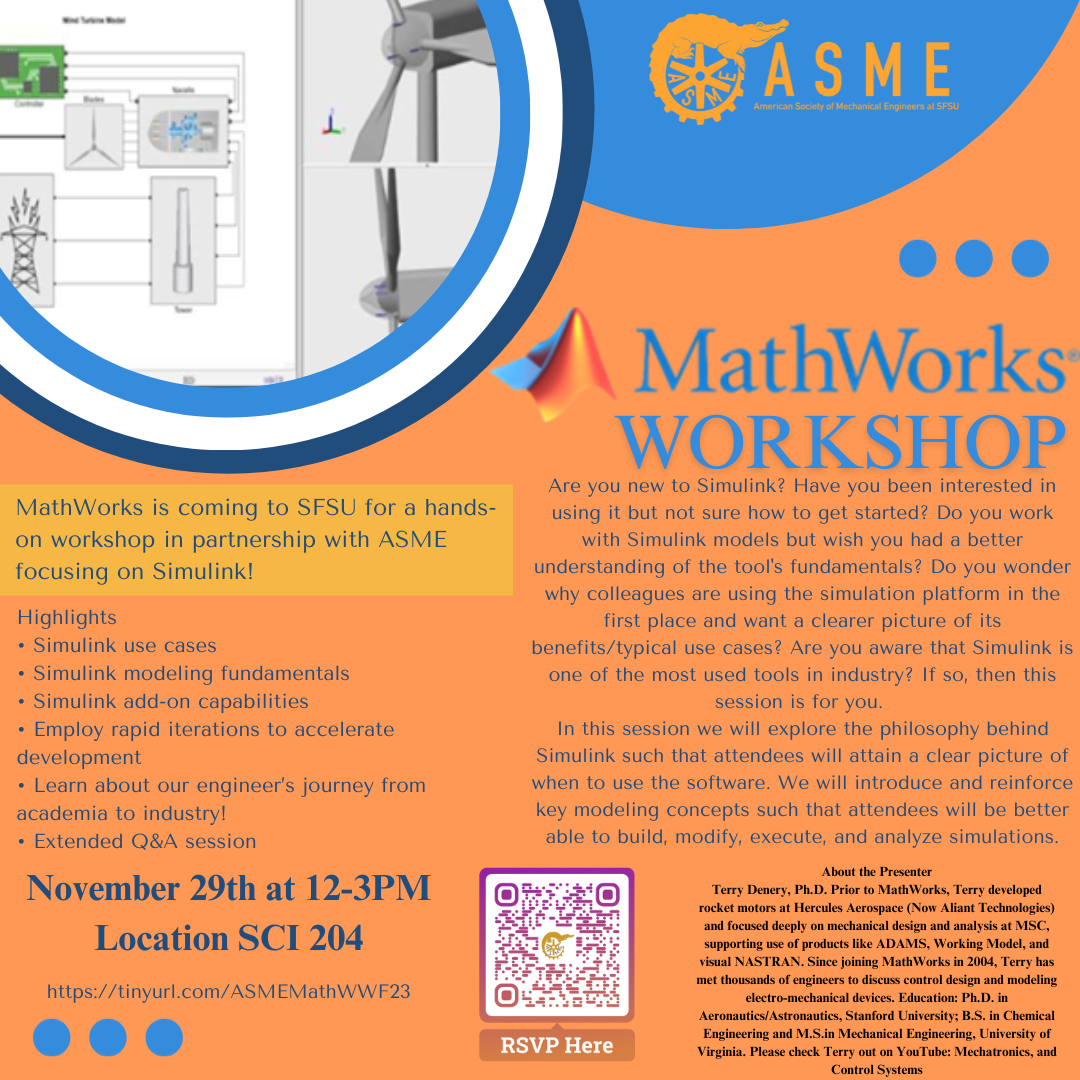
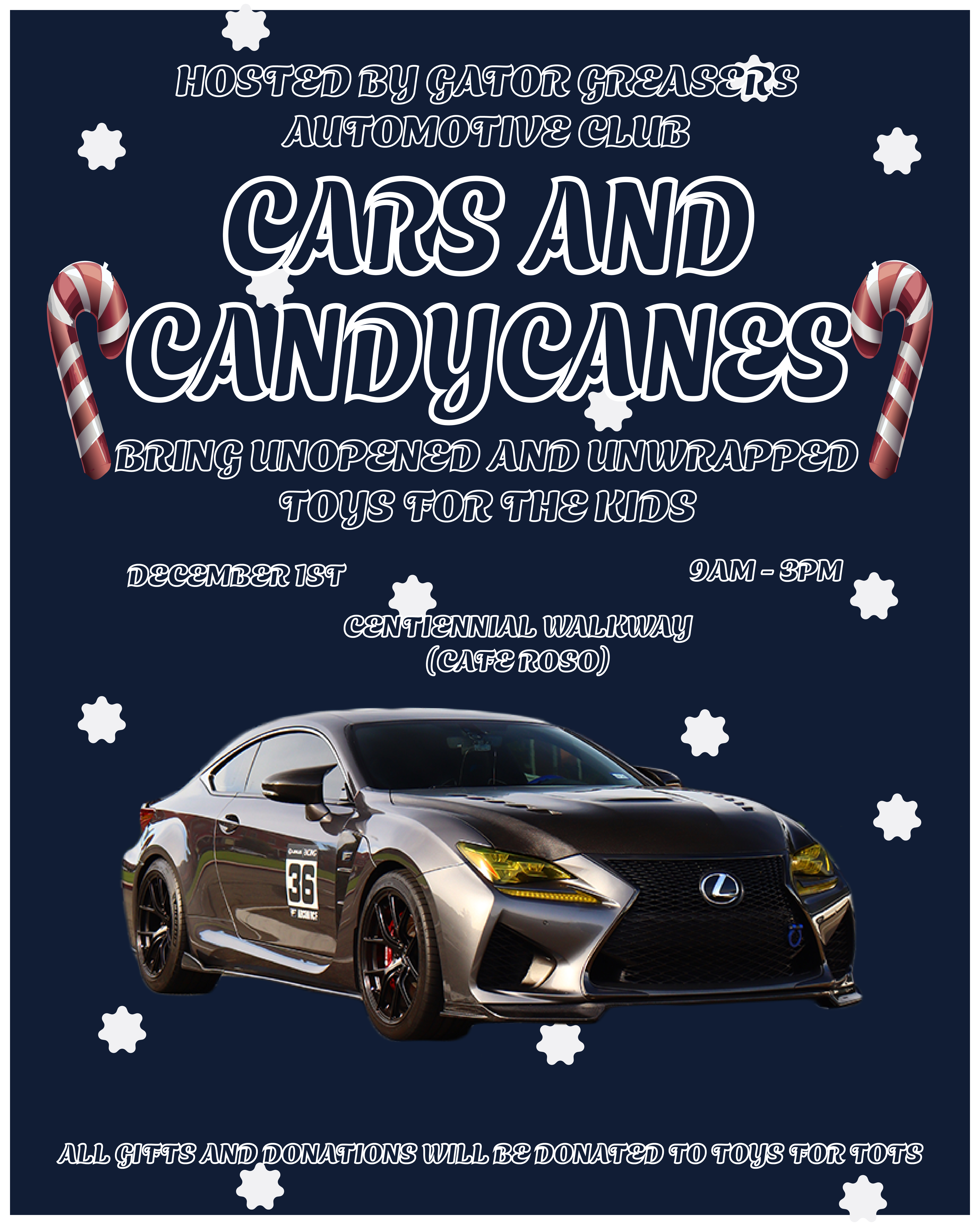
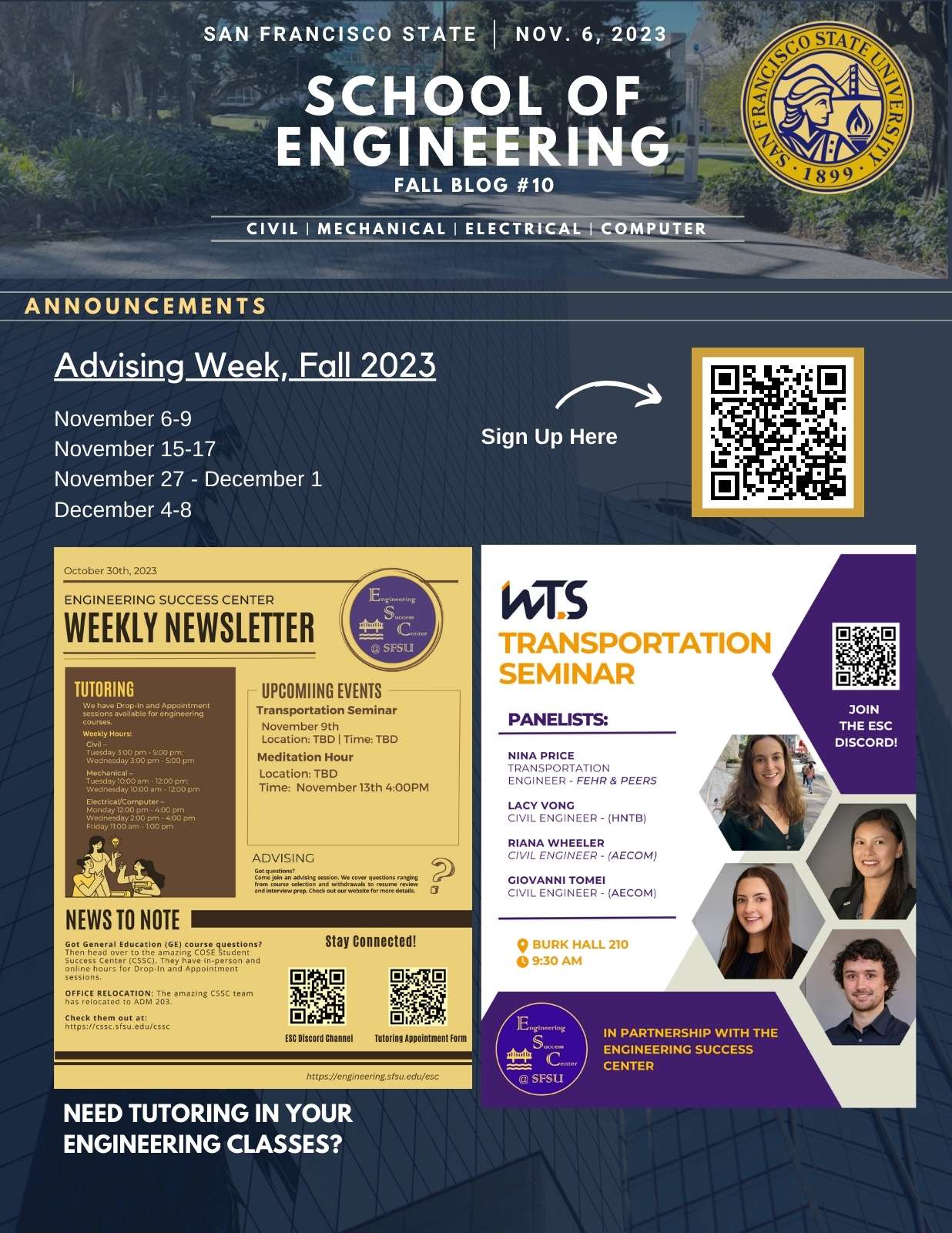
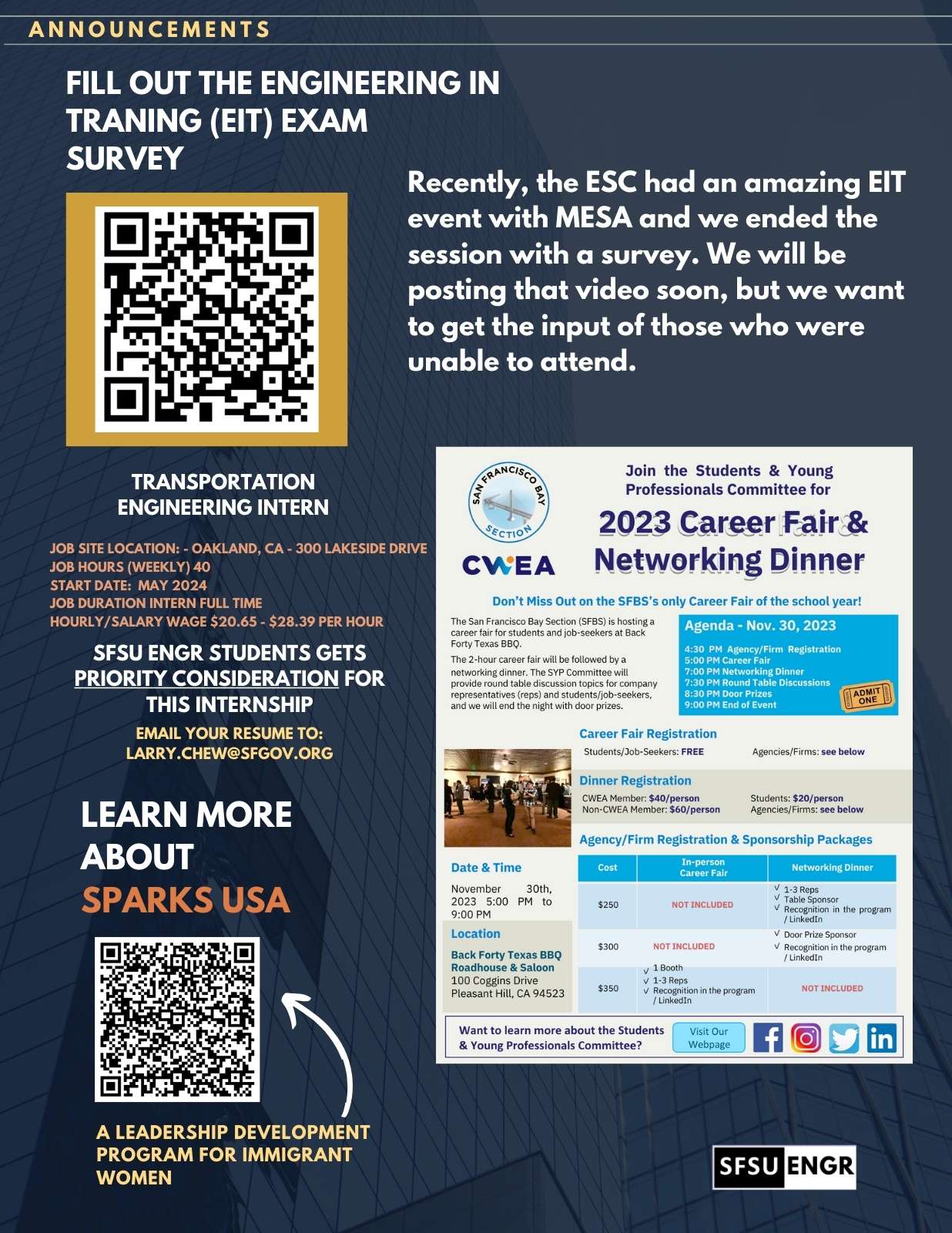
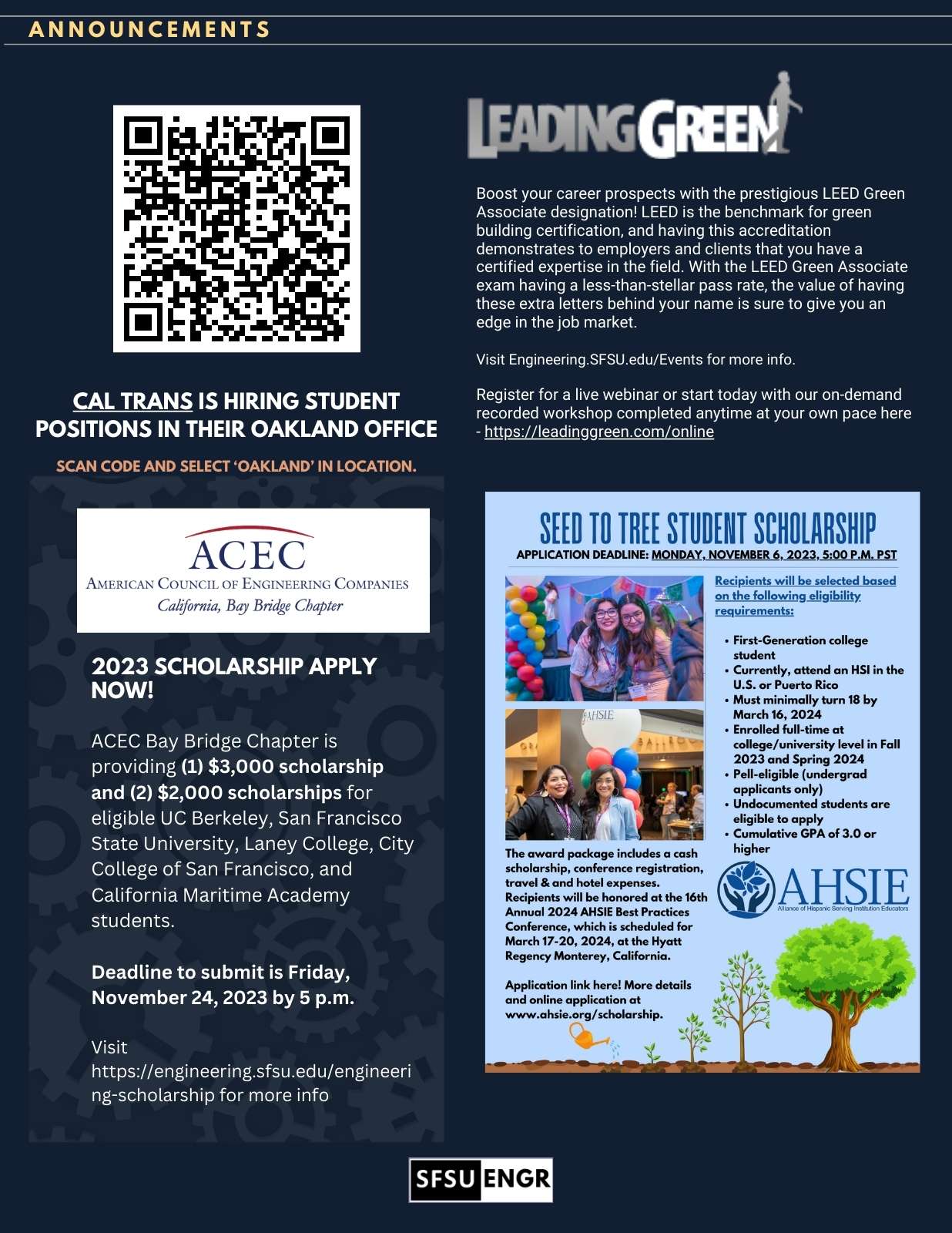
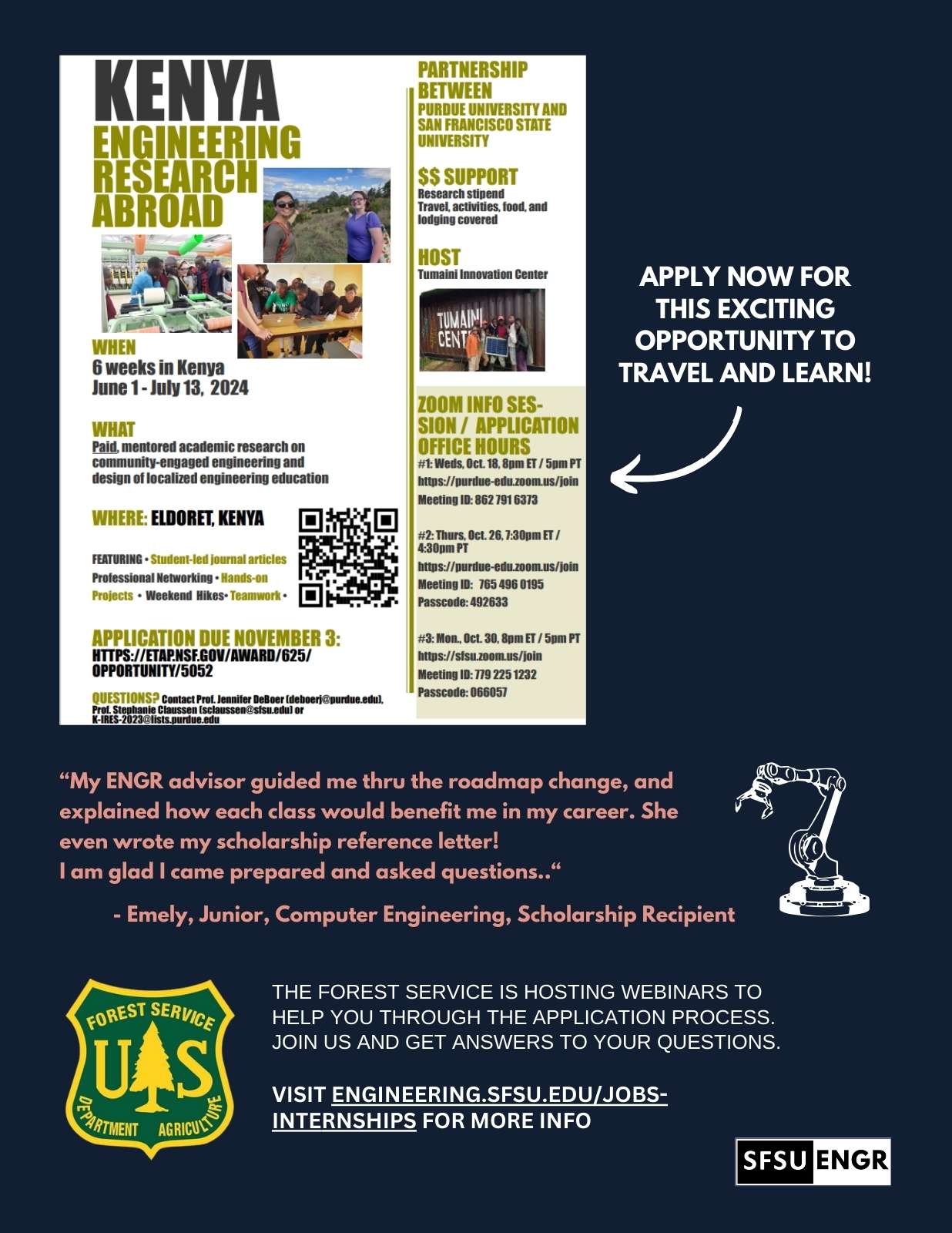
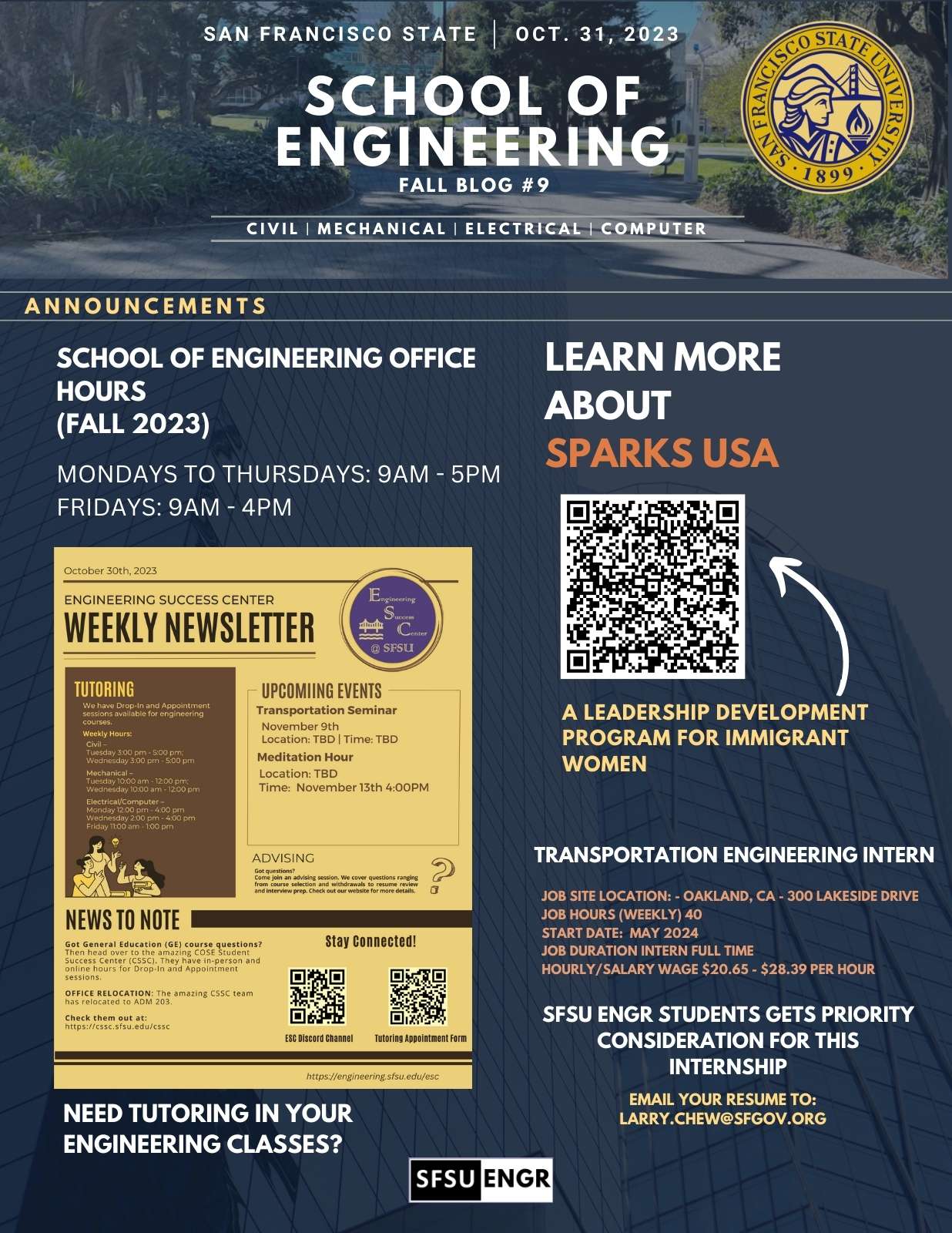
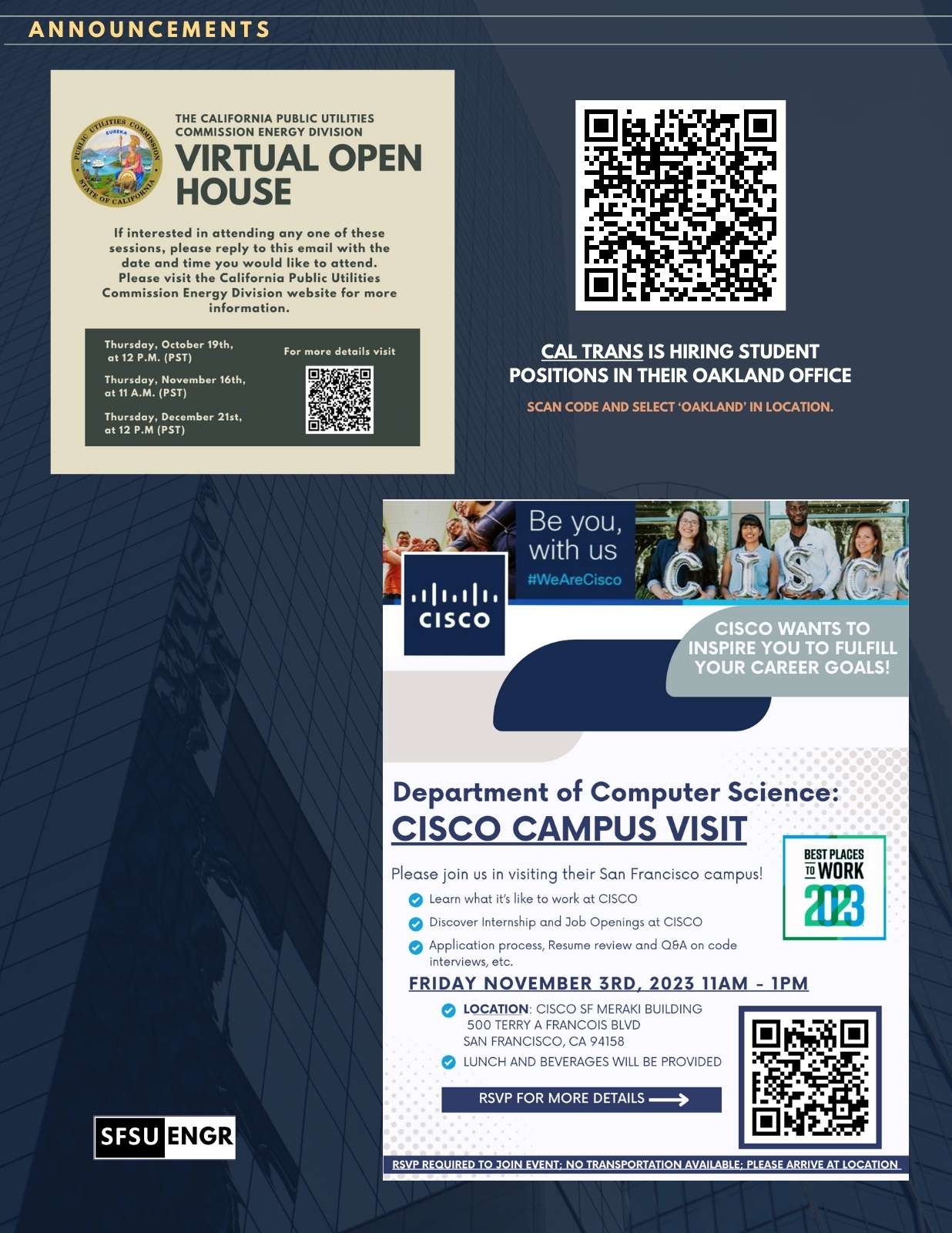
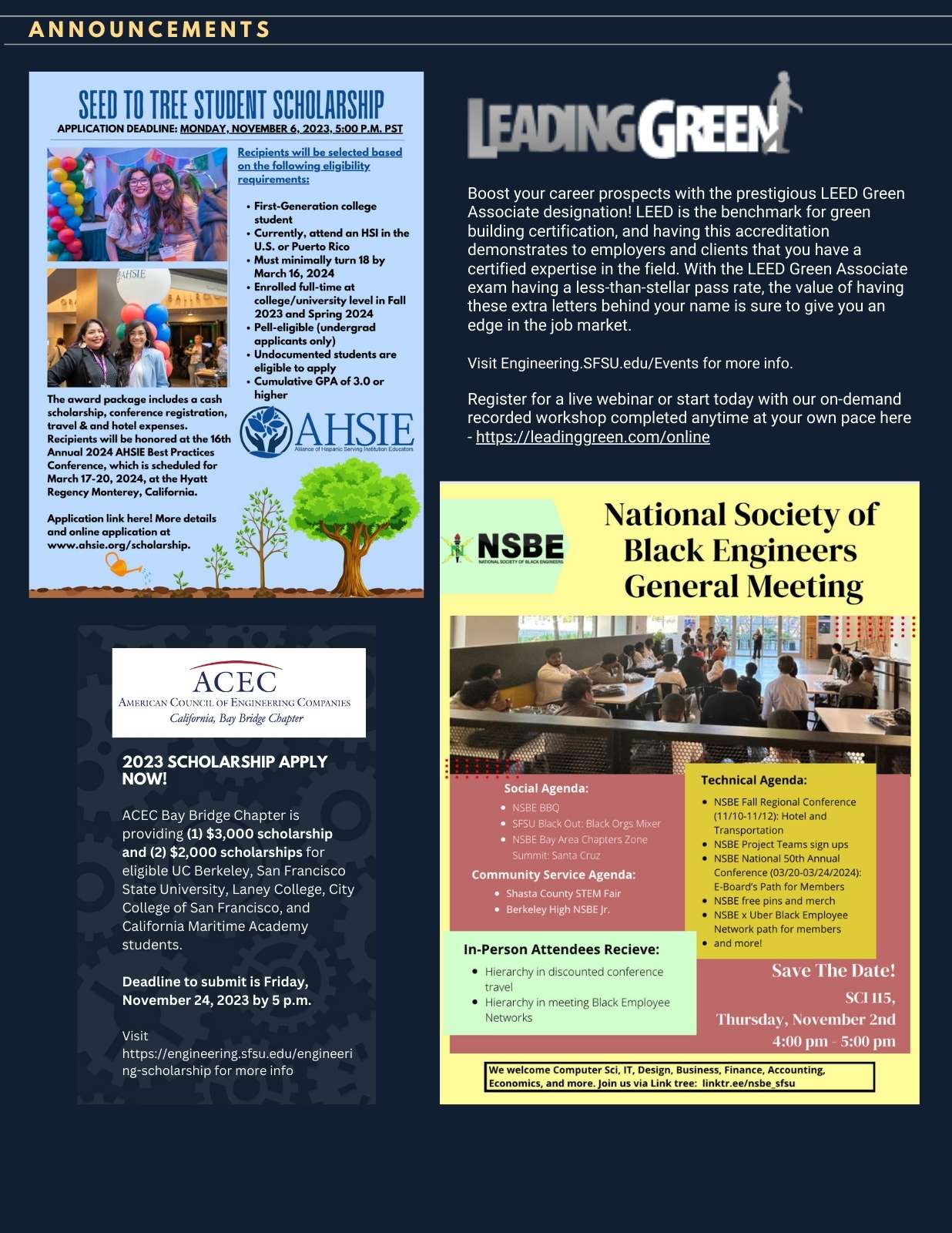
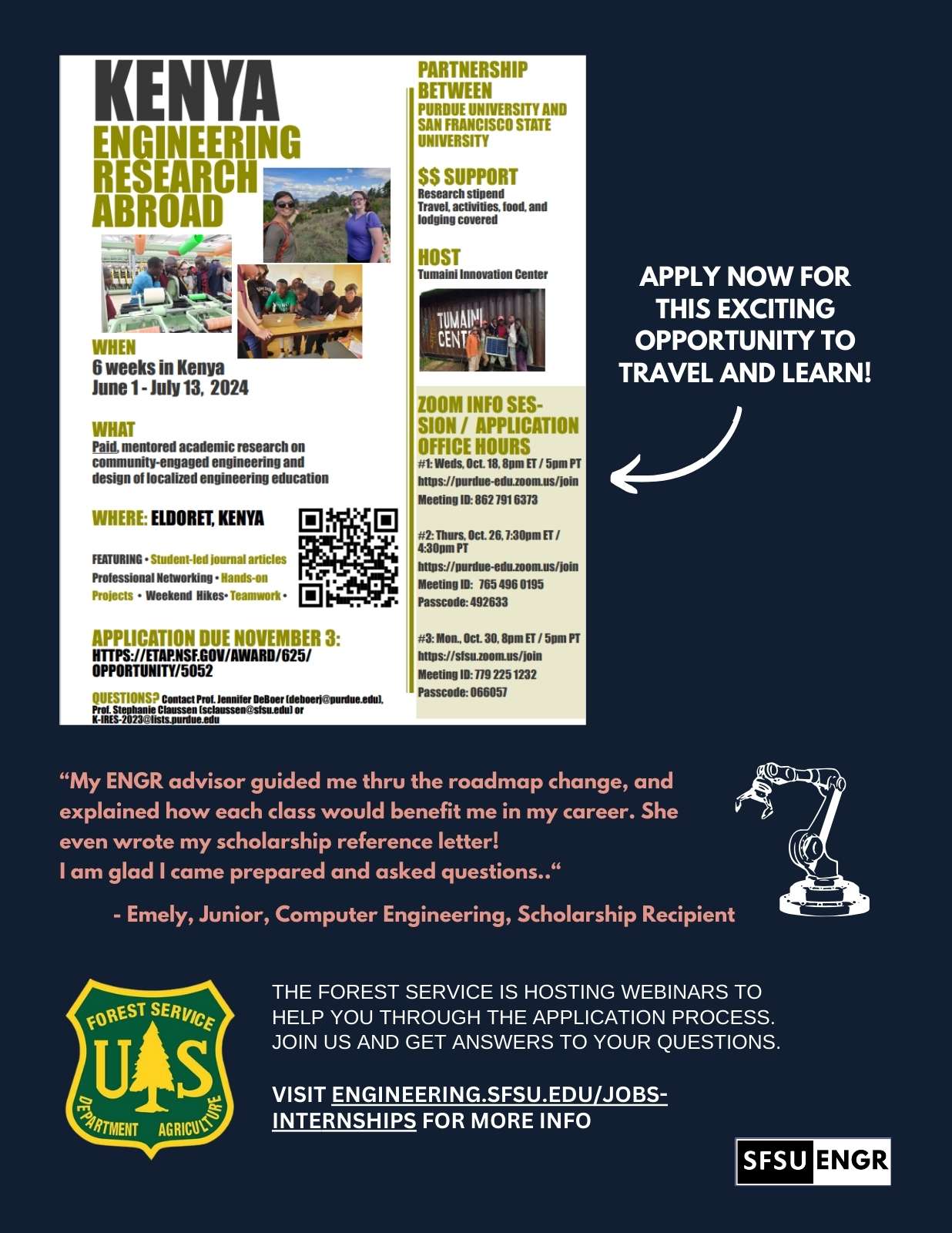
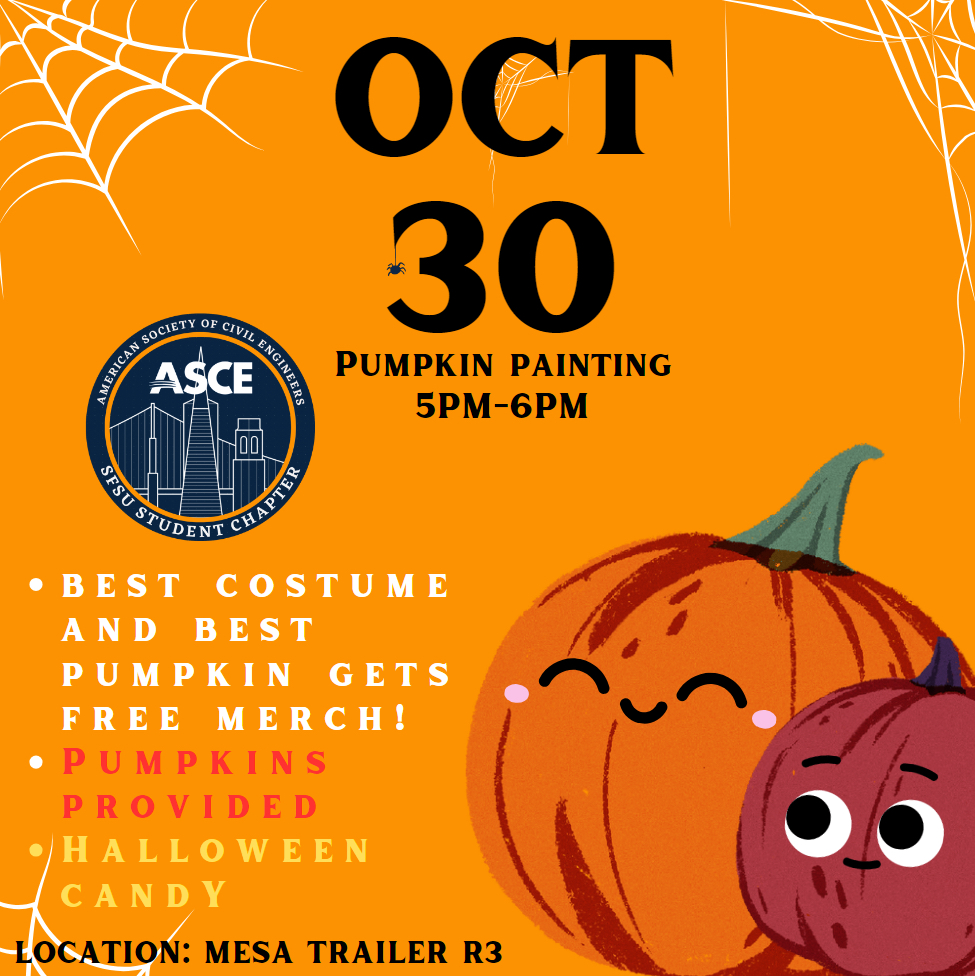
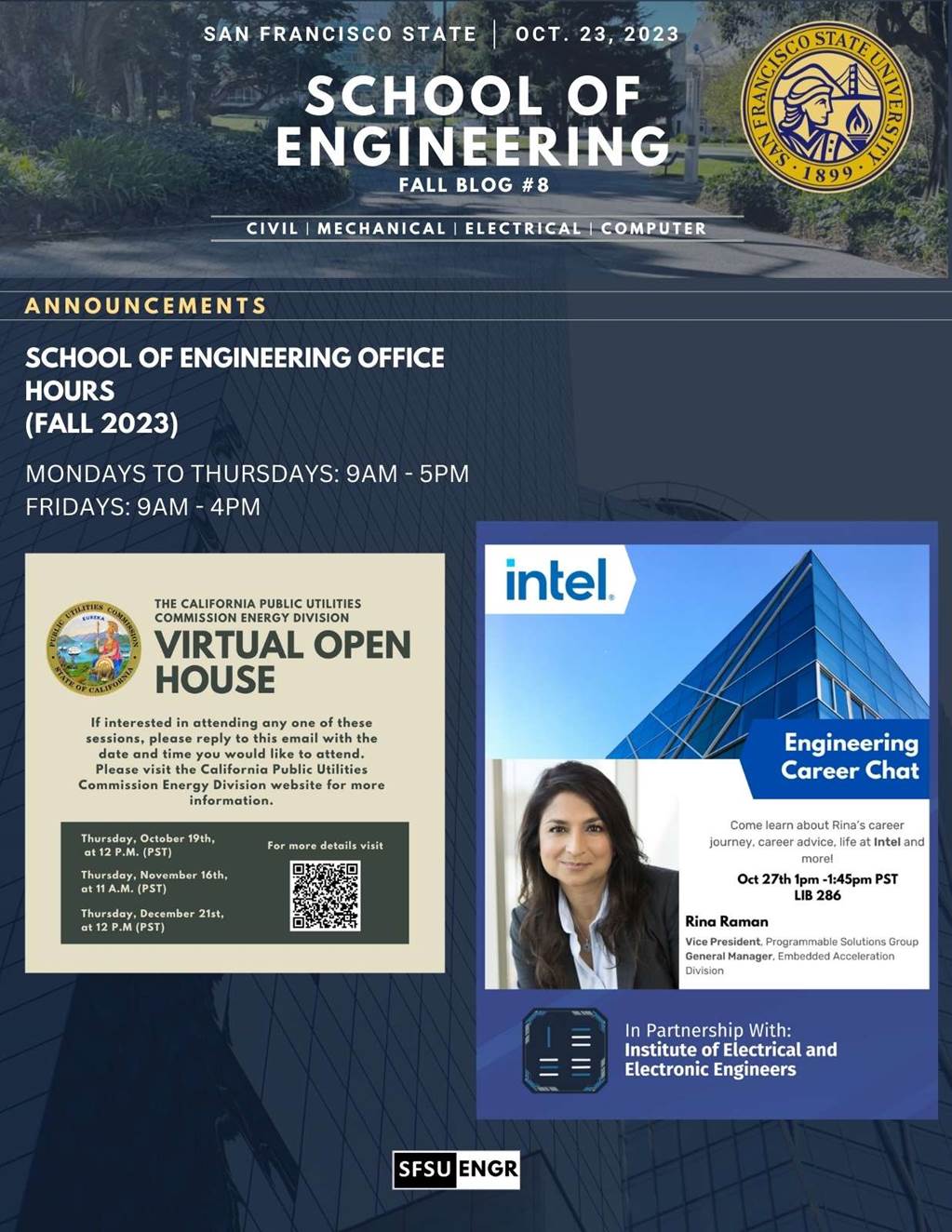
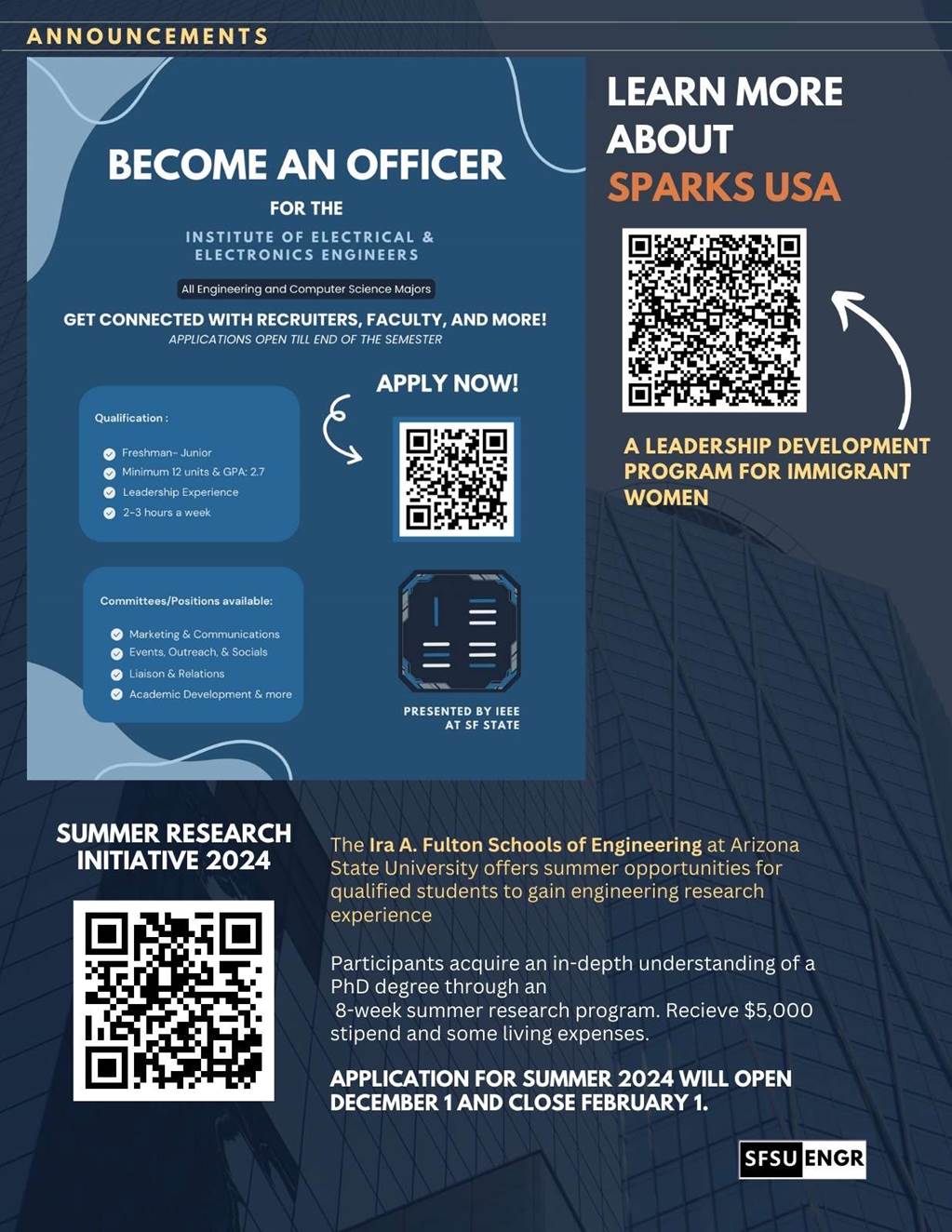
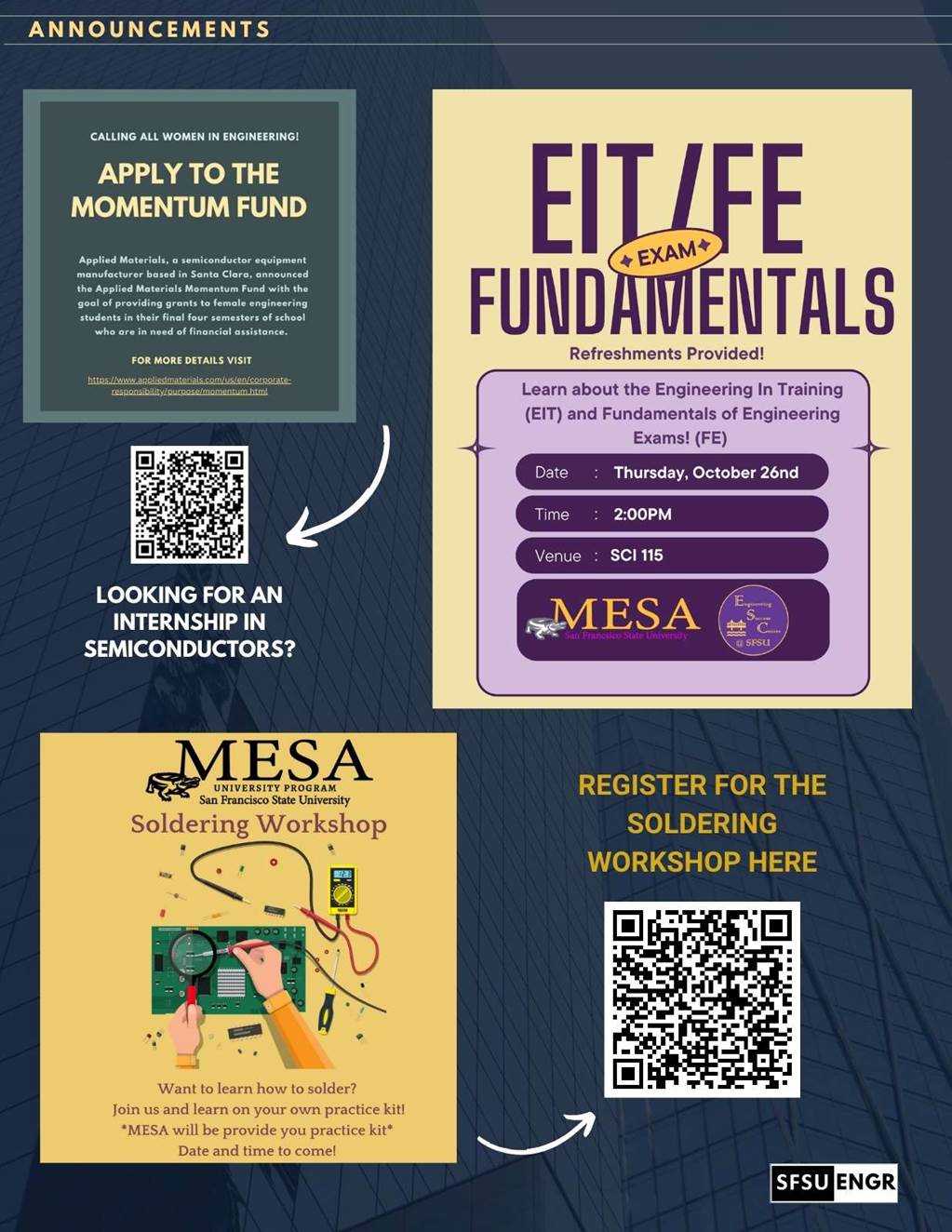
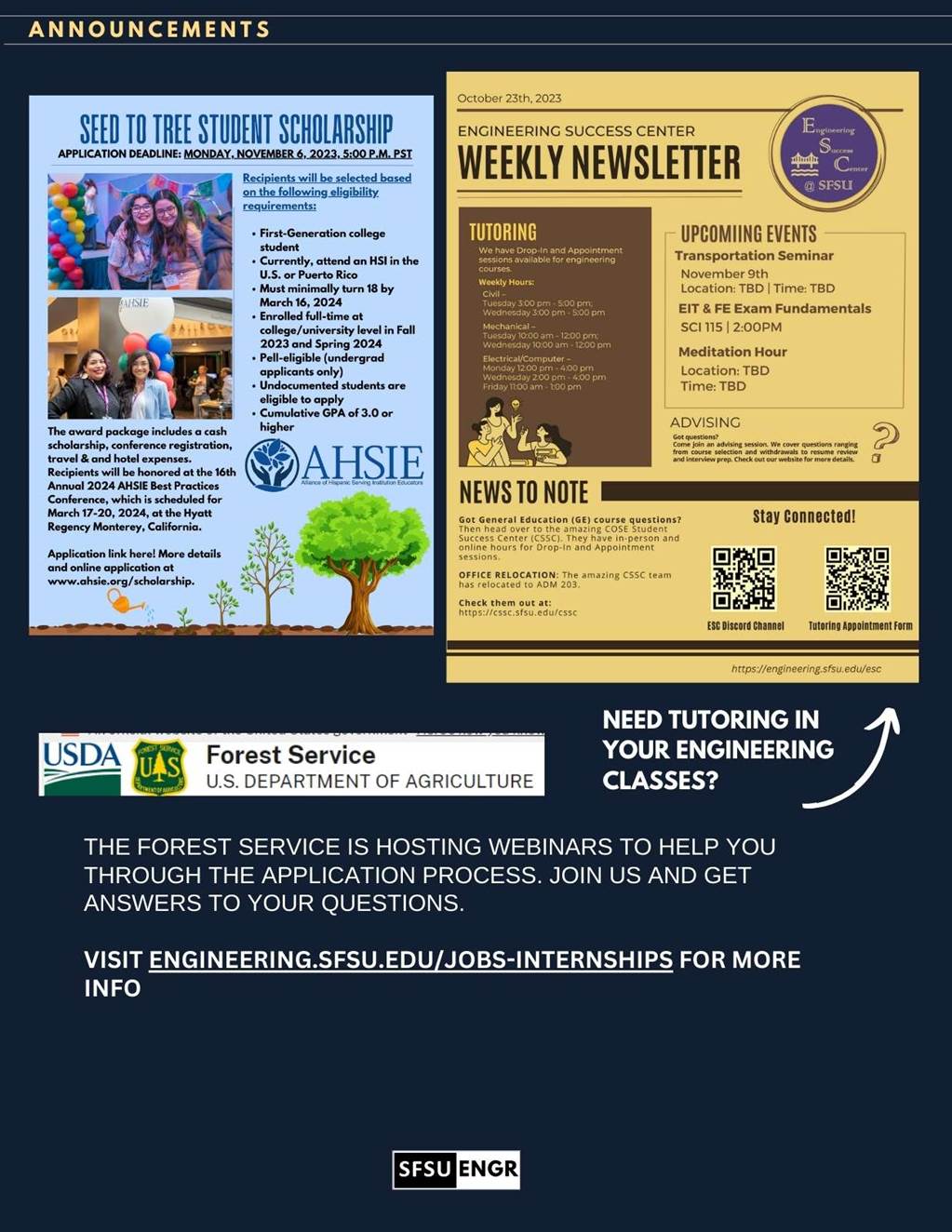
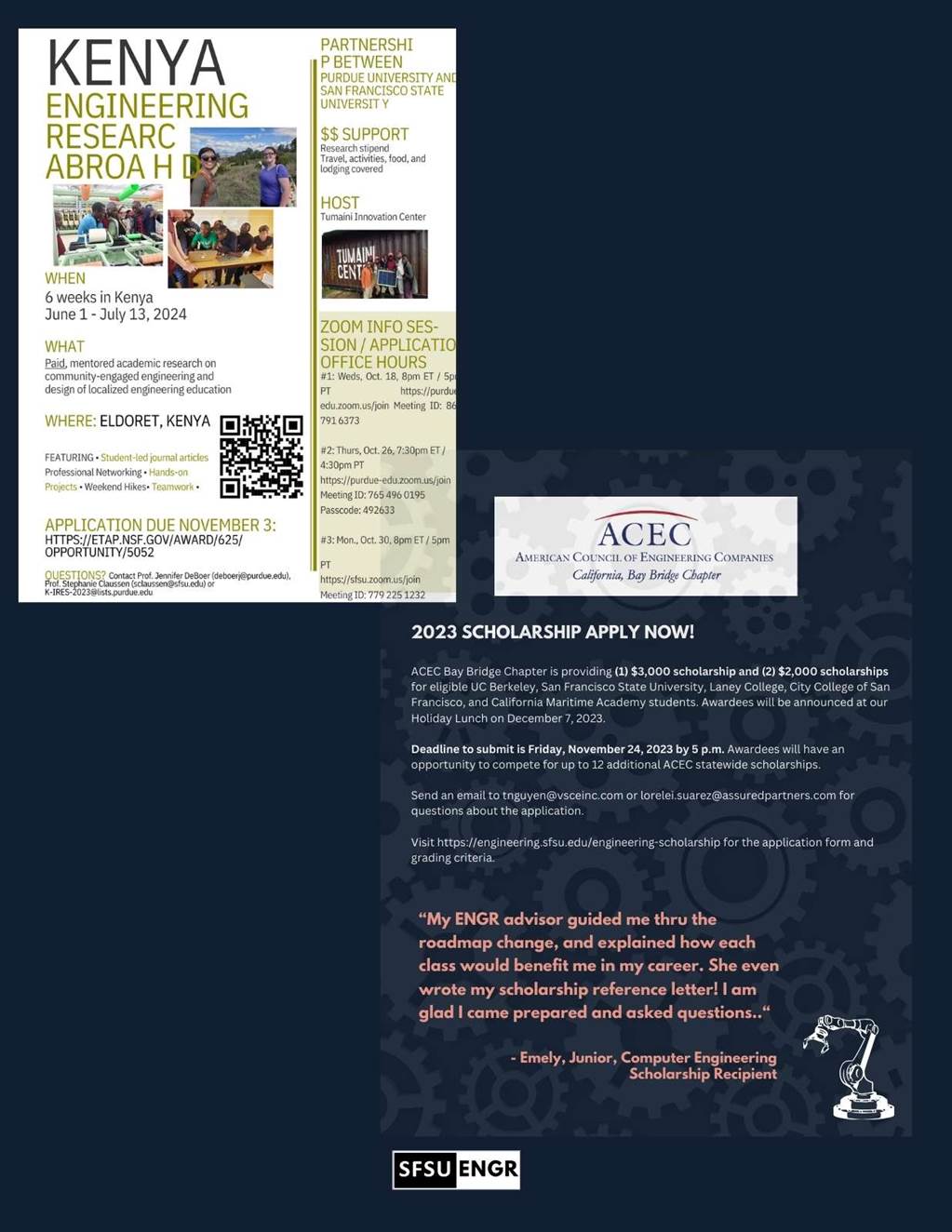
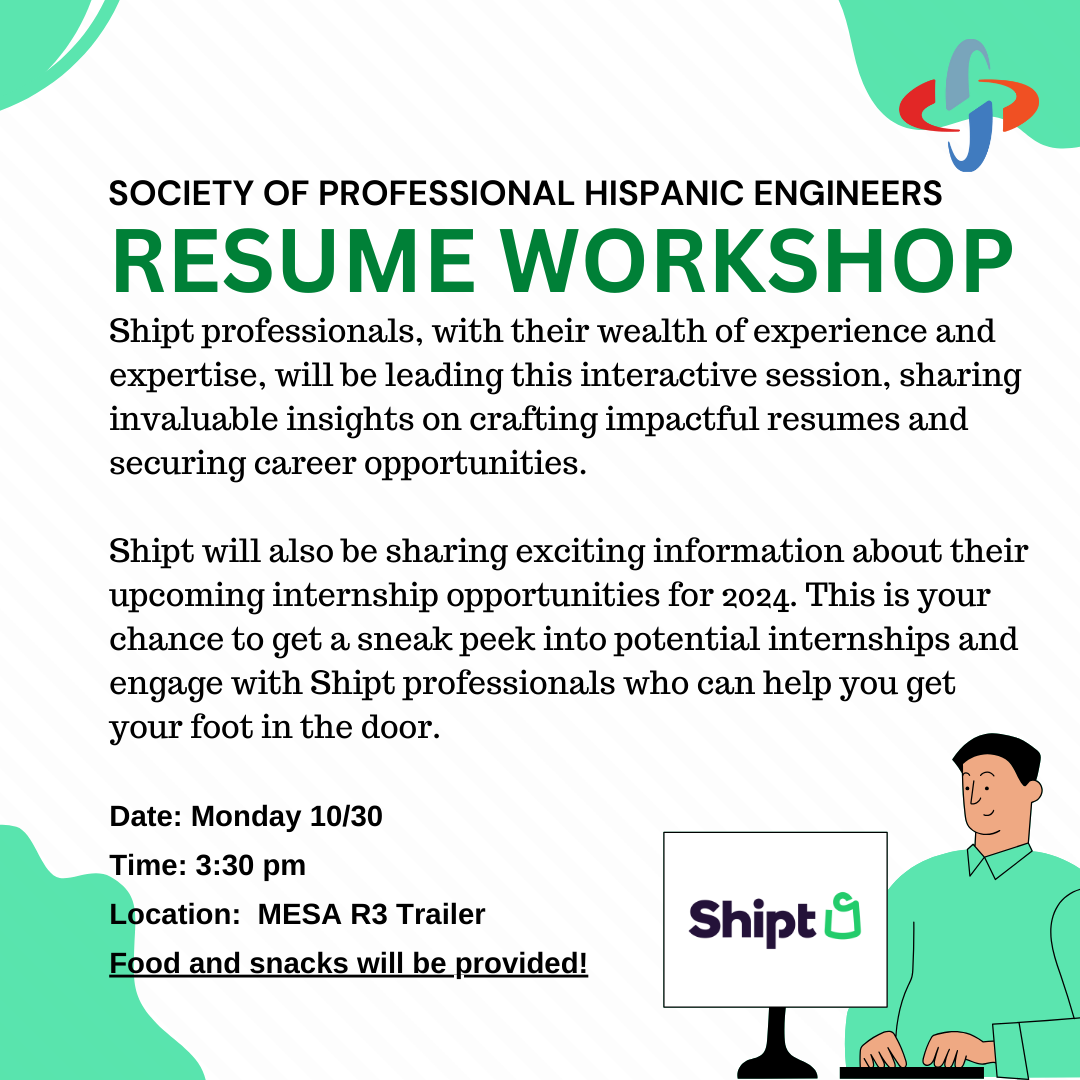
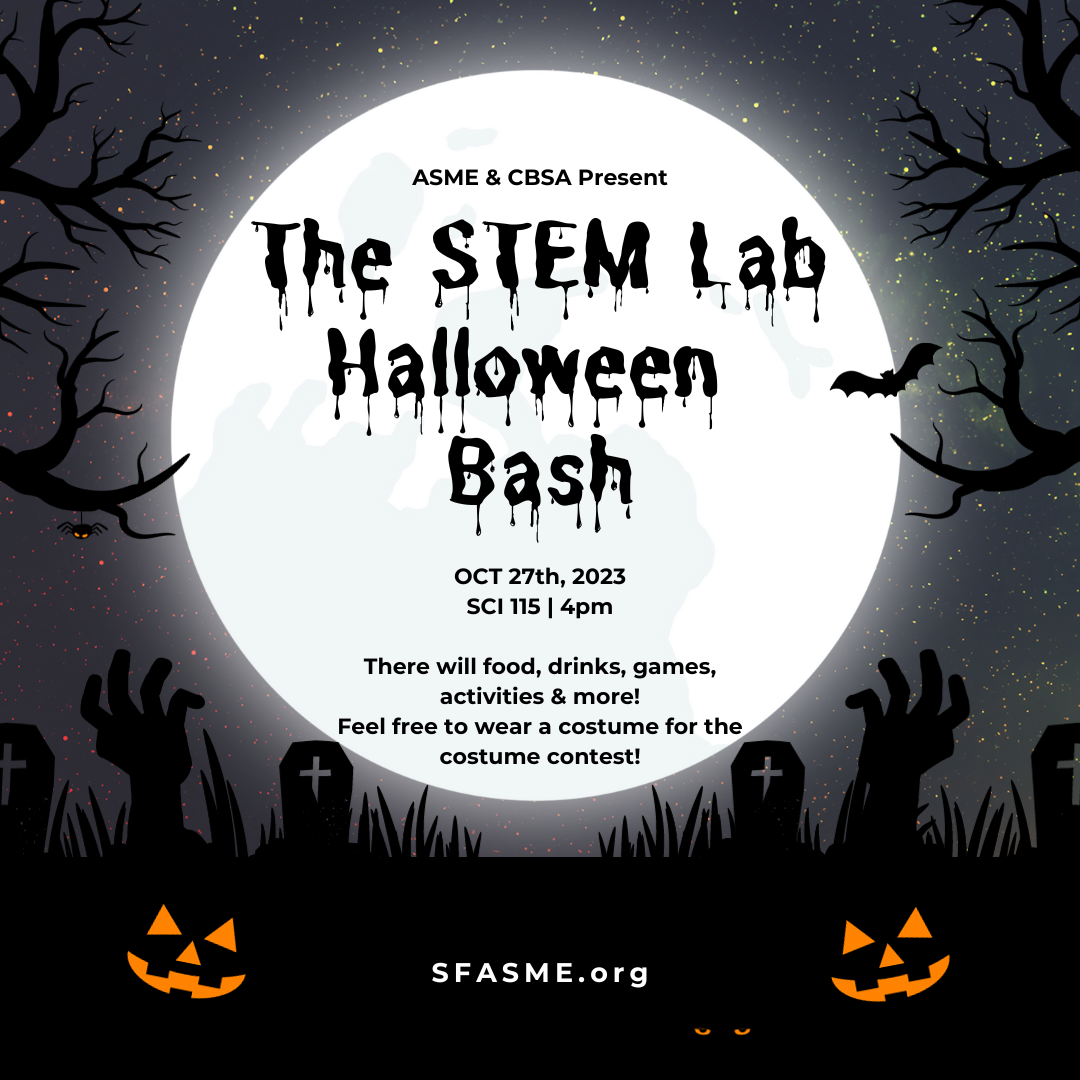
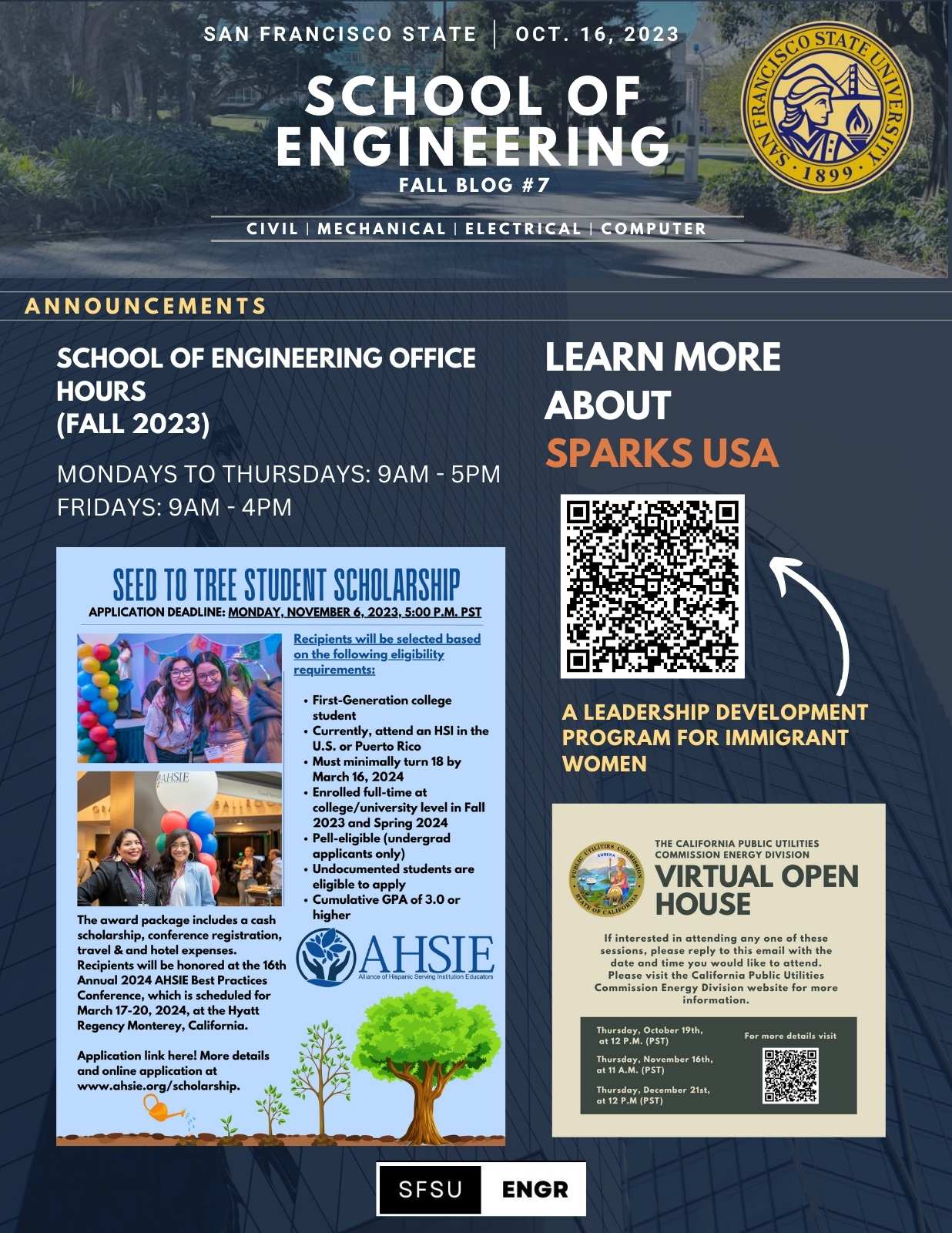
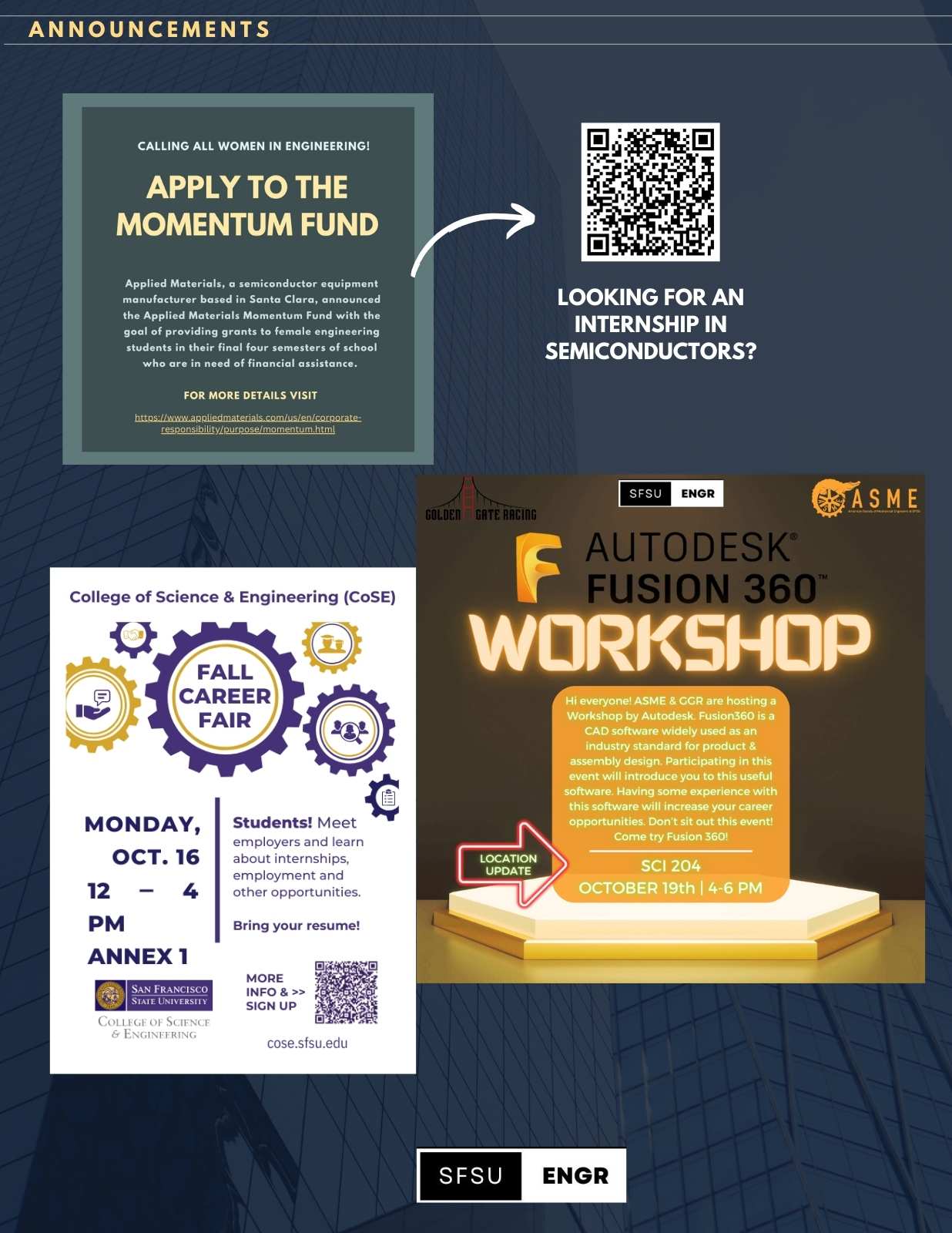
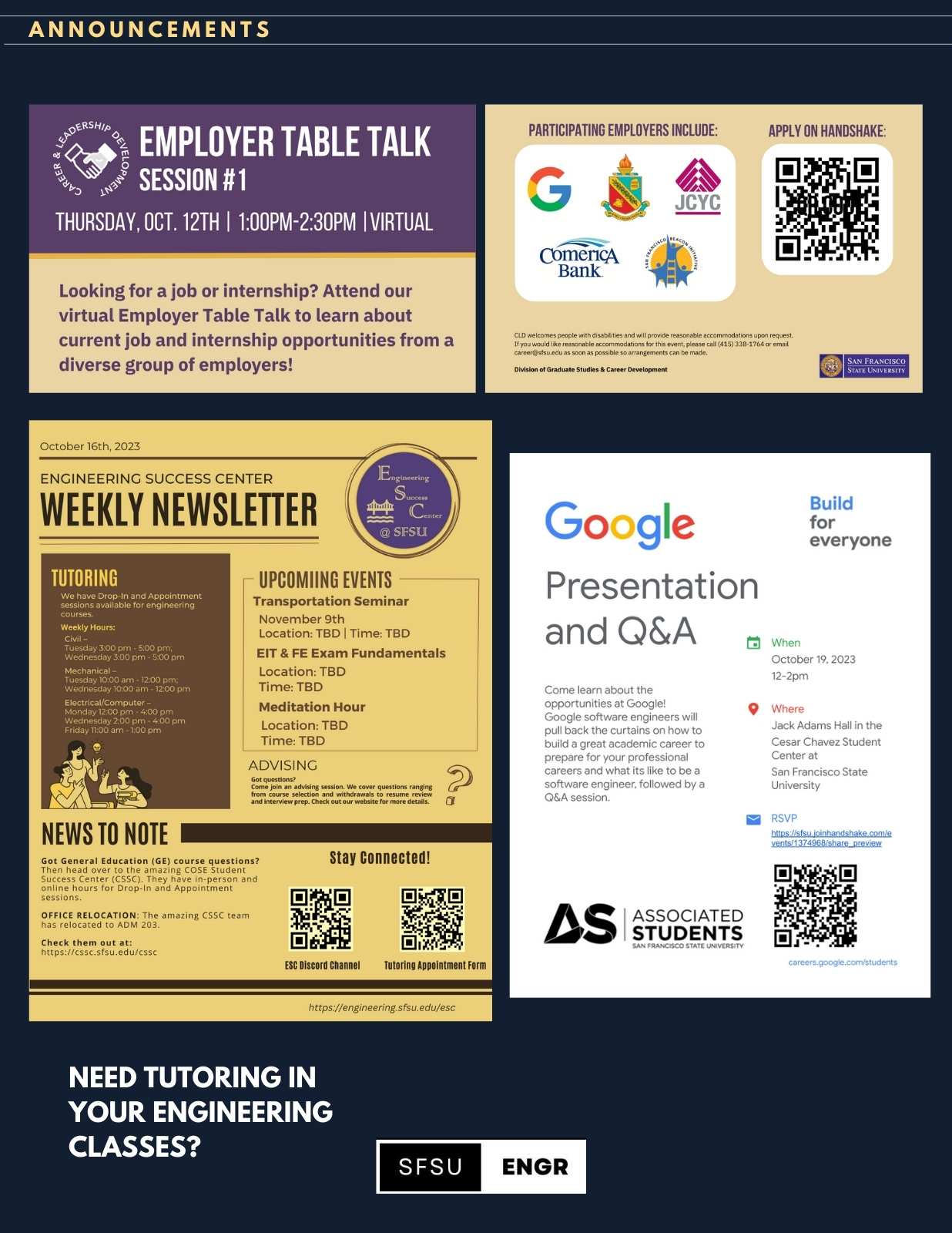
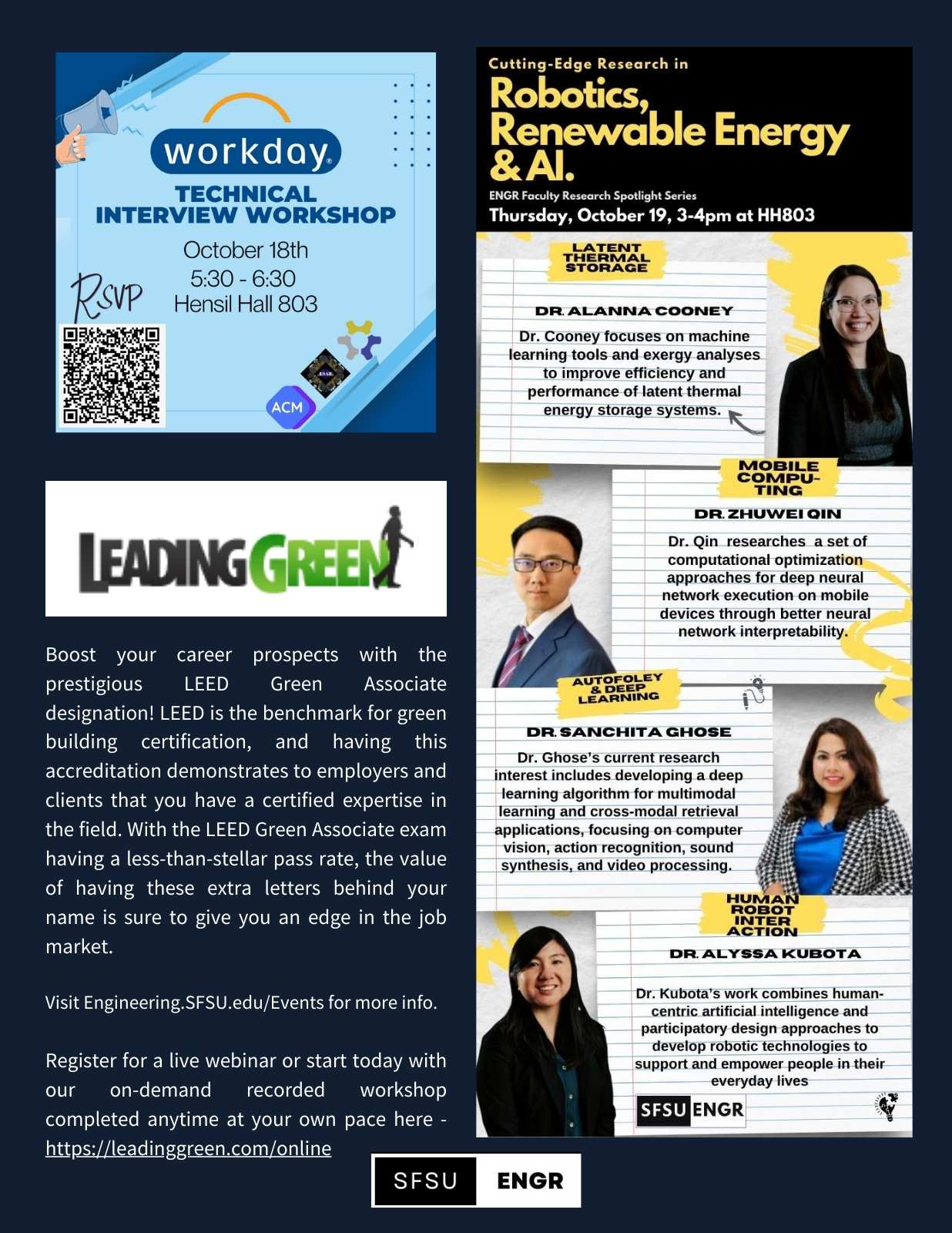
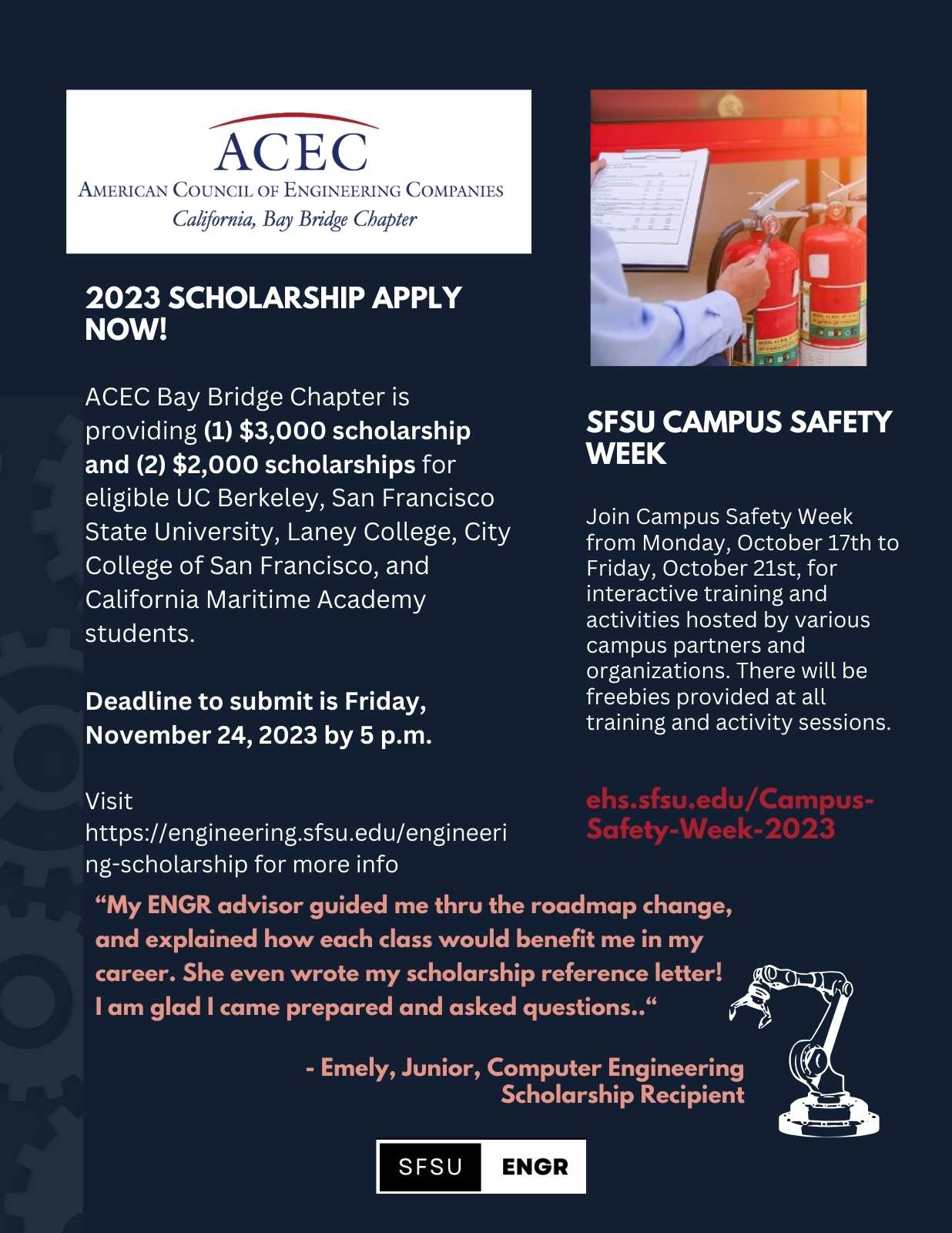
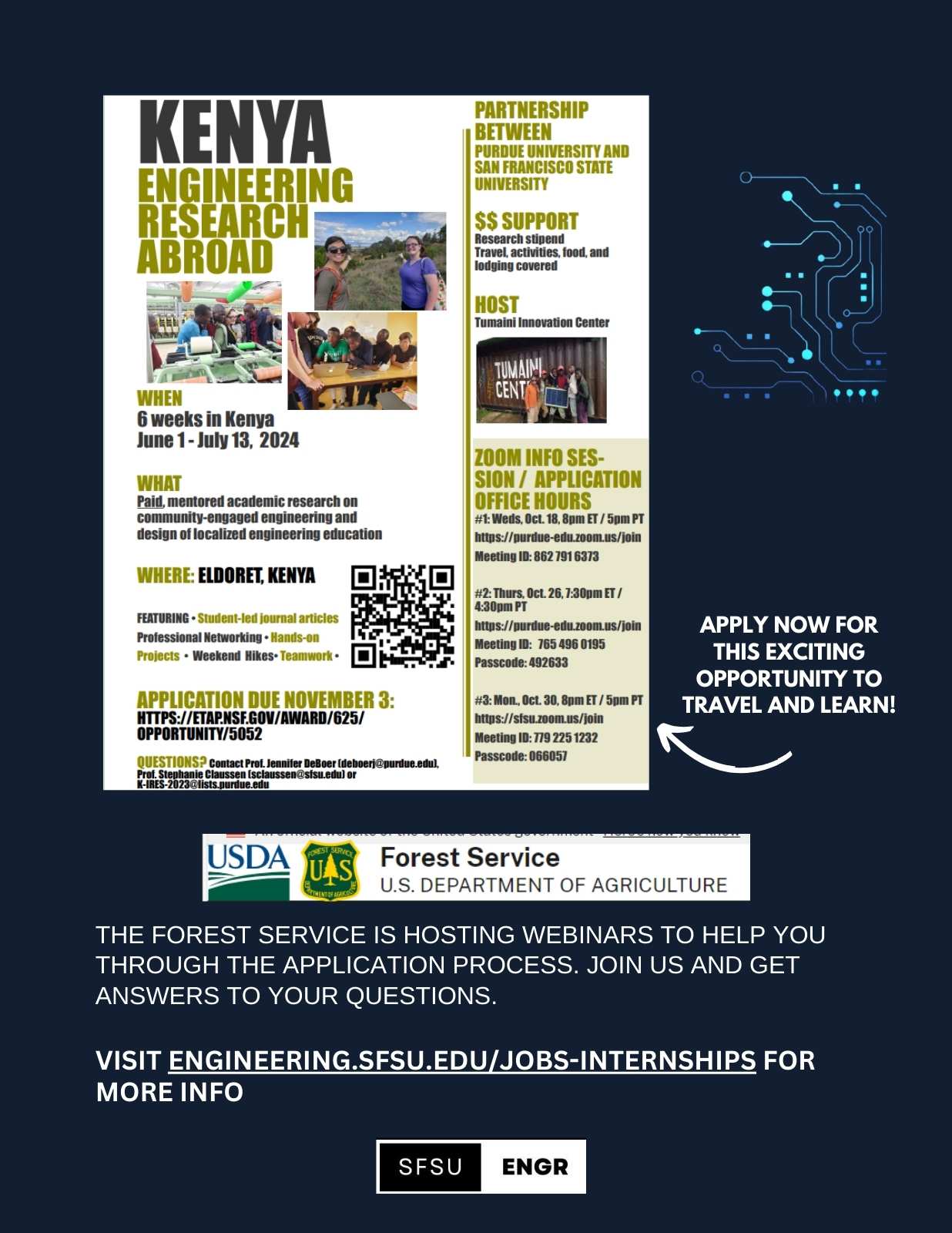
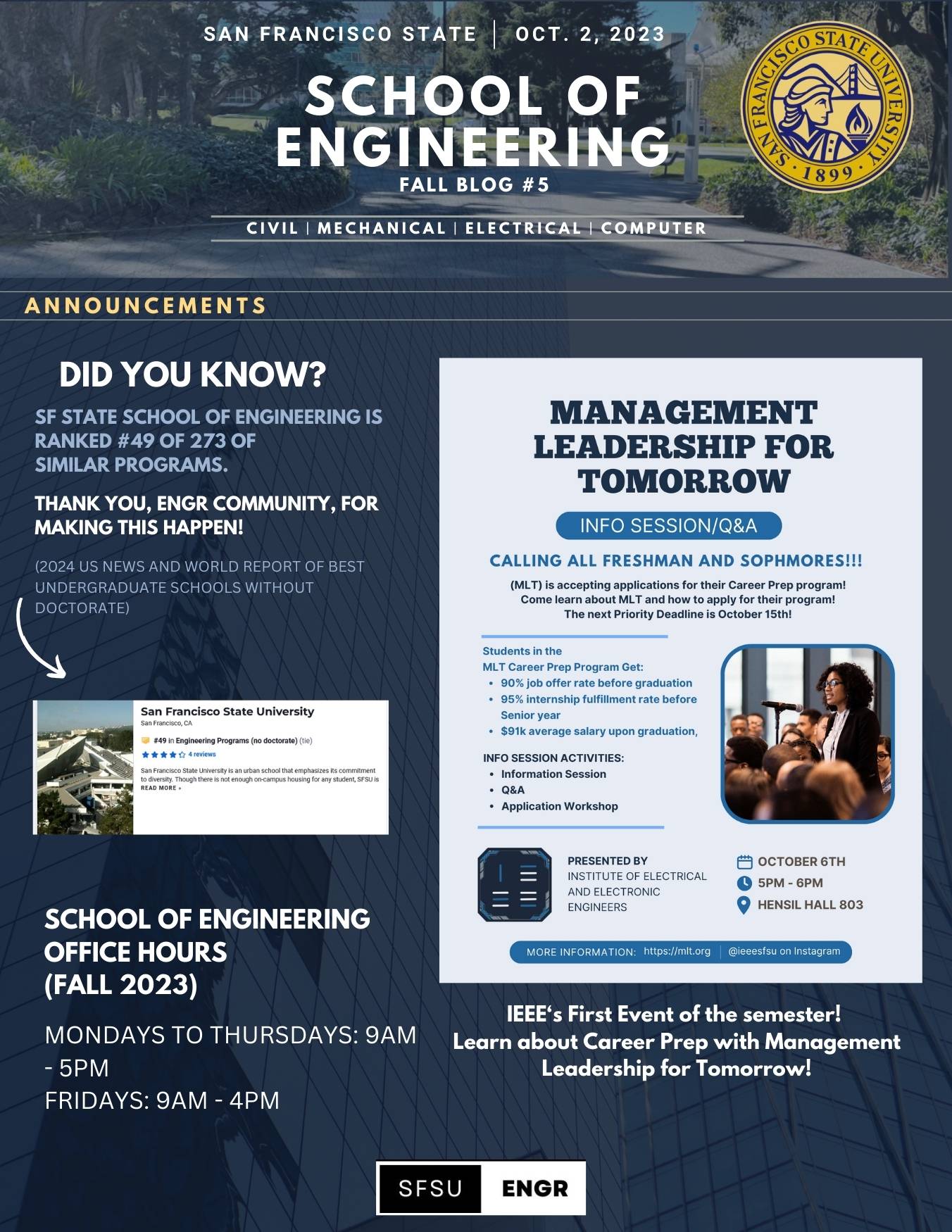
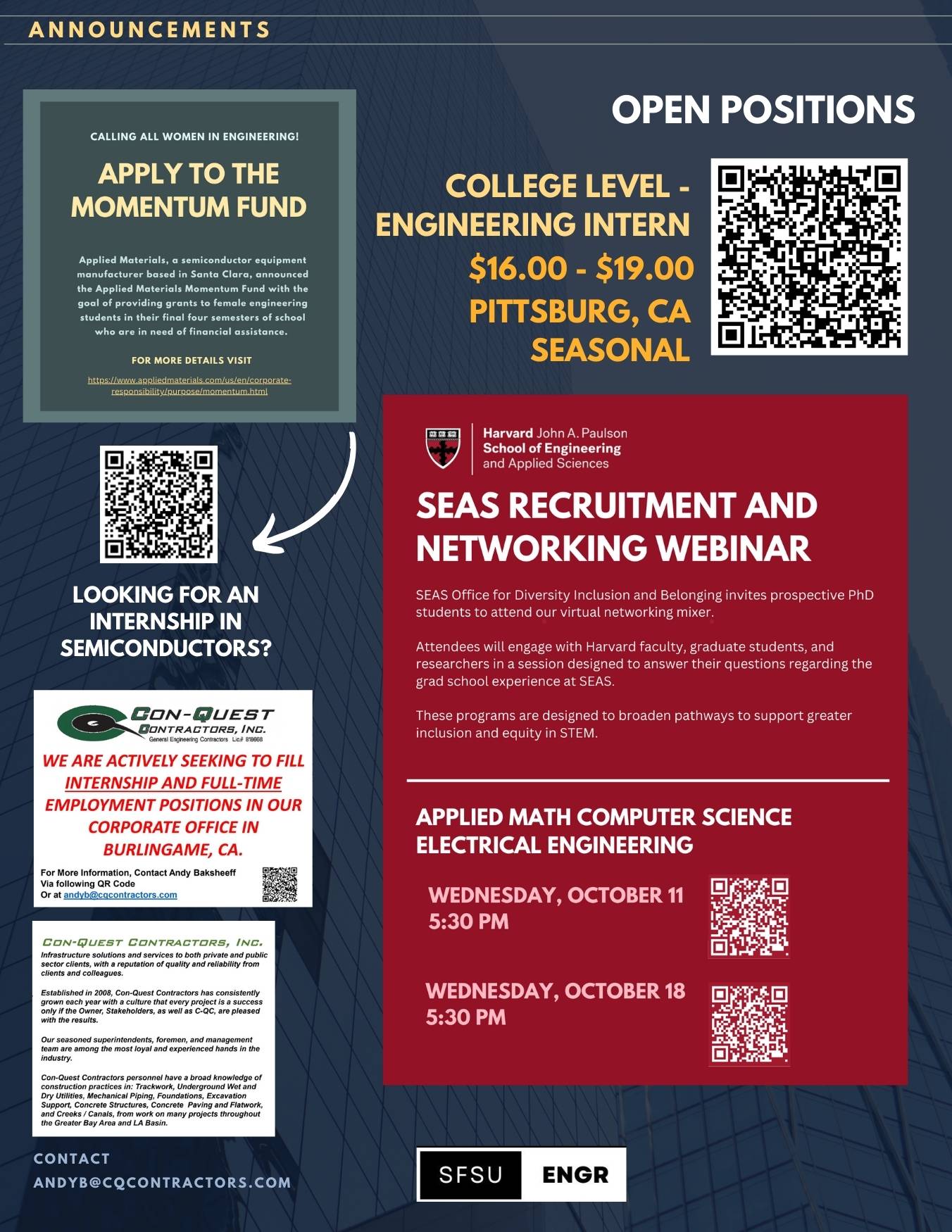
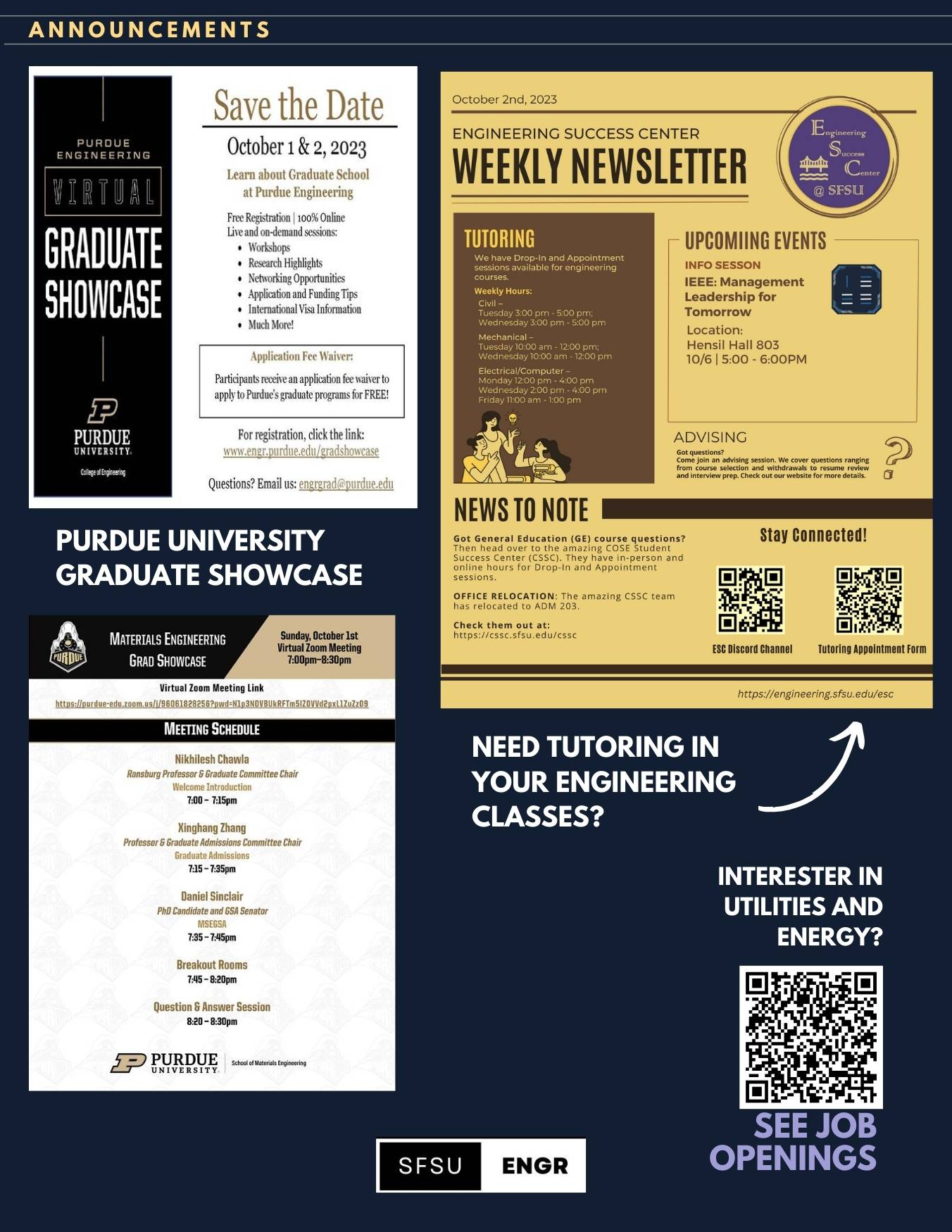
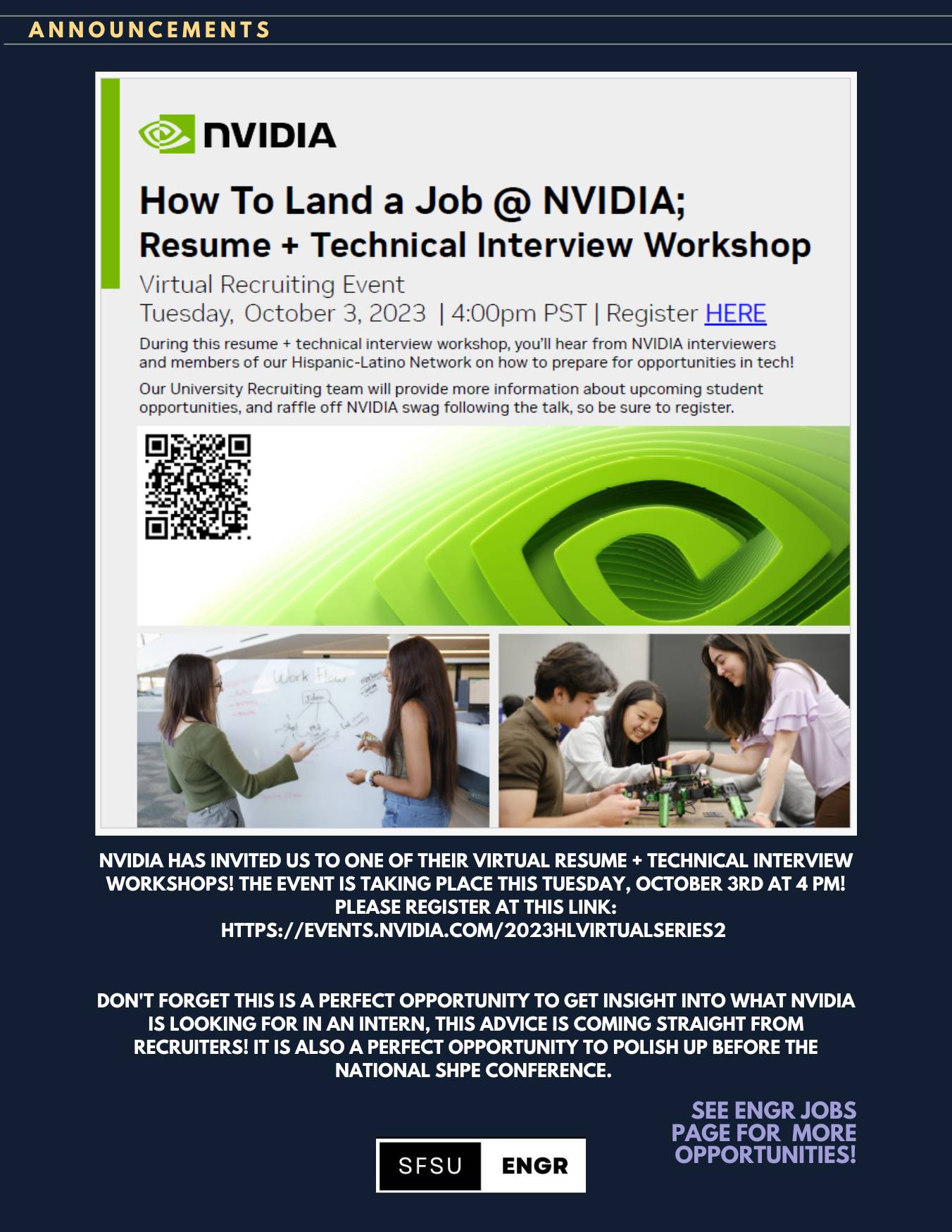
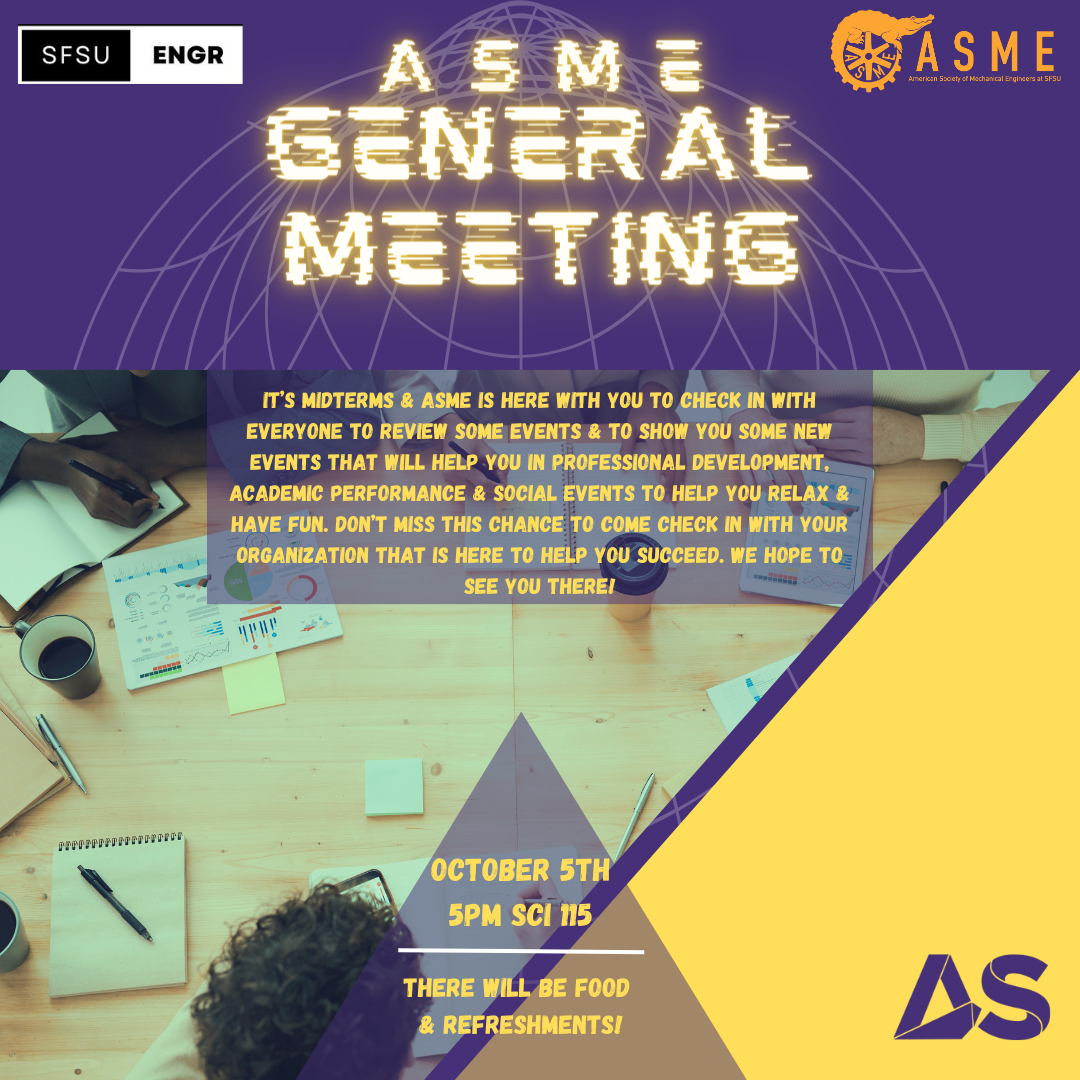
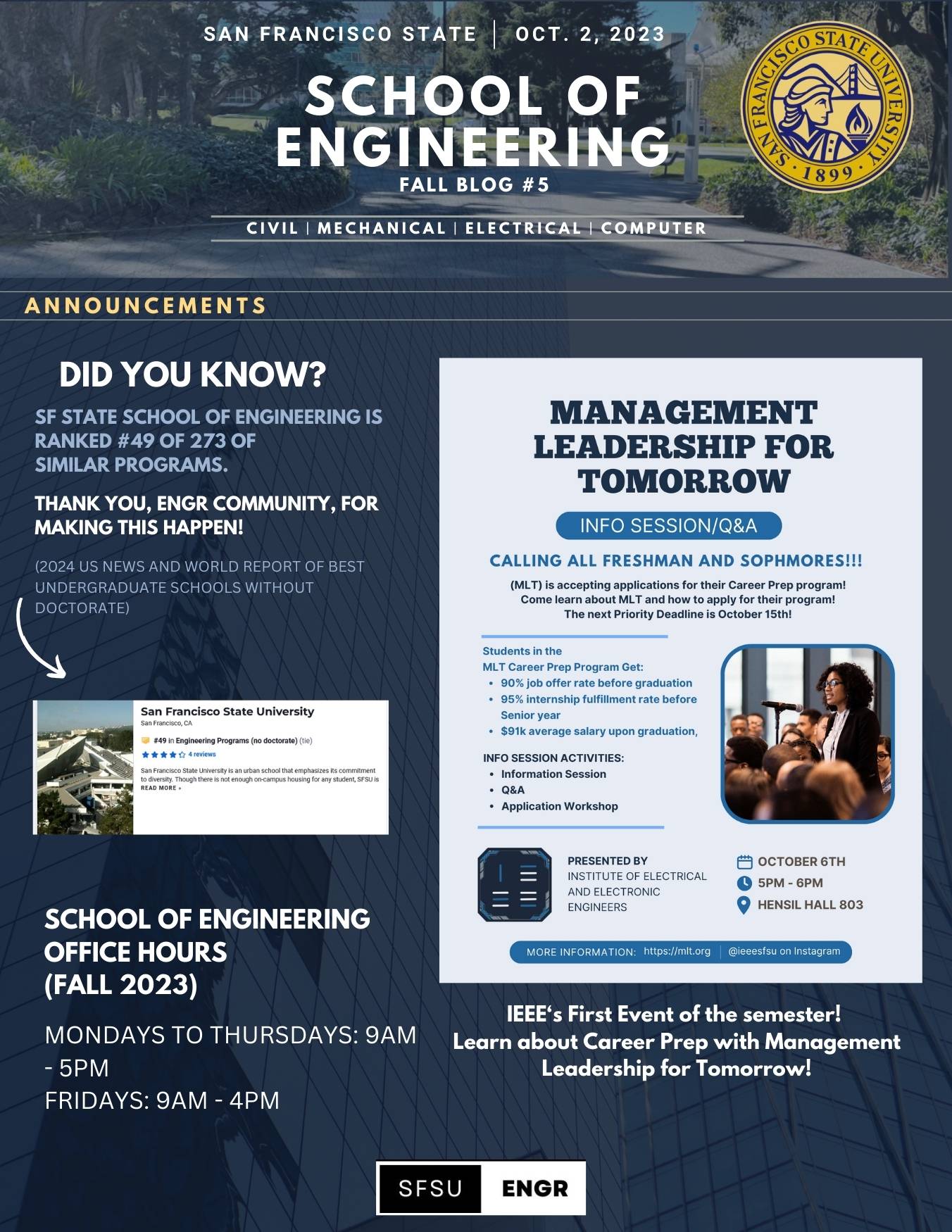
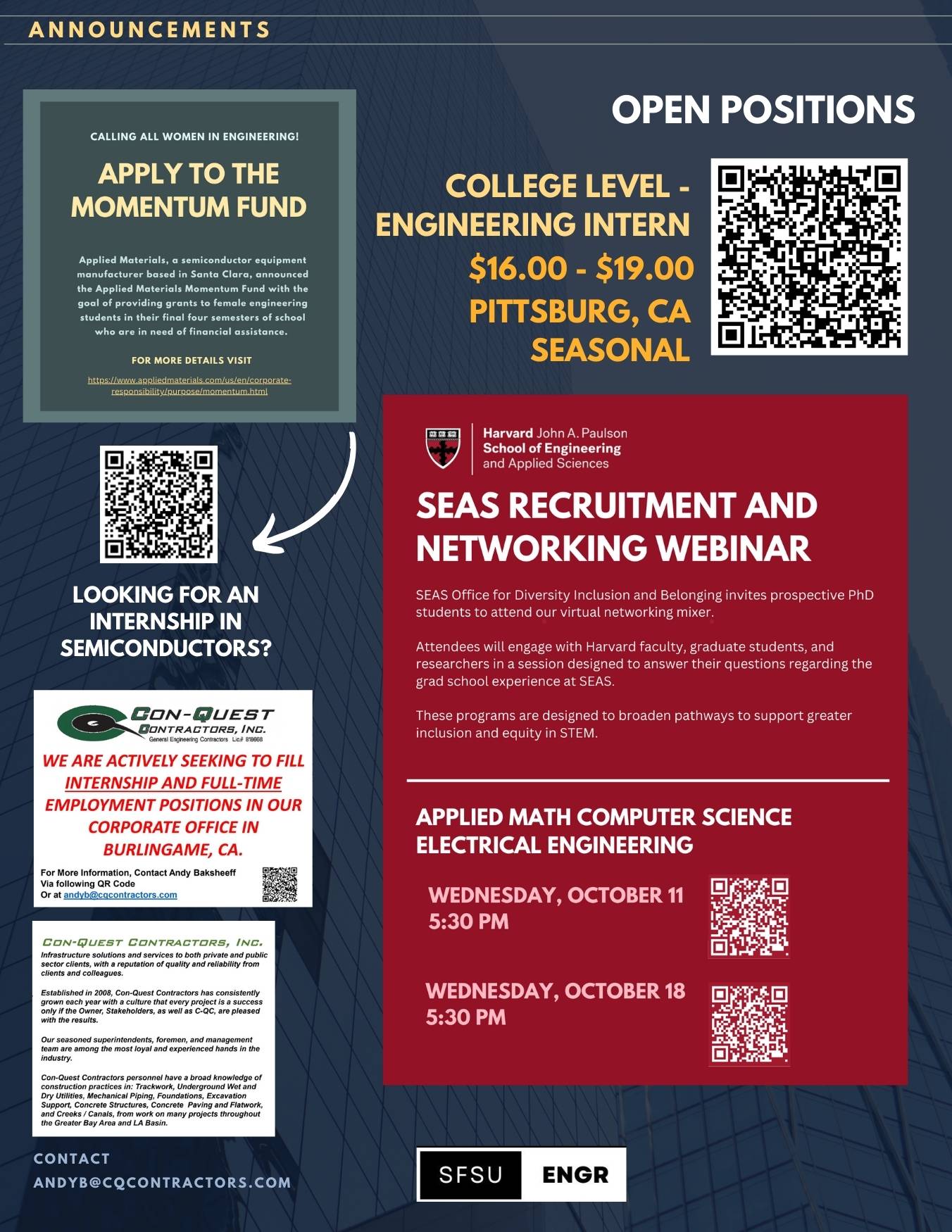
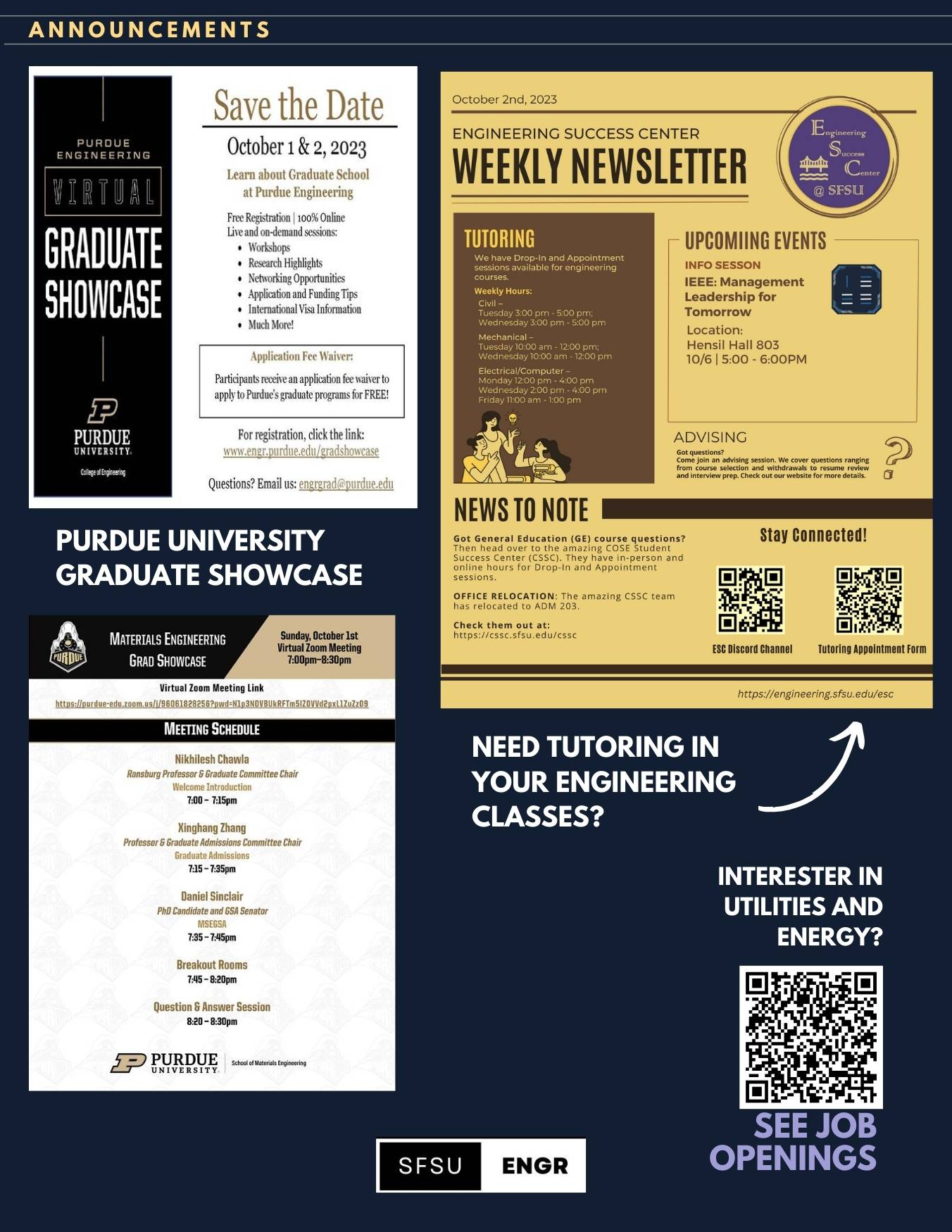
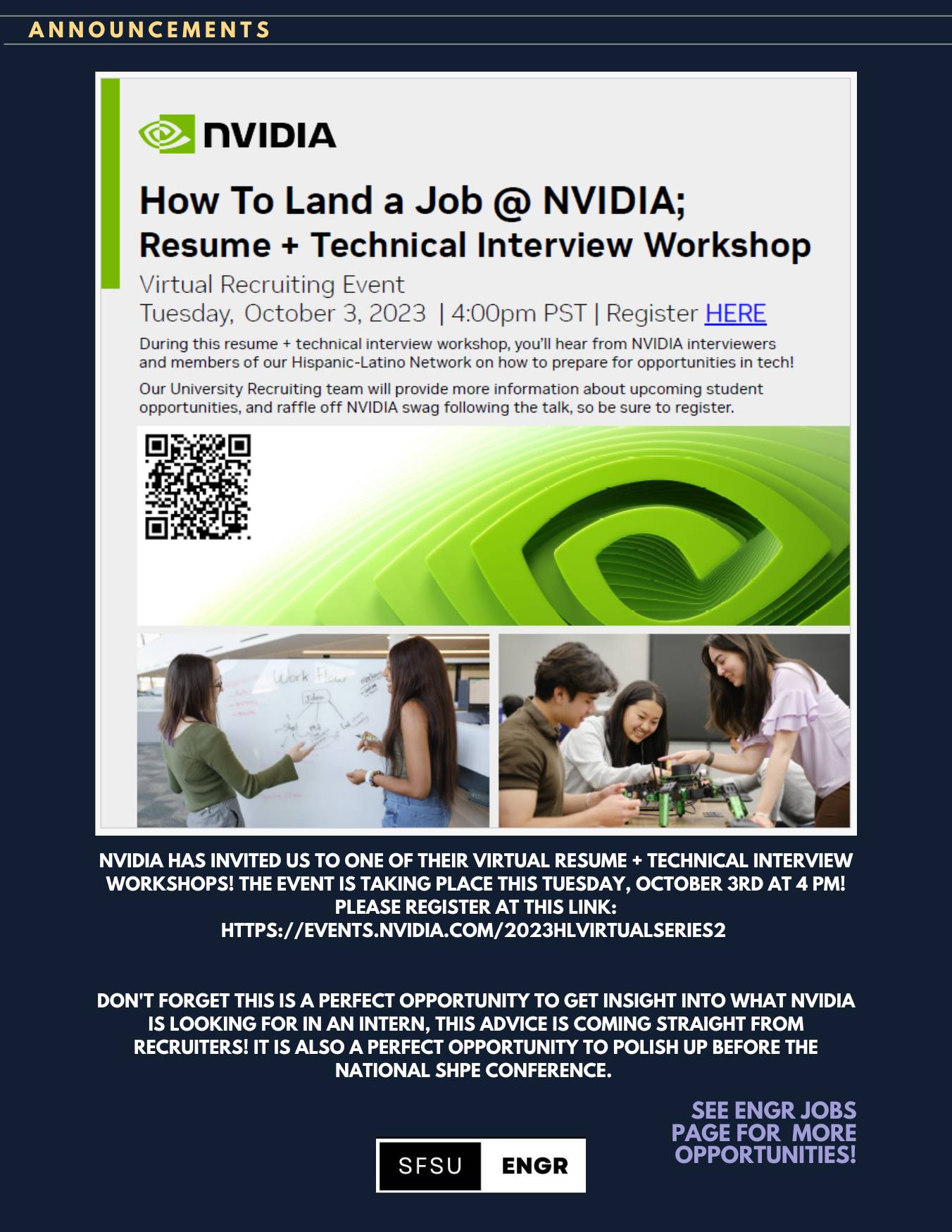
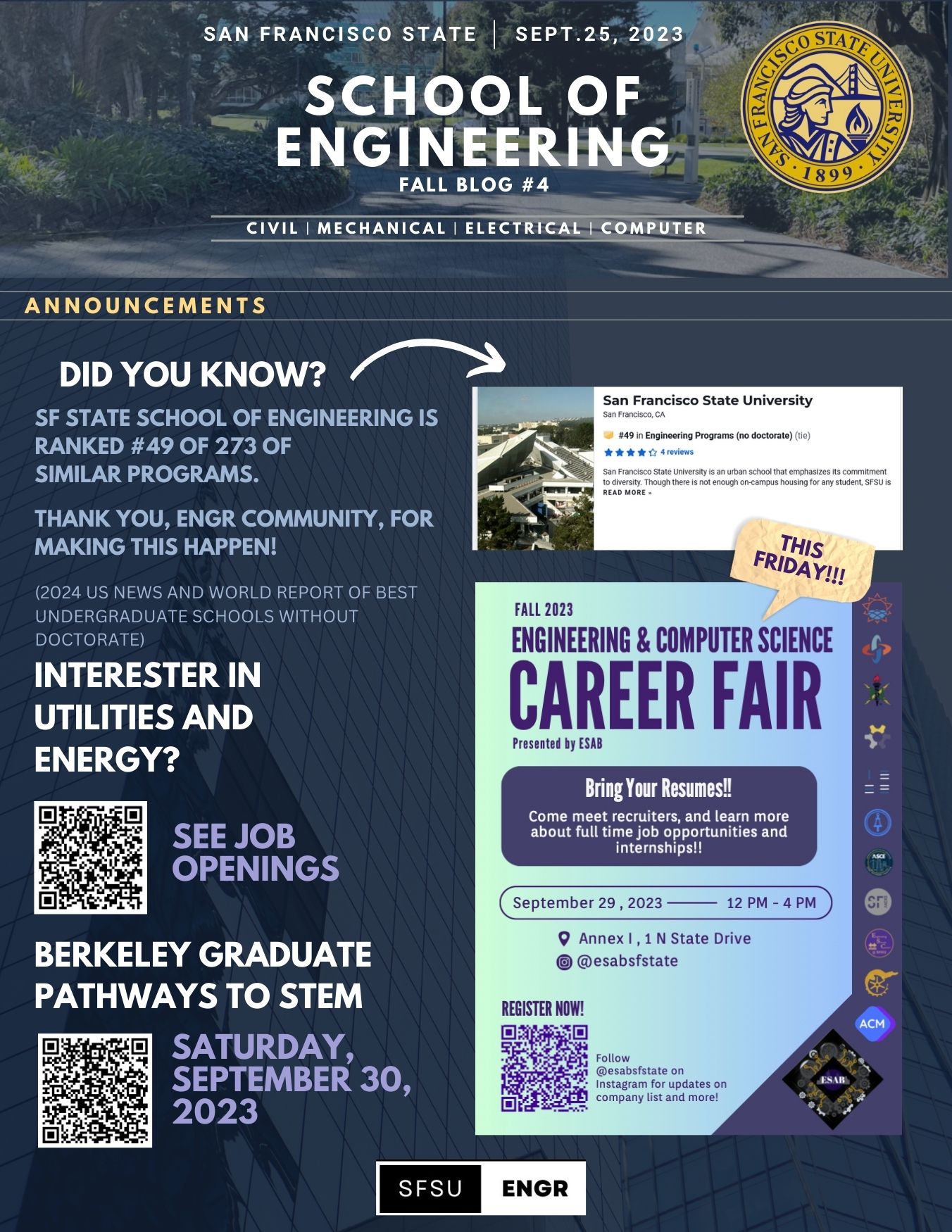
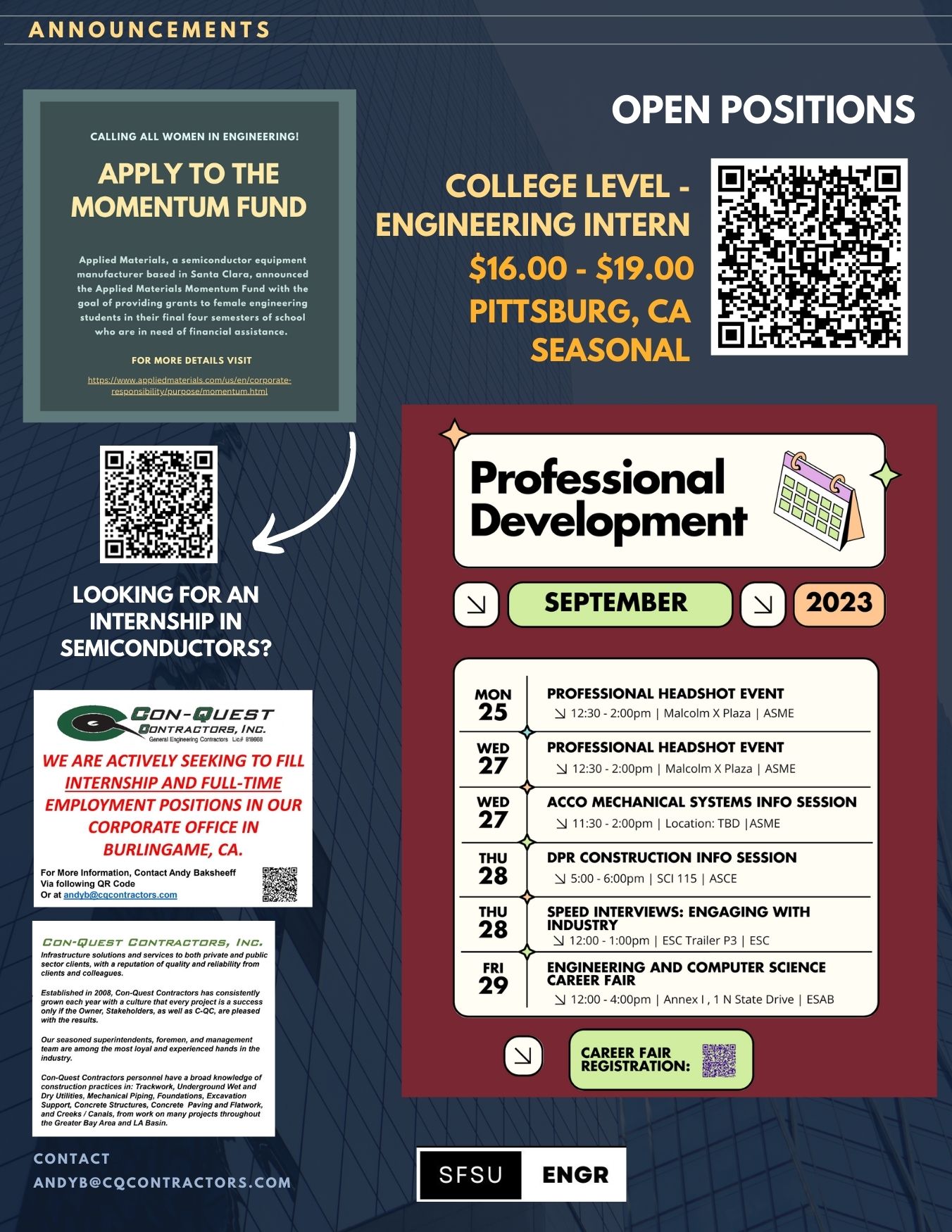
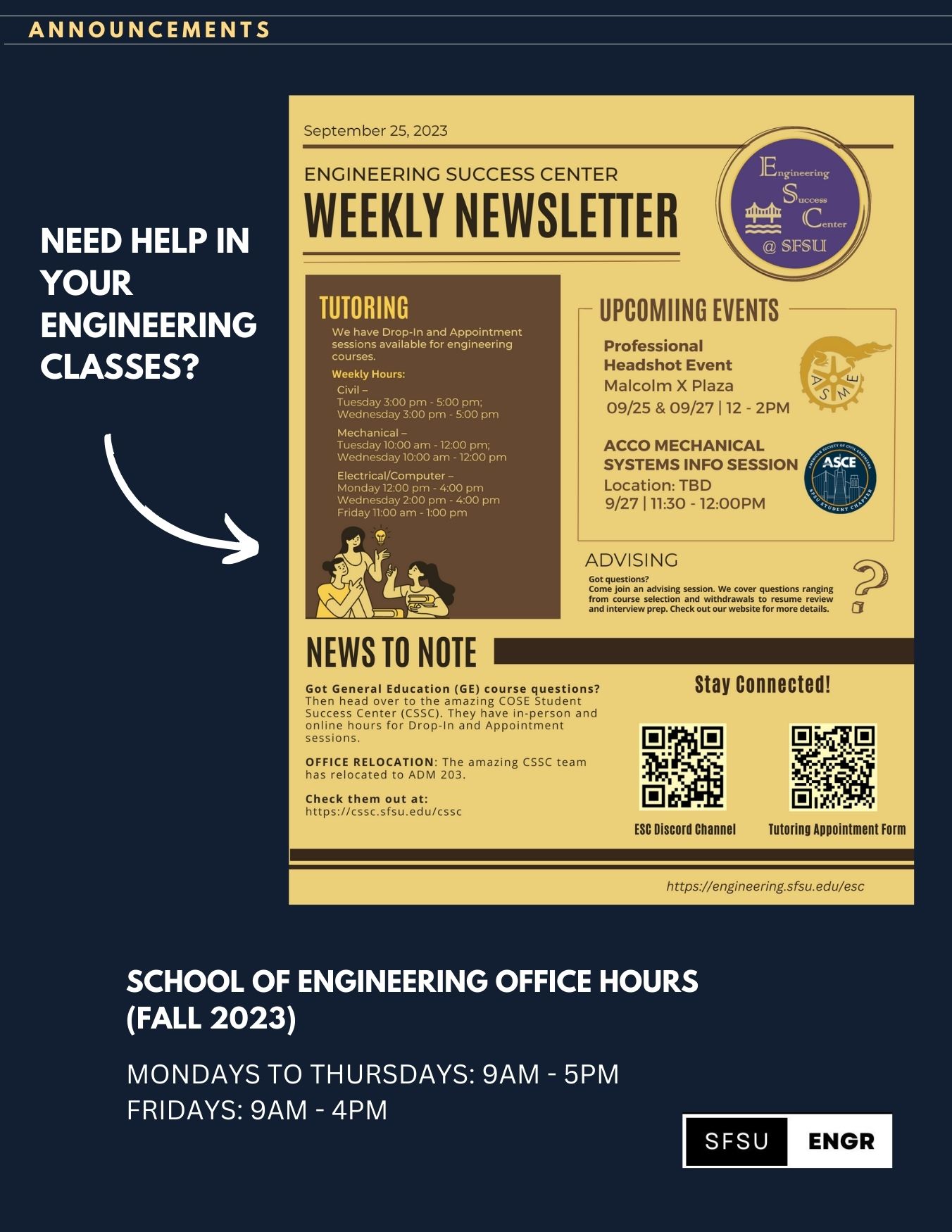
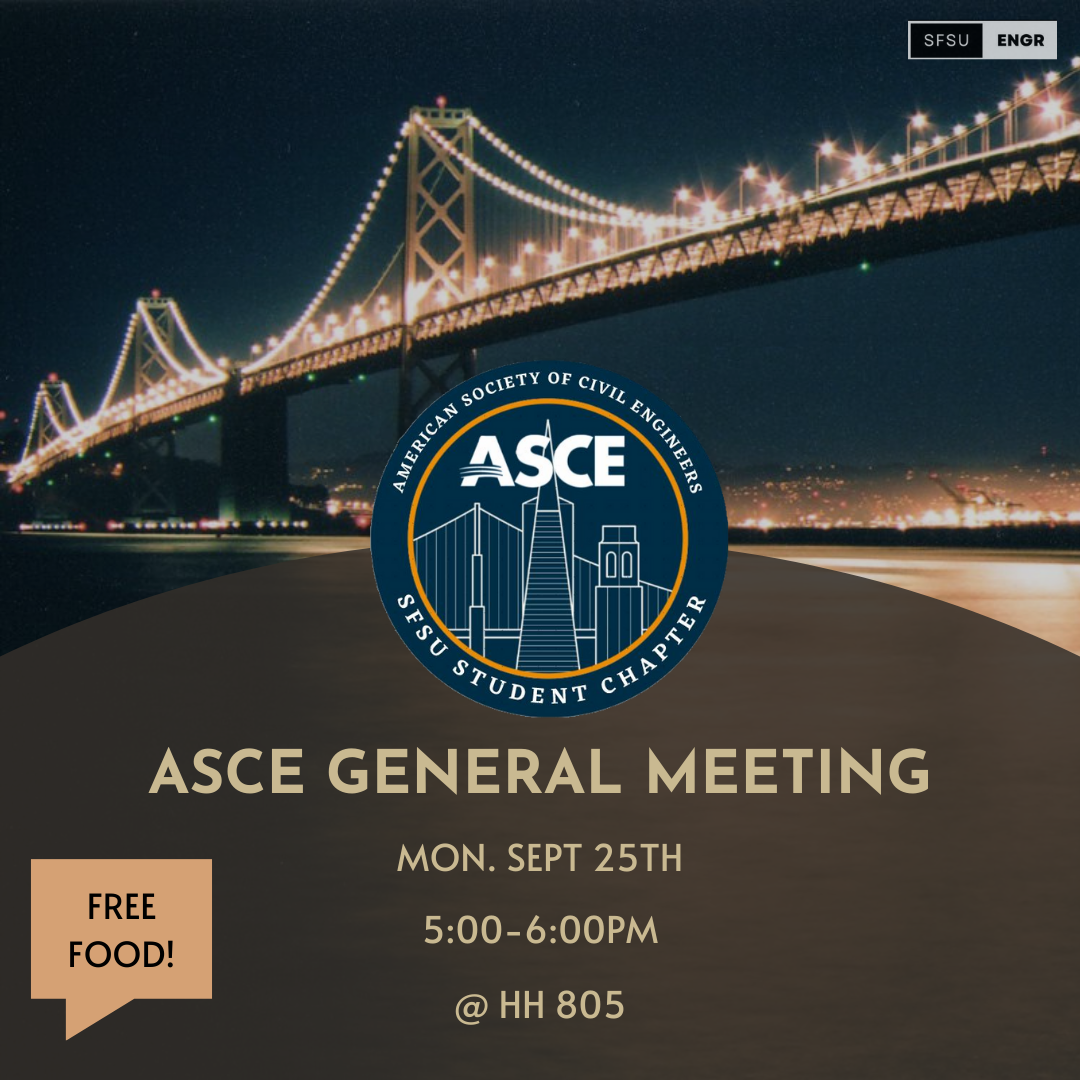
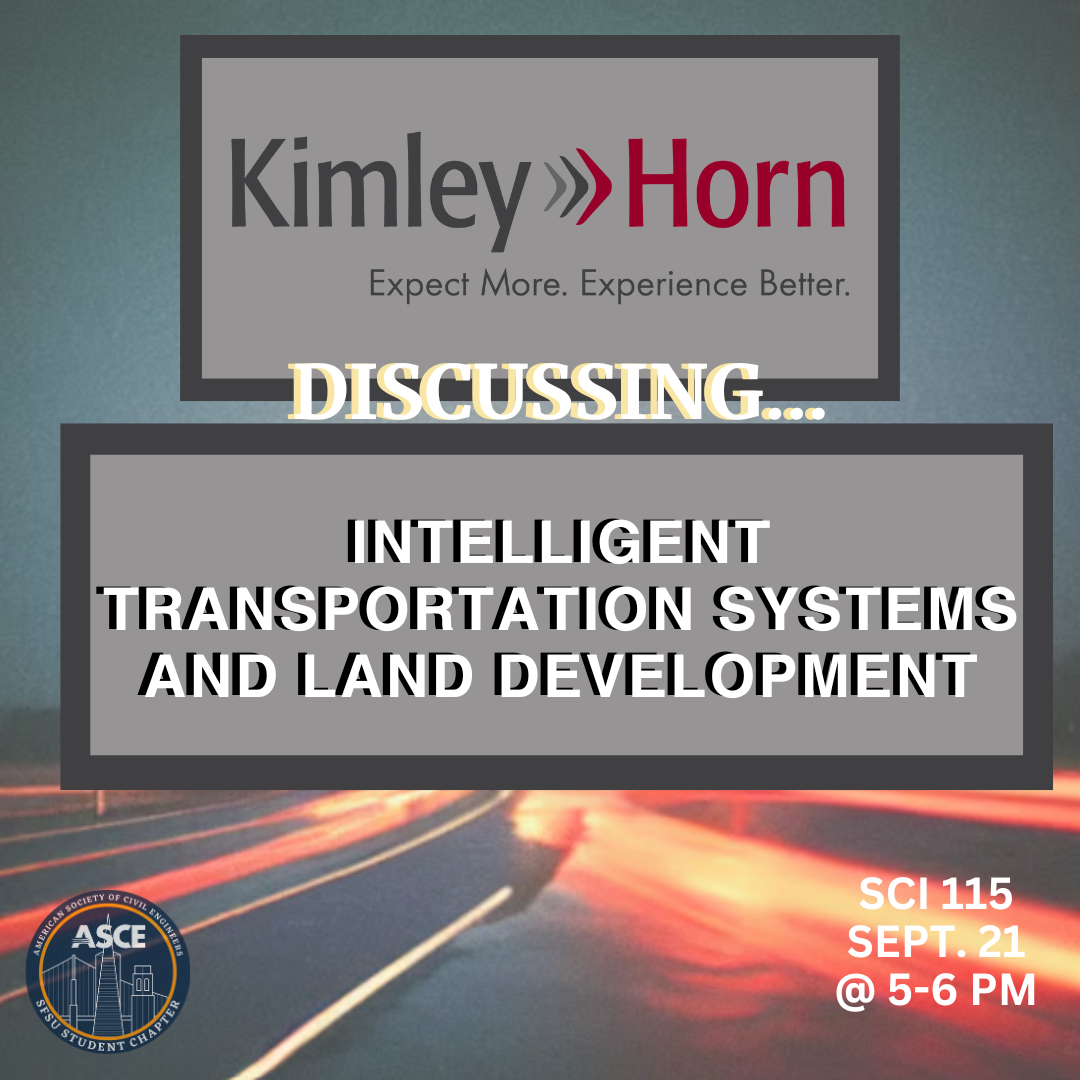
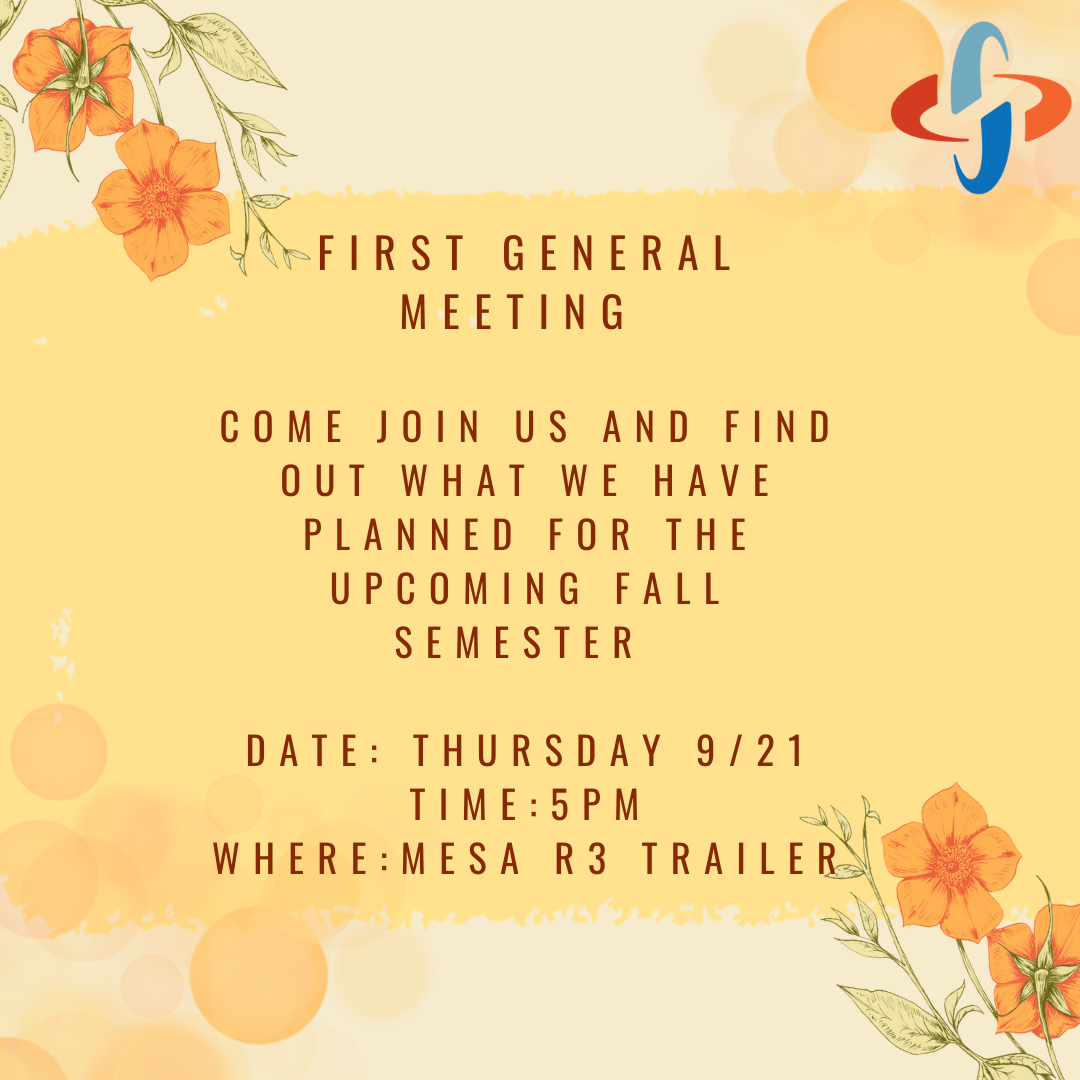
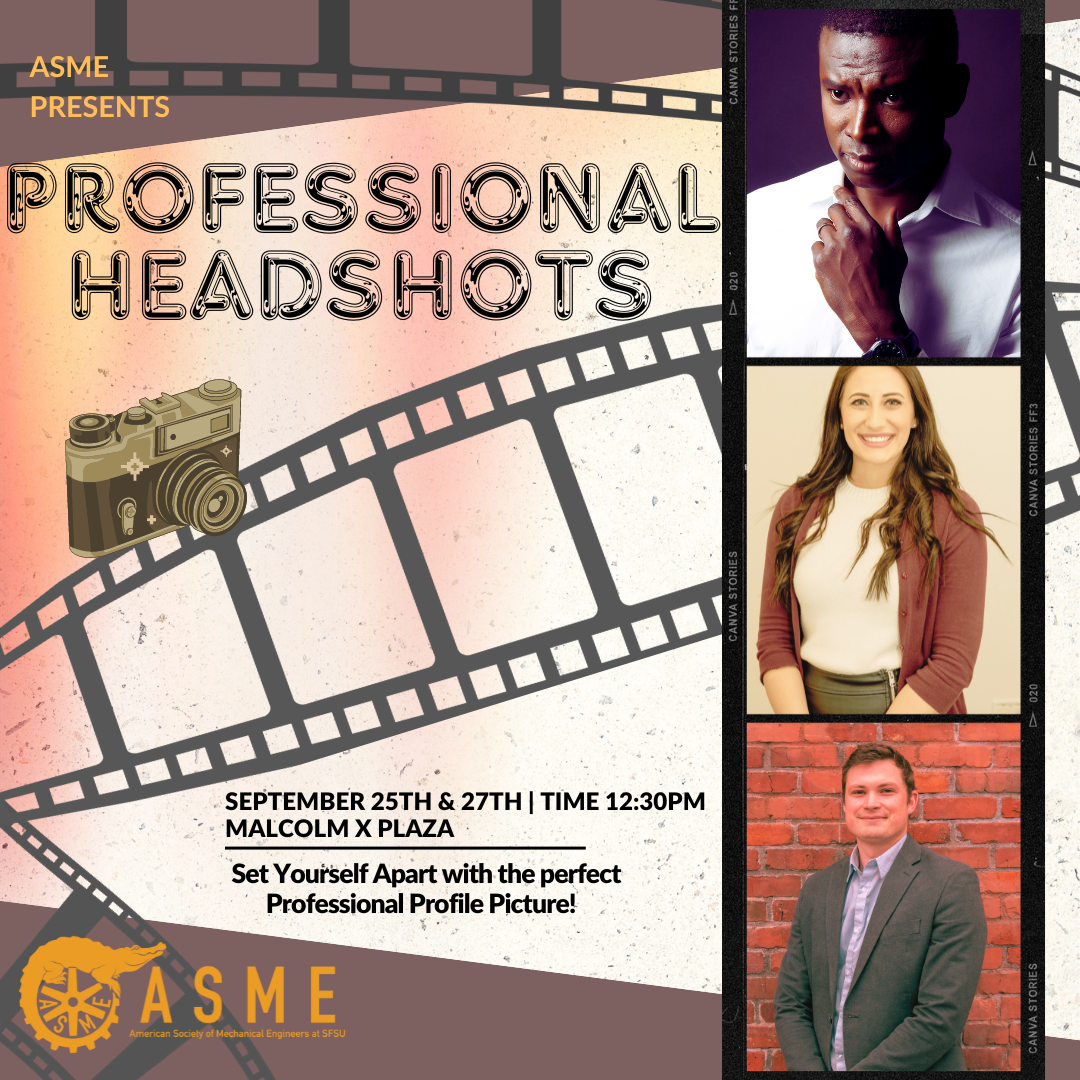
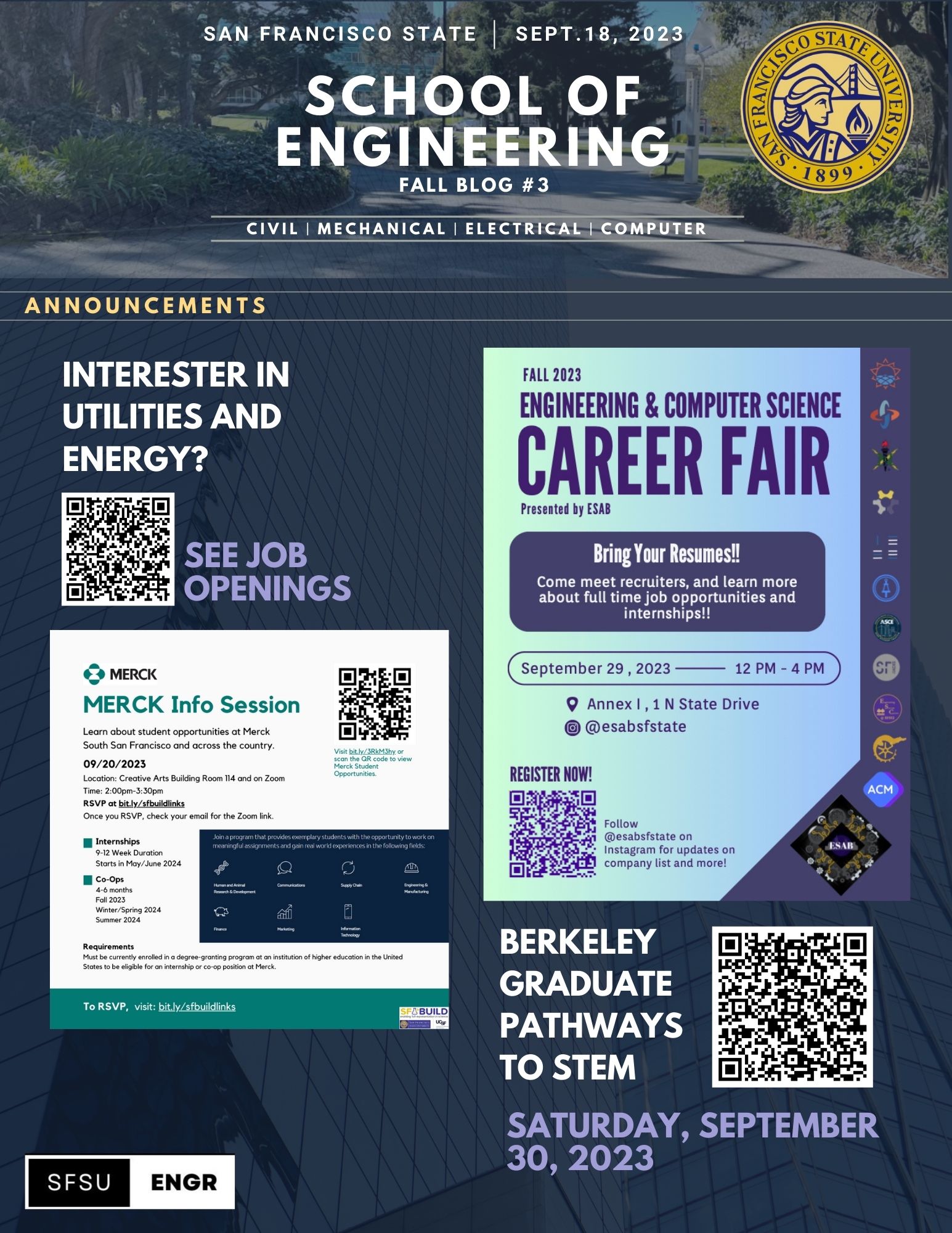
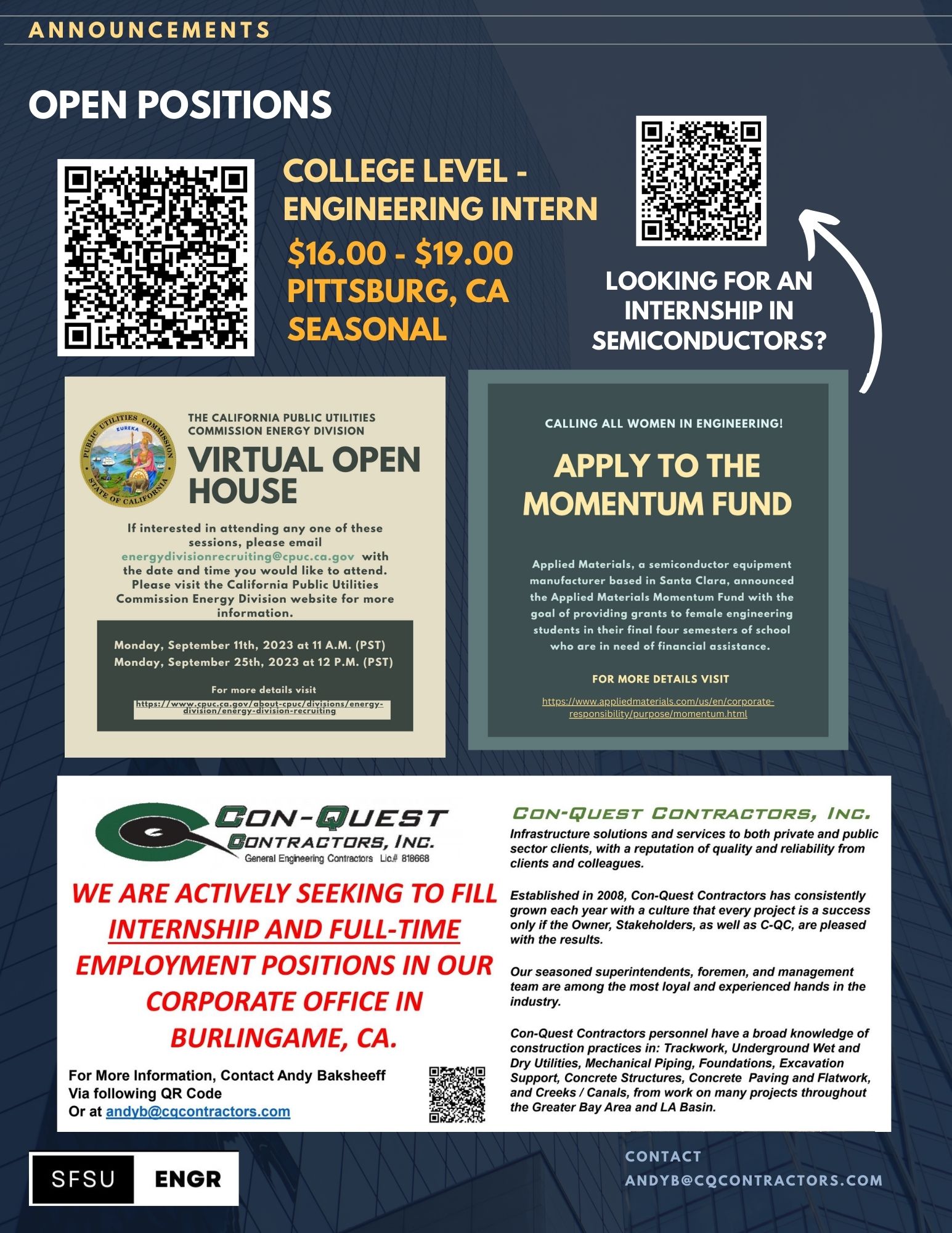
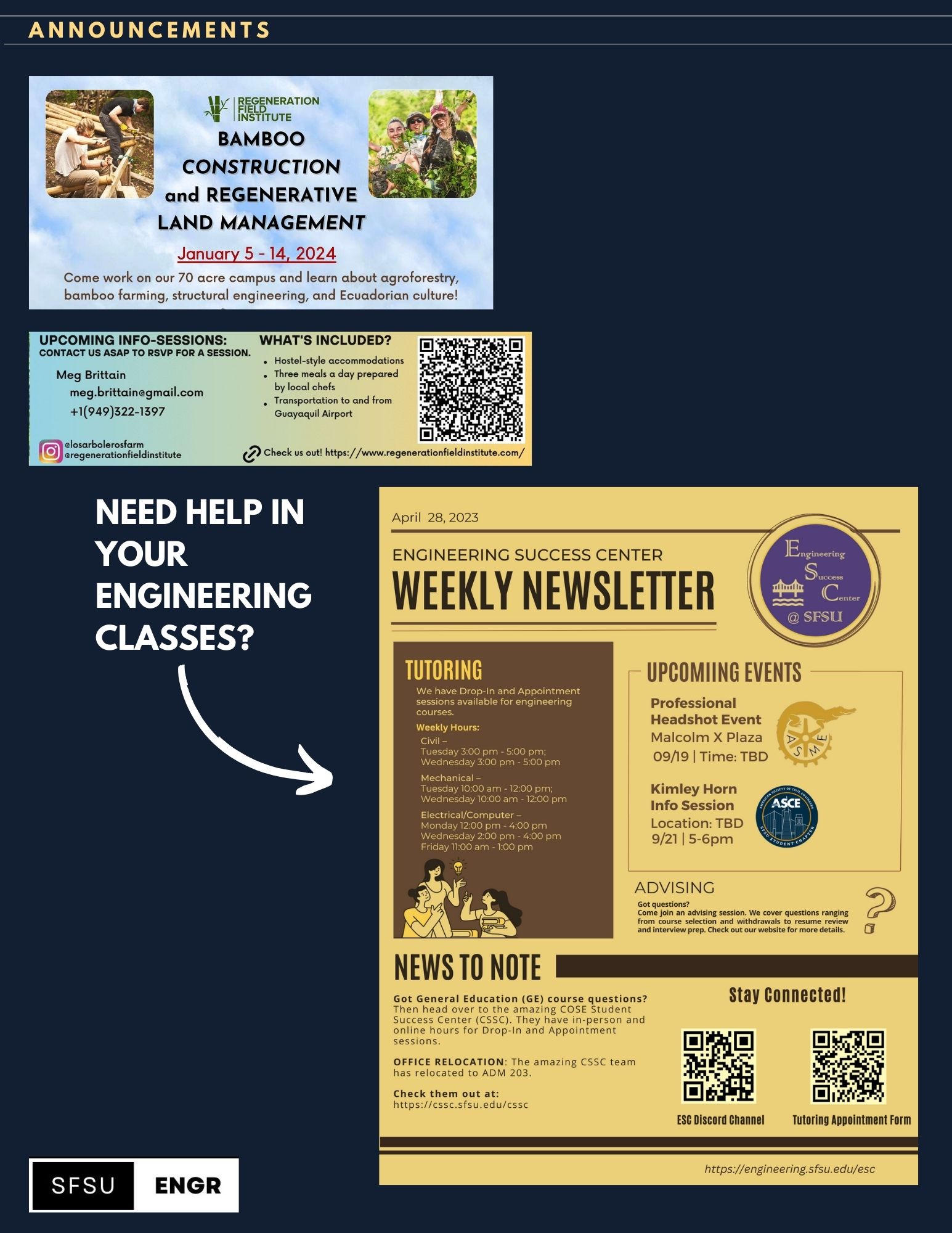
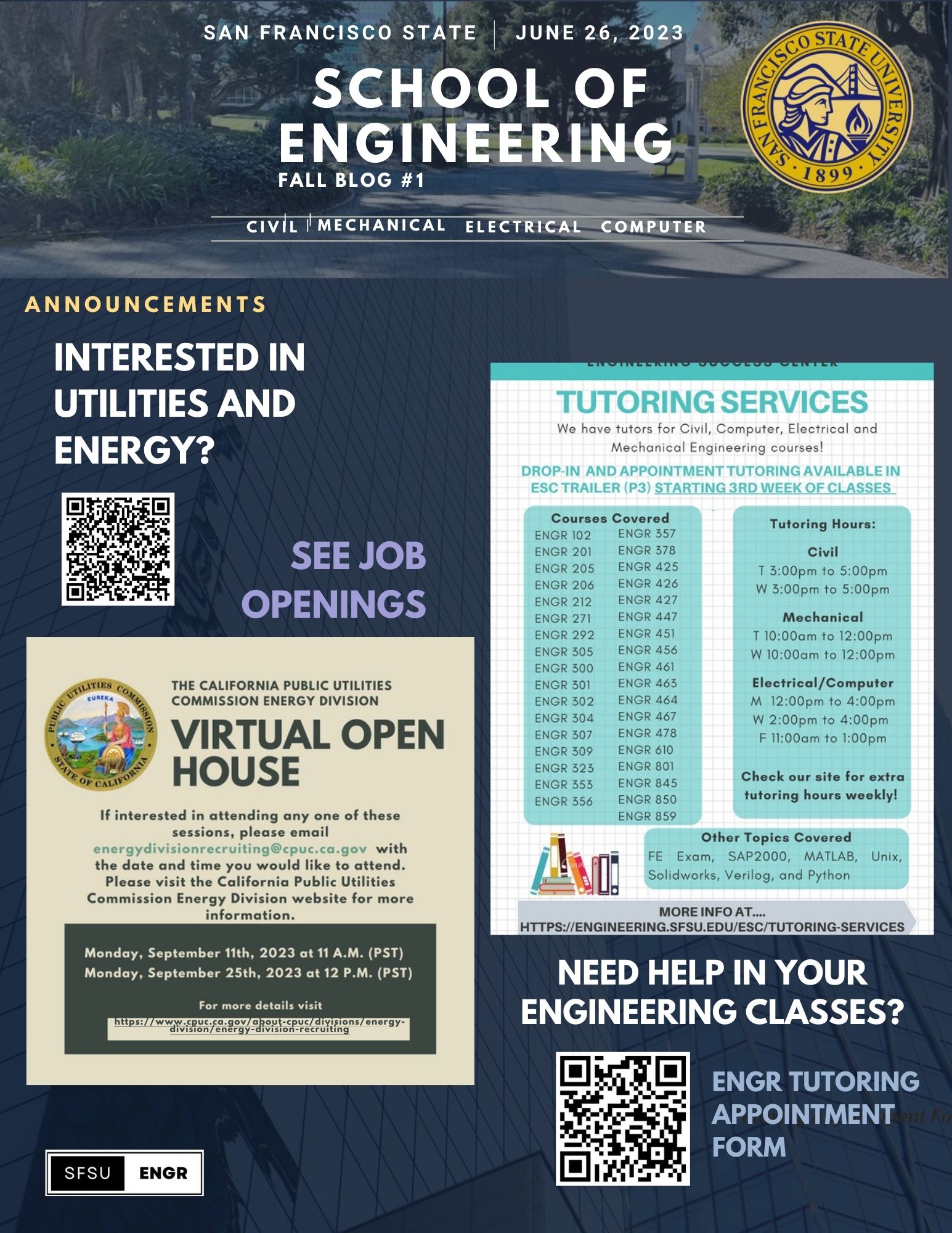
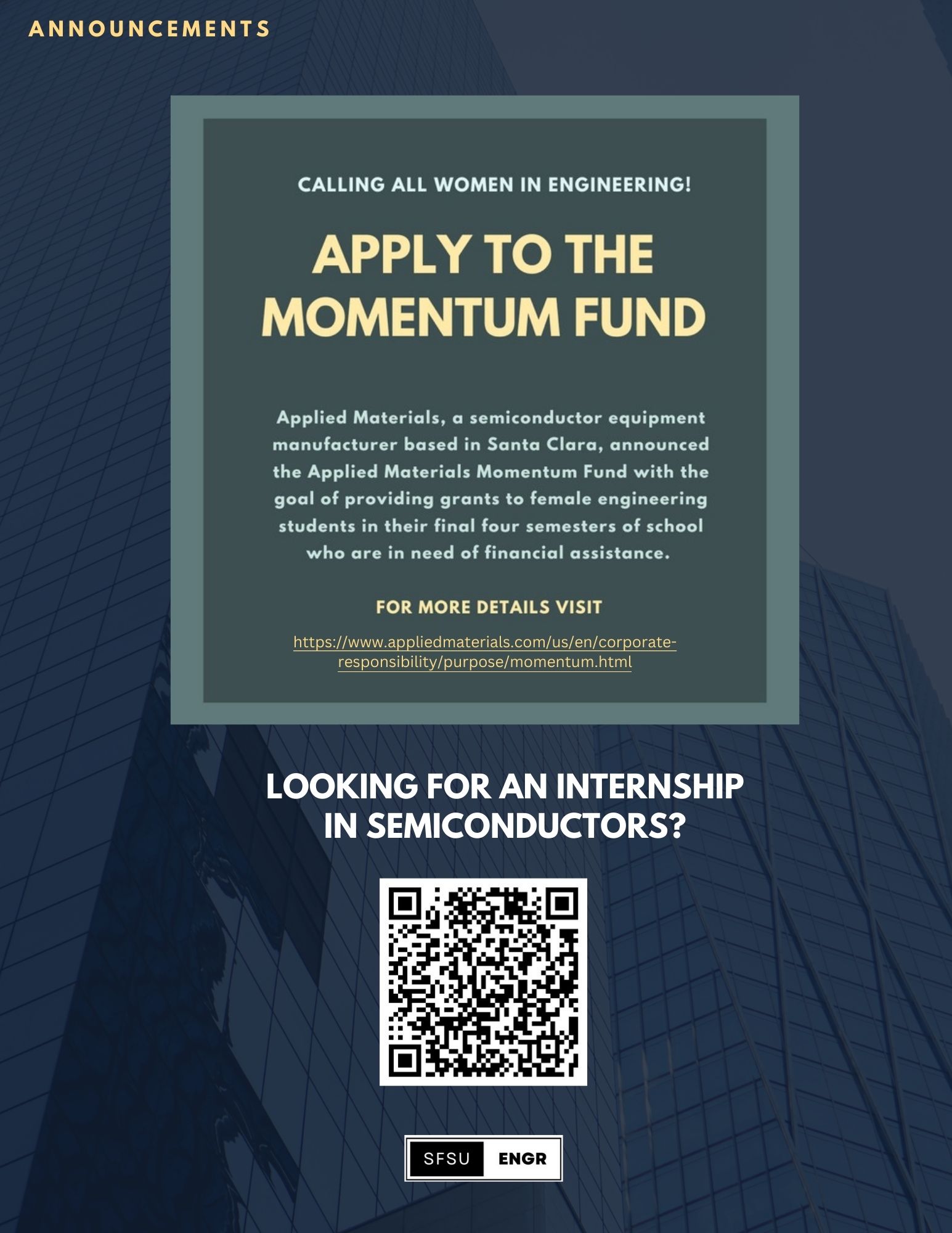
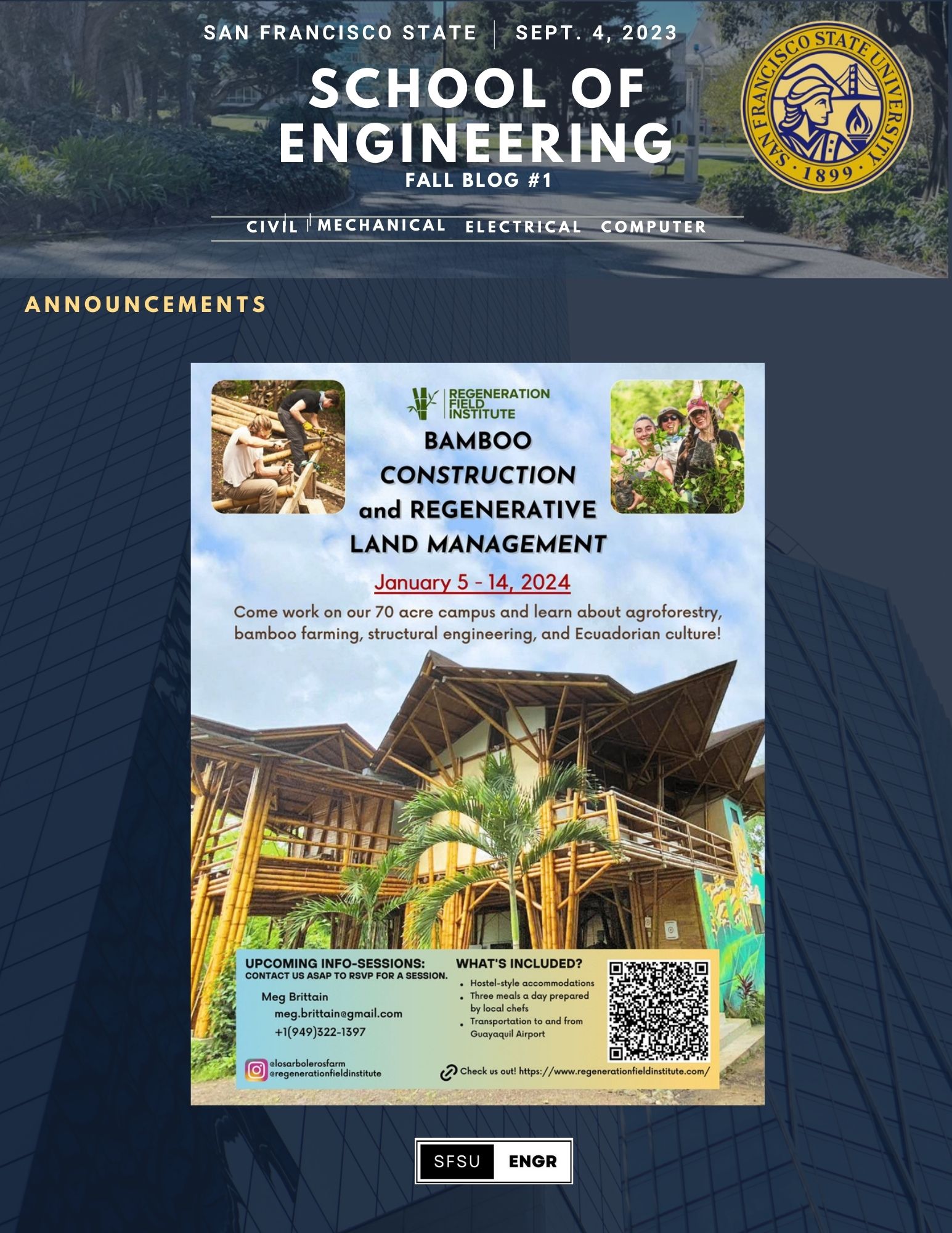
Summer 2023
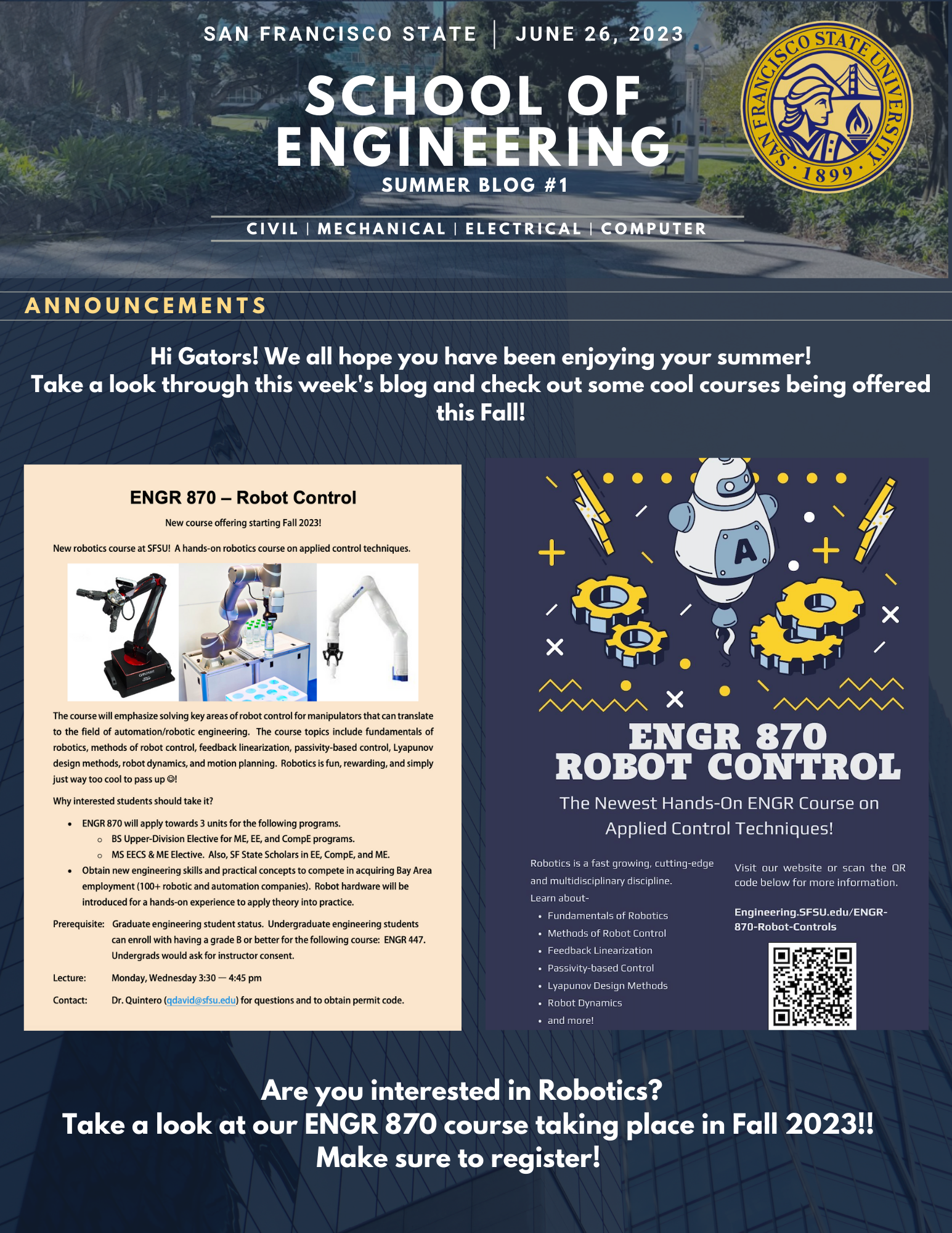
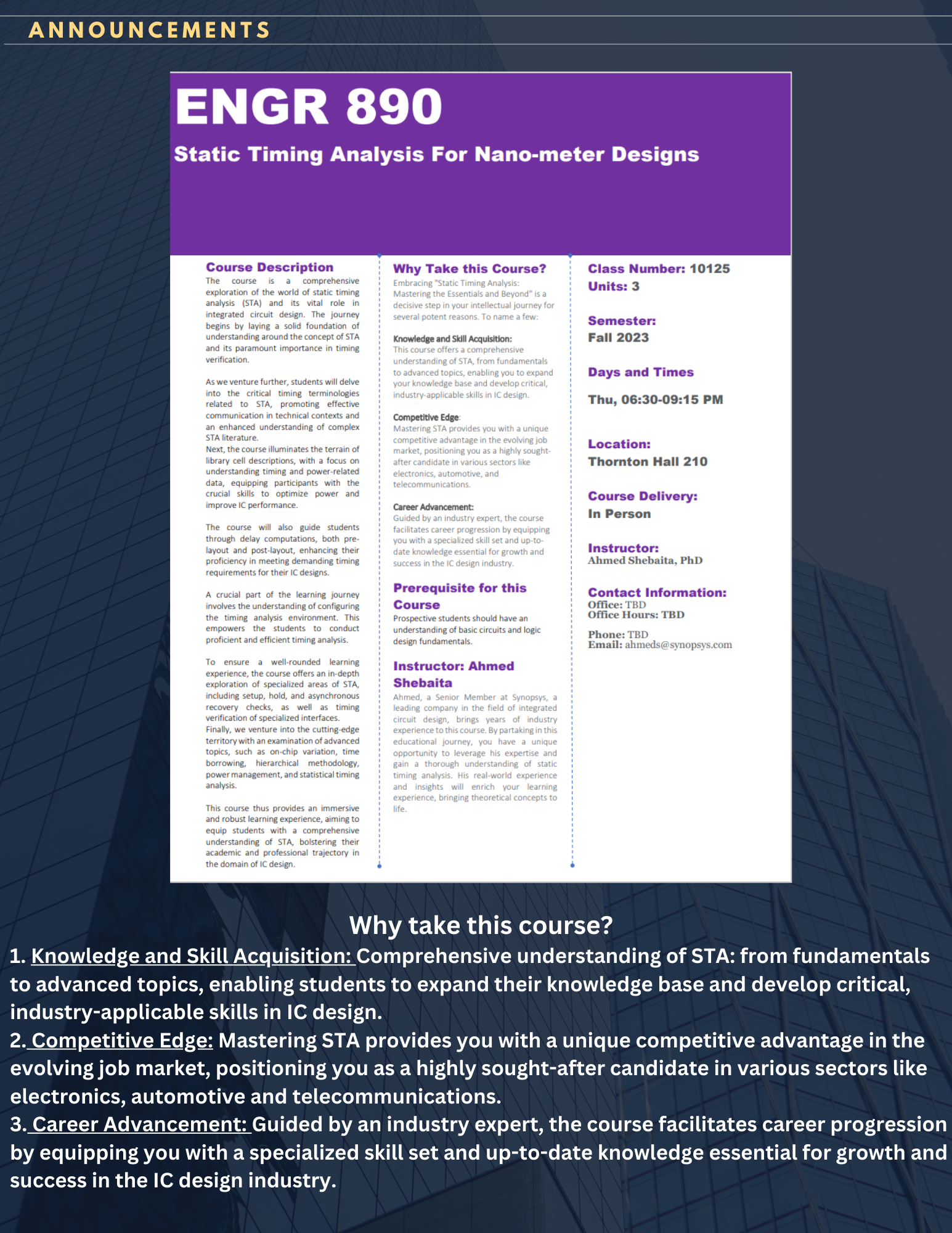
Spring 2023
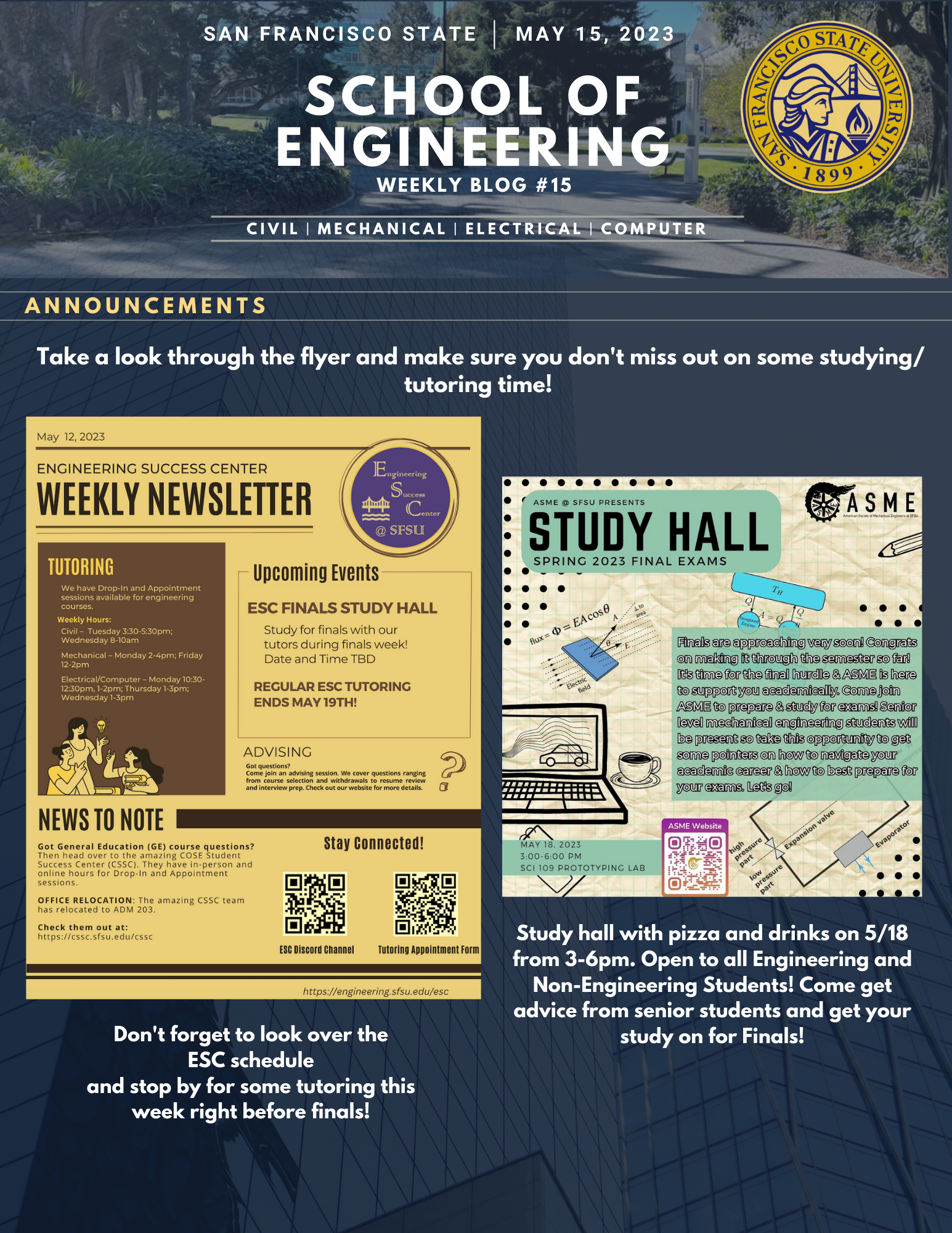
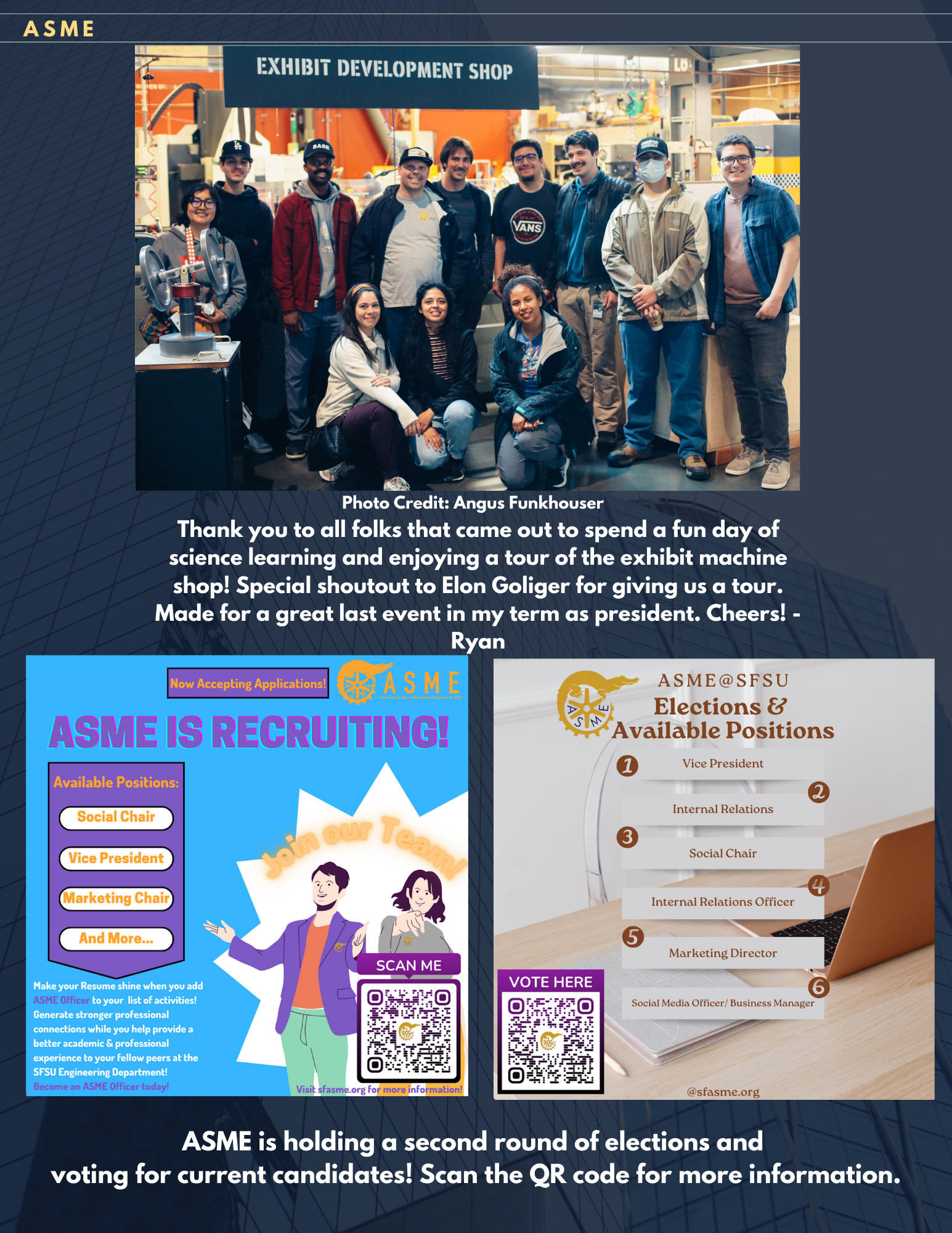
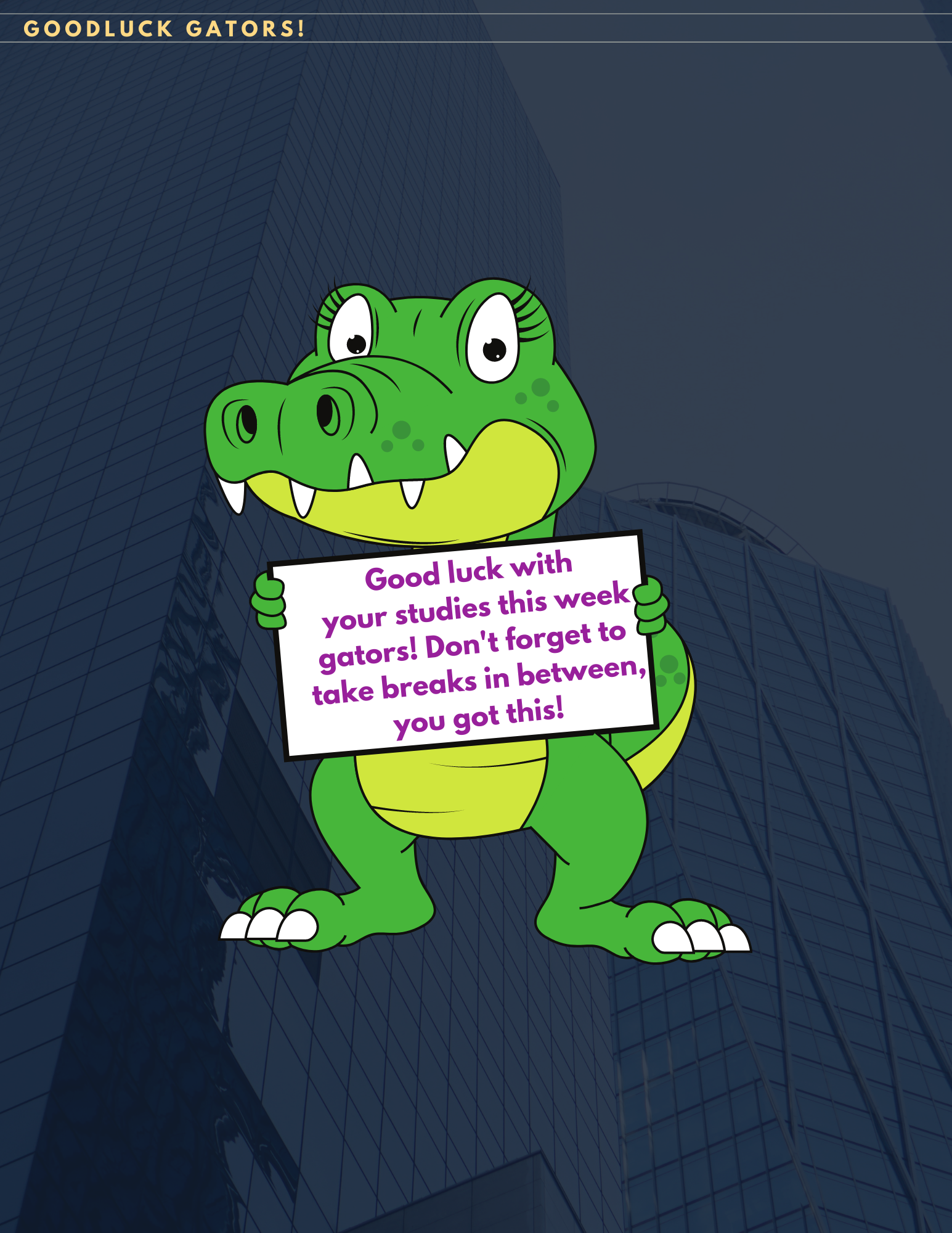
A Modular IR Beacon Sensor with Real-Time Feedback for Environmental Location
Presenter: Rodrigo Aceves
Time & Date: 10 AM, May 19th, 2023
Location: Zoom Meeting ID: 837 2124 2782 Passcode: 187876
Committee Chair: Dr. David Quintero
Committee Member: Dr. Xiaorong Zhang
ABSTRACT
This study introduces a modular IR beacon sensor system for environmental location tracking in mechatronics applications. The research aims to address the limitations of existing systems that primarily differentiate beacons by transmitting frequency information, while the proposed system communicates distance information. By employing Light Detection and Ranging (LiDAR) technology for distance measurements and using Pulse Width Modulation (PWM) for infrared wireless communication, the system offers an innovative approach to location tracking. The modular design incorporates a real-time feedback mechanism in the receiver portion, which leverages non-blocking functions on a microcontroller. Experimental results show that distance data is effectively communicated using PWM, simplifying circuitry and receiver programming without sacrificing precision. The proposed modular IR beacon sensor system provides a versatile and adaptable solution for mechatronics applications, enabling real-time and precise distance measurements using LiDAR technology and PWM-based infrared communication
Analysis of Wi-Fi Probe Request Bursts for Device Counting
Presenter: Taylor Artunian
Time & Date: 1pm, May 18, 2023
Location: Zoom (Link below)
Meeting ID: 837 2124 2782 Passcode: 187876
Committee Chair: Dr. Xiaorong Zhang
Committee Member: Dr. Yiyi Wang
ABSTRACT
With urban populations growing worldwide, the need for accurate counts of pedestrians and other forms of traffic is increasingly important for infrastructure development. Many of the existing solutions involving LiDAR or cameras are expensive, immobile and invade privacy. However, researchers have been attempting to solve this problem by passively listening to Wi-Fi packets known as Probe Requests. As a technology, Wi-Fi does not suffer the same drawback as the previously mentioned ones: it is inexpensive, very mobile and does not directly reveal personally identifiable information. Despite its advantages, the main challenge in using Wi-Fi is the introduction of MAC address randomization. Wi-Fi devices periodically send out a series of packets known as Probe Requests to connect to a network but with MAC address randomization, these packets no longer have a consistent, unique identifier. Instead, each group of packets, known as a burst, uses a random MAC address. To get around this issue, researchers have focused on new ways to correlate Probe Requests from the same device through the use of data other than the MAC address. This includes fields such as RSSI, 802.11 channel, frame length, and Information Elements. Our research has expanded upon this work by defining new characteristics which look not at individual Probe Requests, but at Probe Request bursts instead. Observing devices in electromagnetic isolation has allowed us to discover and calculate these characteristics as well as compare them between phones. Our results show that these characteristics are produced from stable distributions for individual phones across time and are also significantly different between different phones. It is our belief that the usage of Burst Level Characteristics in a machine learning model could enable model-type identification and even individual device identification.
A Probabilistic- Based Approach to Phase Variable Estimation for Lower Limb Prostheses Control
Electrical and Computer Engineering
Master Oral Defense
Presenter: Lakshmi Priya Jeevagan
Time & Date: 9-10 AM, May 16th, 2023
Location: HH803
Thesis Advisor: Dr. David Quintero
Thesis Committee: Dr. Xiaorong Zhang, Dr. Hamid Mahmoodi
Zoom Info:
Meeting ID: 821 2362 4549
Passcode: 503295
Abstract
A mechanical phase variable represents human gait progression that can parameterize the joint kinematic trajectories for lowerlimb prostheses control. We propose a probabilistic-based approach that uses a maximum likelihood estimation technique to derive a holonomic phase variable for a continuous gait phase estimation. We evaluated the proposed phase variable algorithm across various walking speeds using IMU sensor to measure thigh angle, and introduced start and stop transitions to evaluate robustness for non-rhythmic behaviors. The analysis demonstrates a probabilistic adaptation for correcting gait phase abnormalities that can drive locomotion progression for lower-limb prostheses control
A Review of Methods for Estimating Population Density in Public Spaces Using Wi-Fi Probe Requests Despite MAC Randomization
Electrical and Computer Engineering
Master Oral Defense
Presenter: Darren Newell
Time & Date: 10 AM, May 12th, 2023
Location: https://sfsu.zoom.us/j/85924377102?pwd=cHdyWmNMSkVNQ054NzFab1ZwaXE0Zz09
Committee Chair: Dr. Xiaorong Zhang
Committee Members: Dr. Yiyi Wang
Abstract
Our study assessed the effectiveness of Wi-Fi probe request data as a low-cost tool for crowd estimation. In our experiments, probe request data captured from five phone models under a variety of scenarios isolated in a Faraday Cage was analyzed. Additionally, existing research and analysis methods from five papers were reviewed and analyzed, informed by our own data. The findings were that some of the existing counting methods proposed in the reviewed literature do not accurately account for the behavior of phones employing probe request randomization techniques, also known as MAC randomization. These counting methods still hold promise for reliable crowd estimation, but they need to account for variability using probability or a corrective factor.
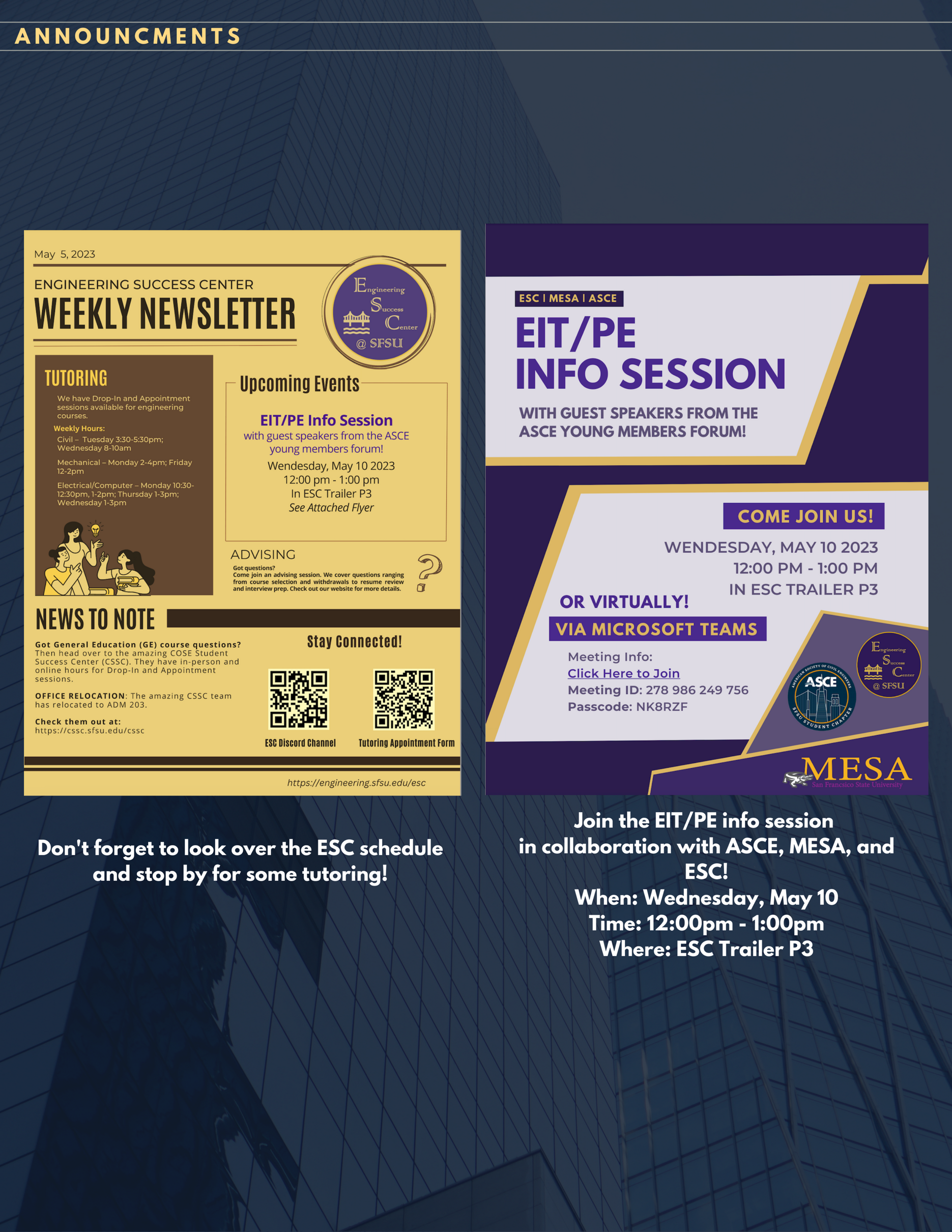
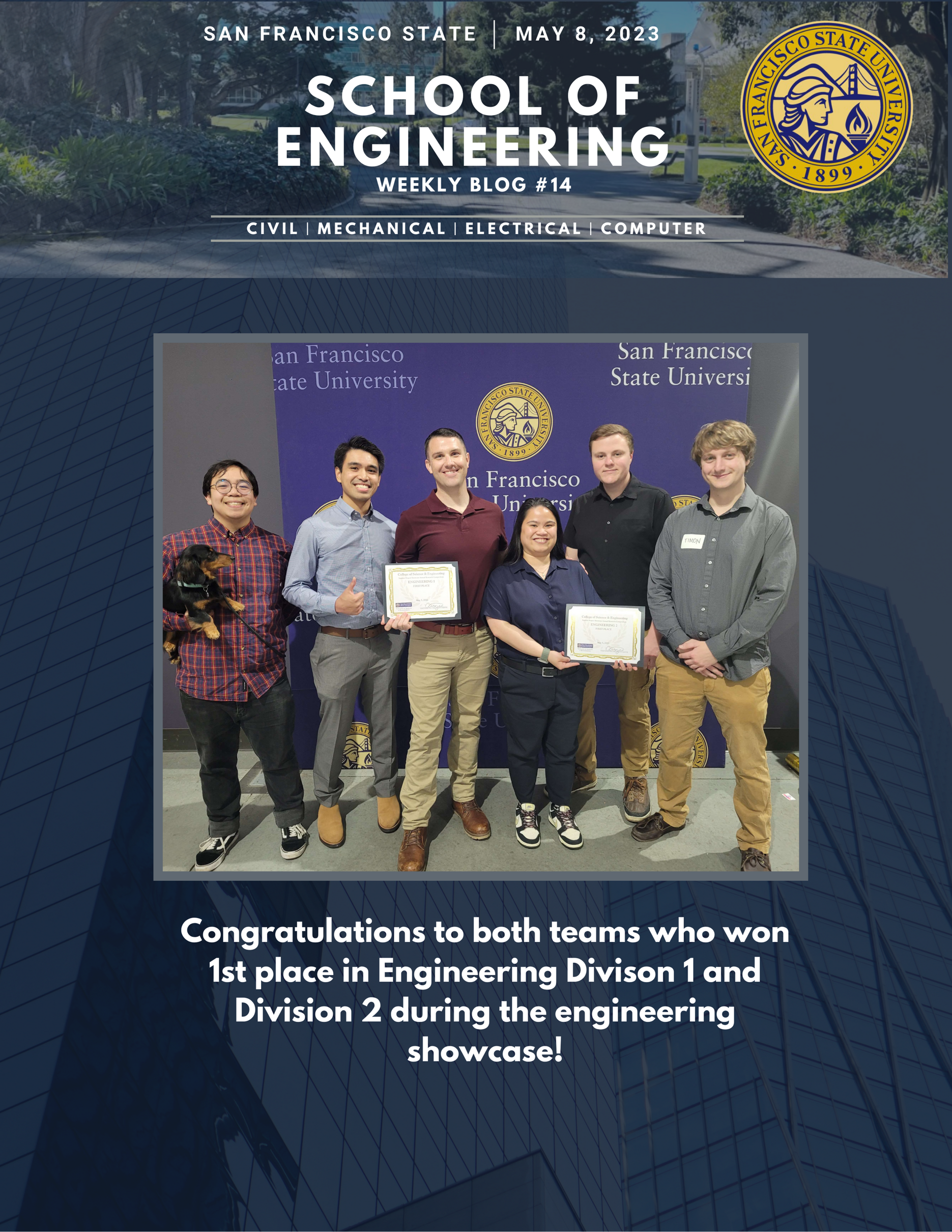
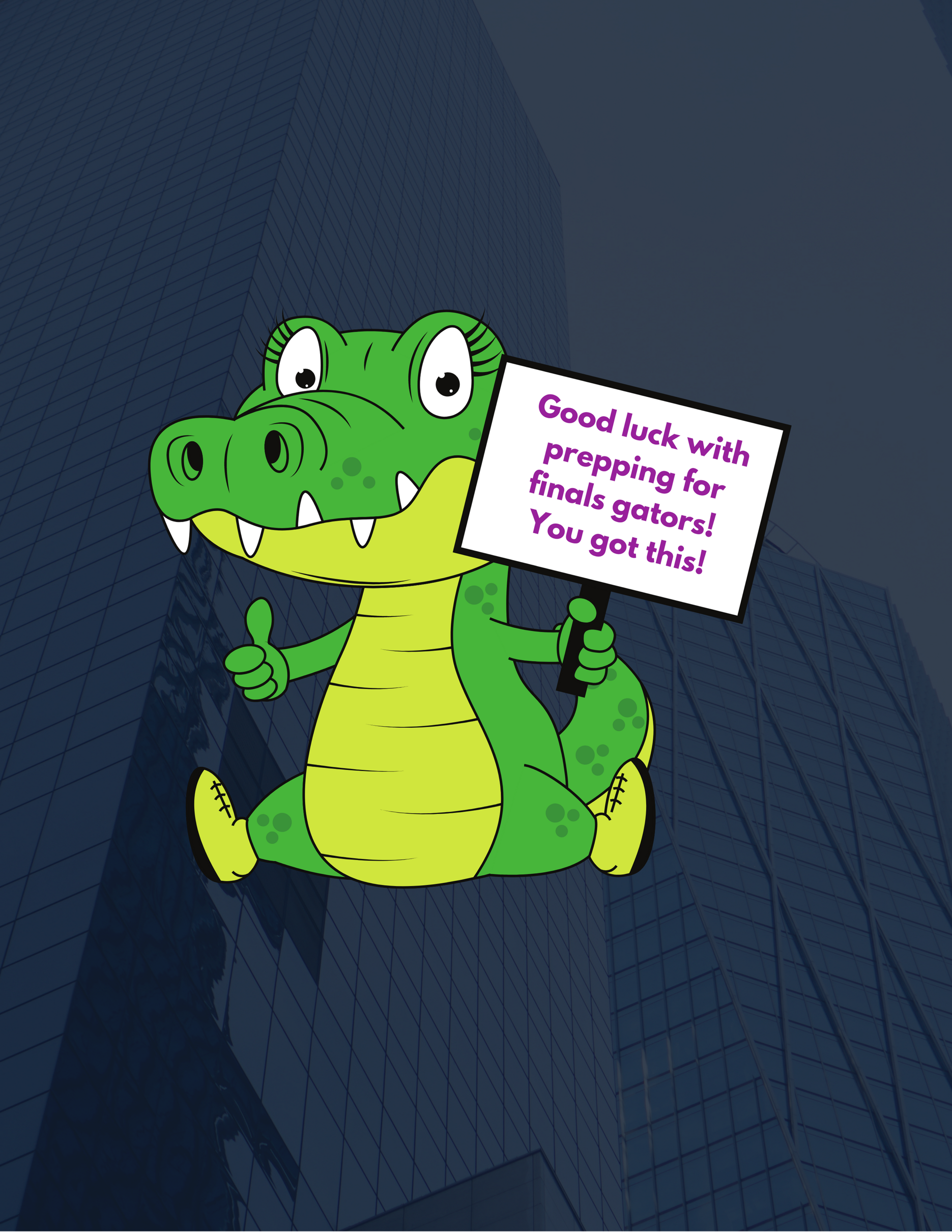
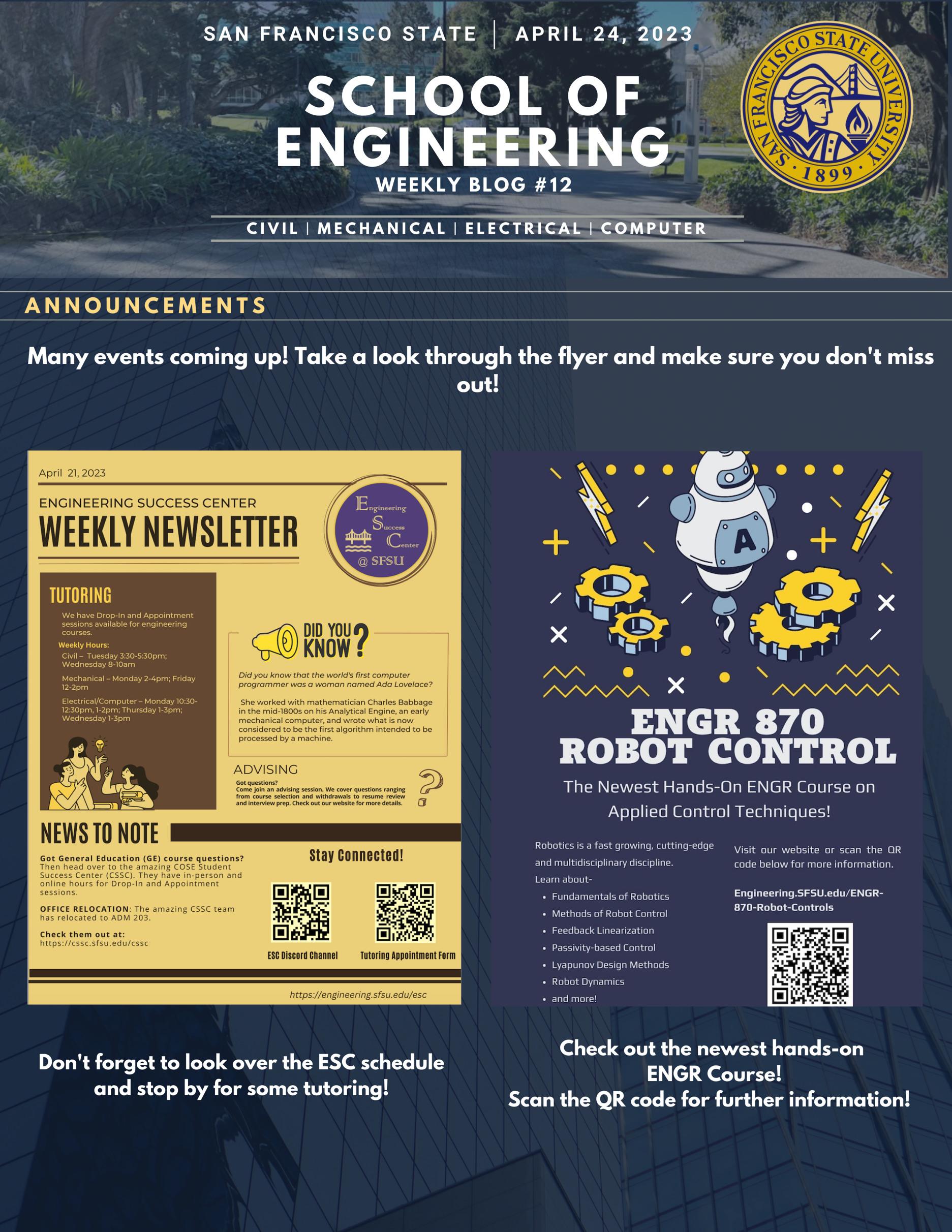
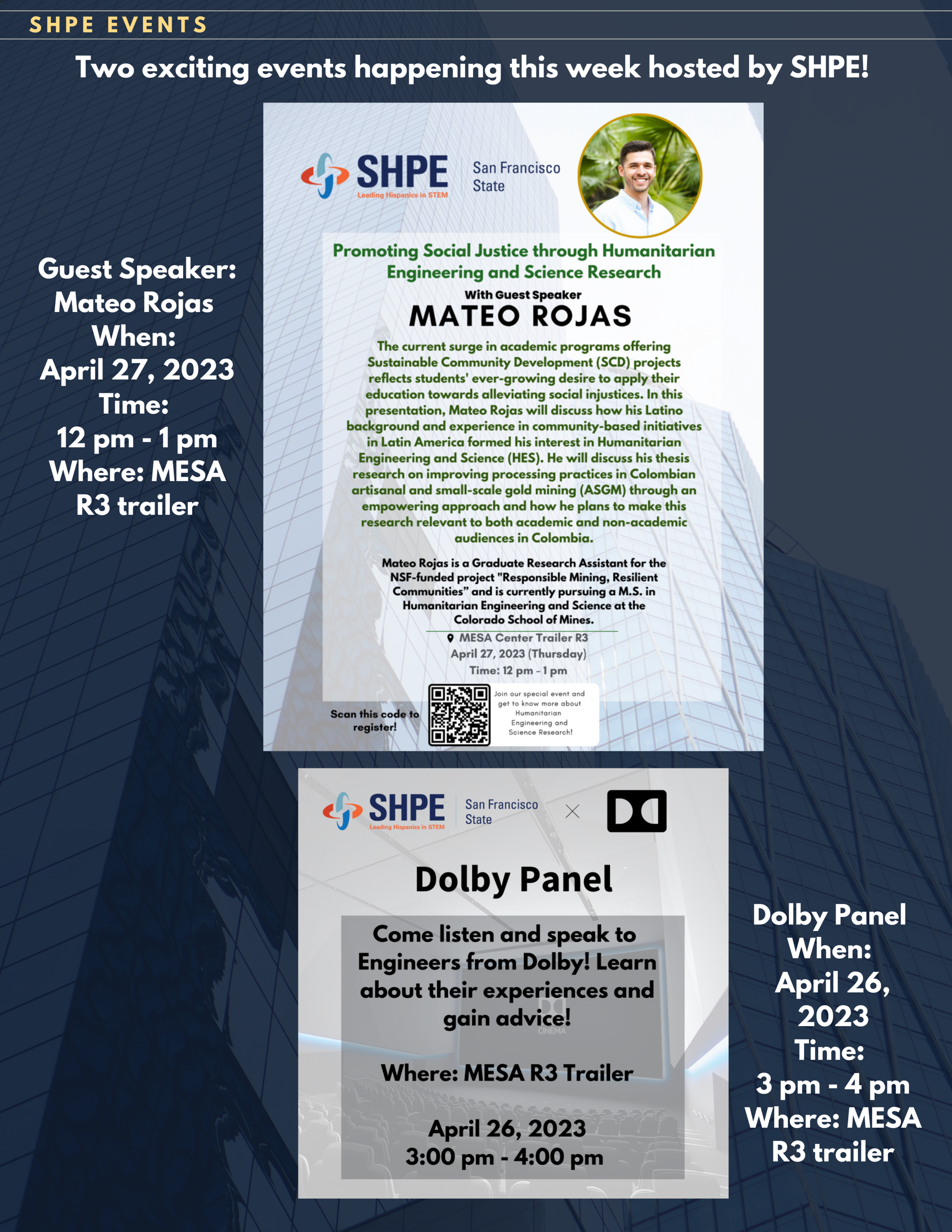
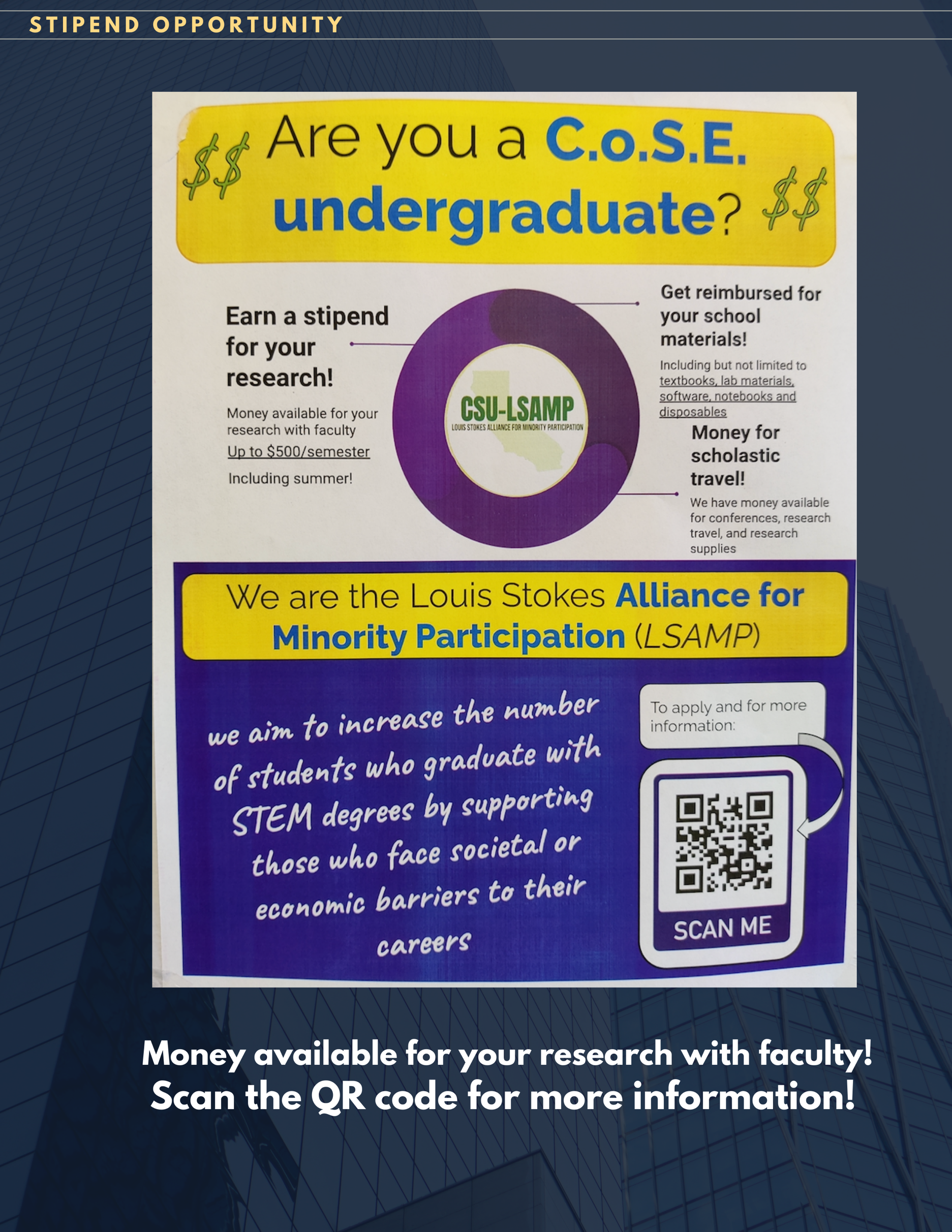
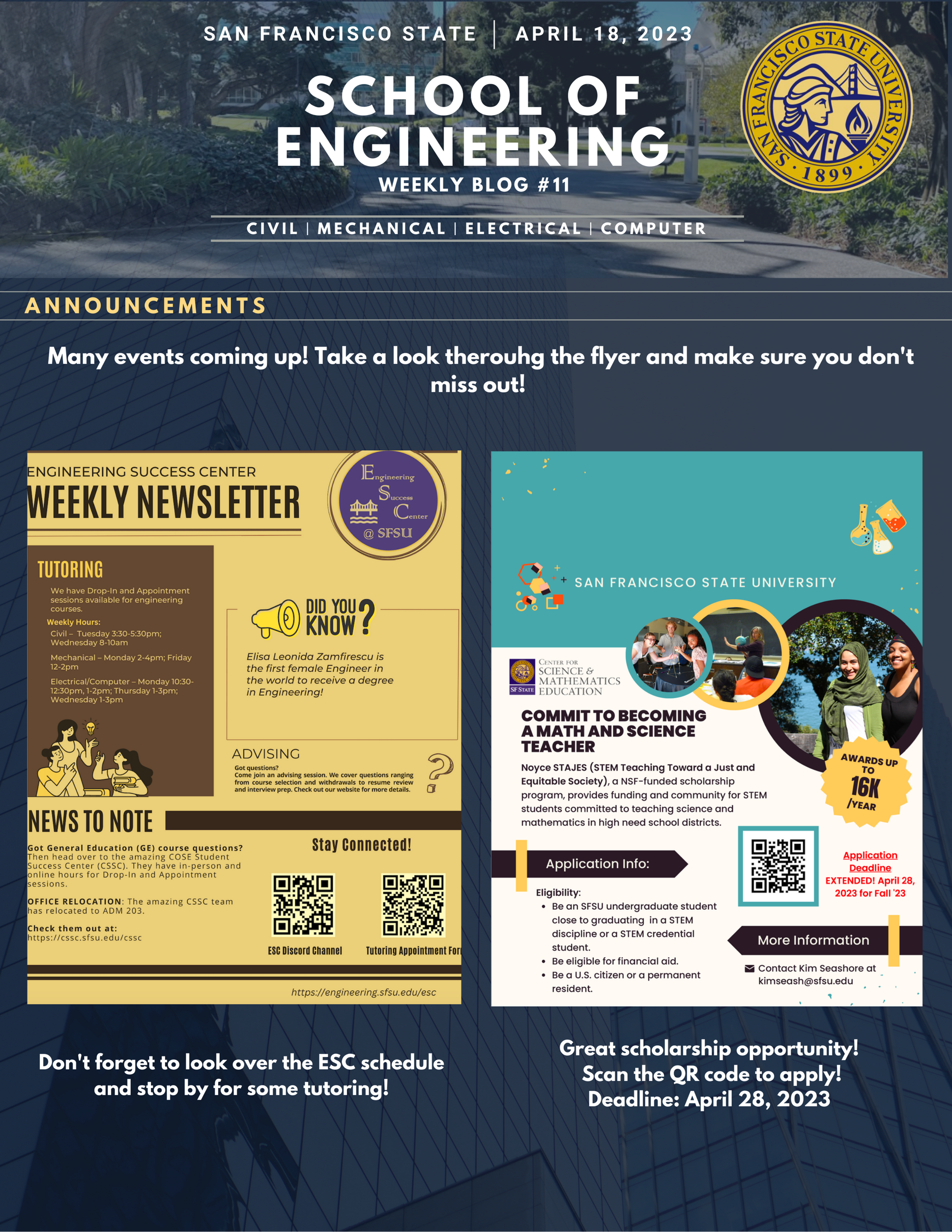
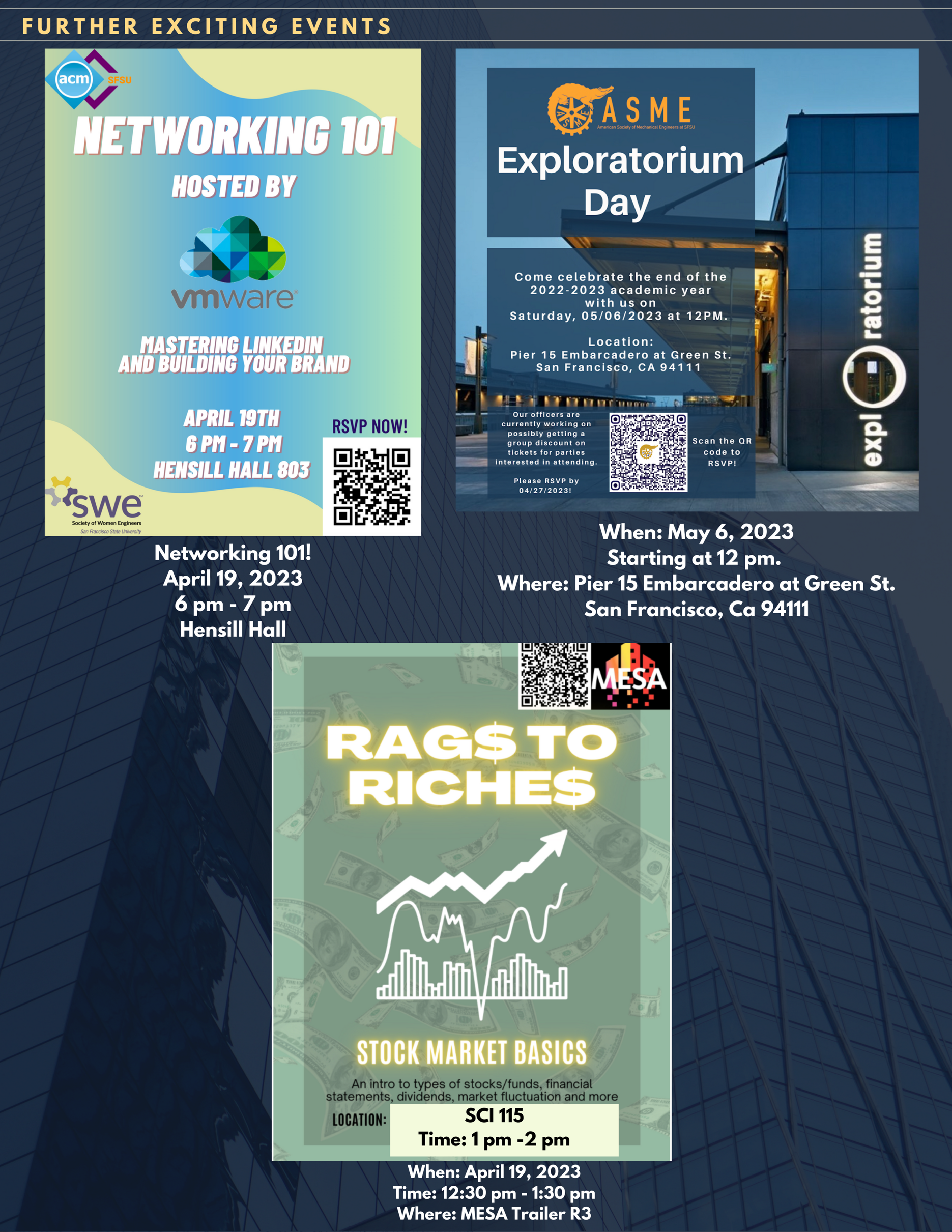
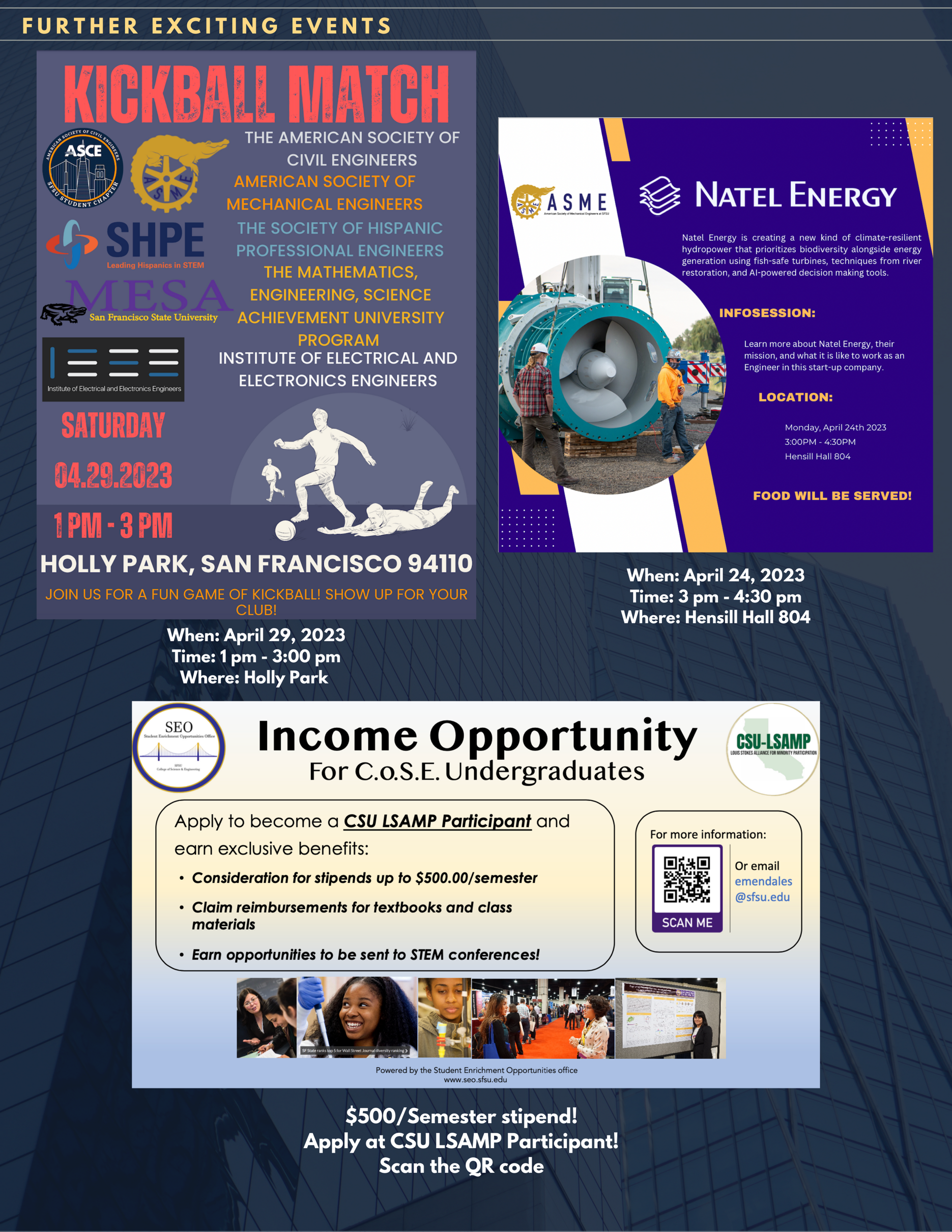
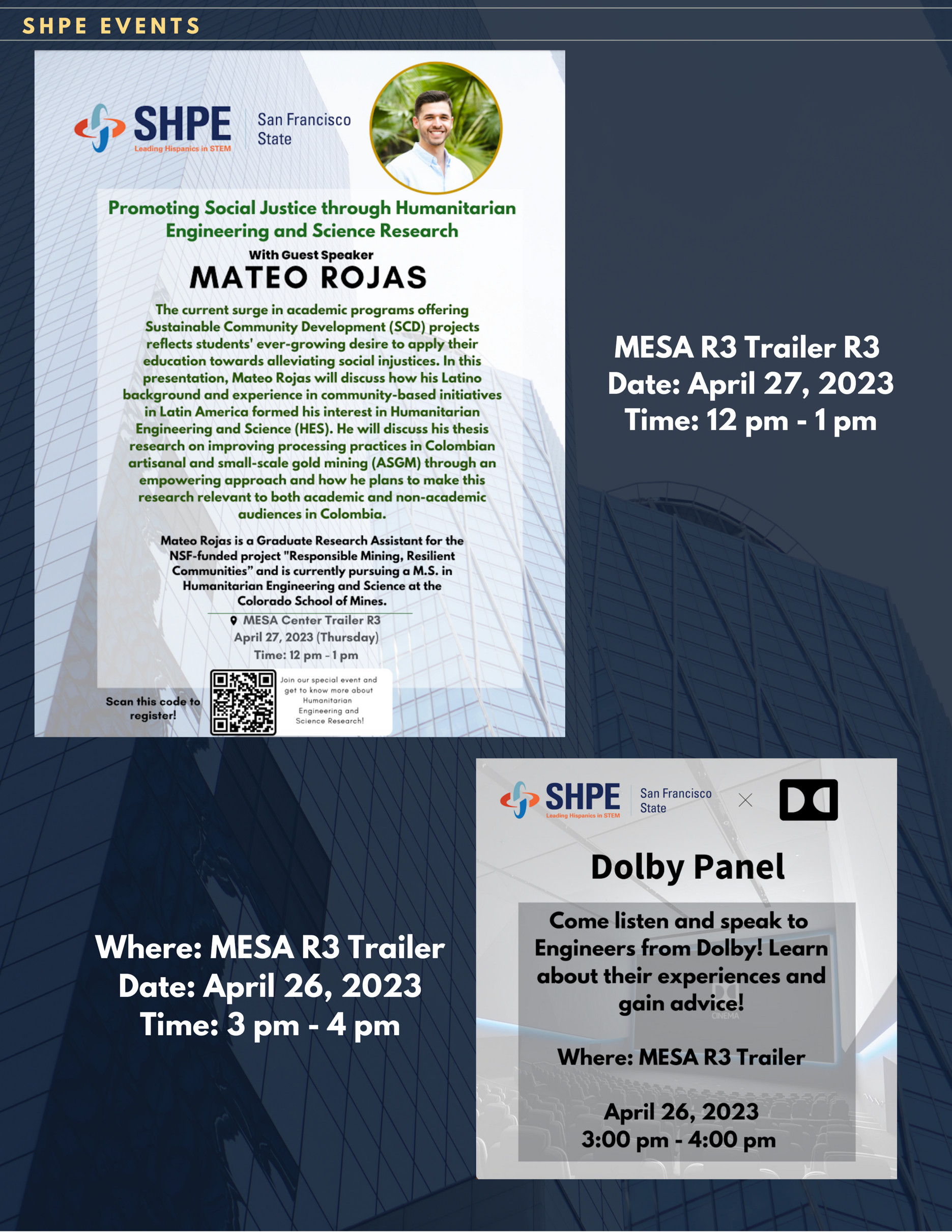
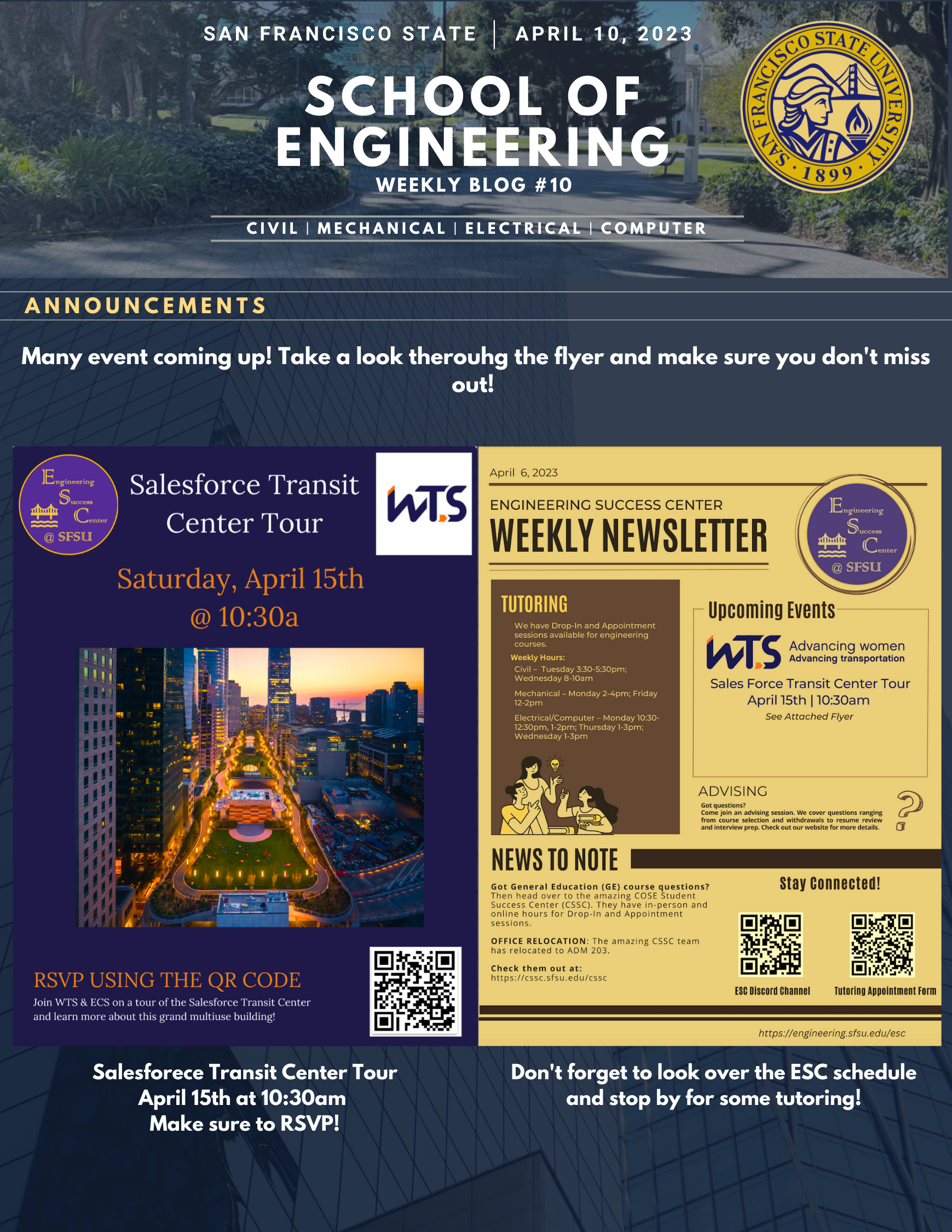
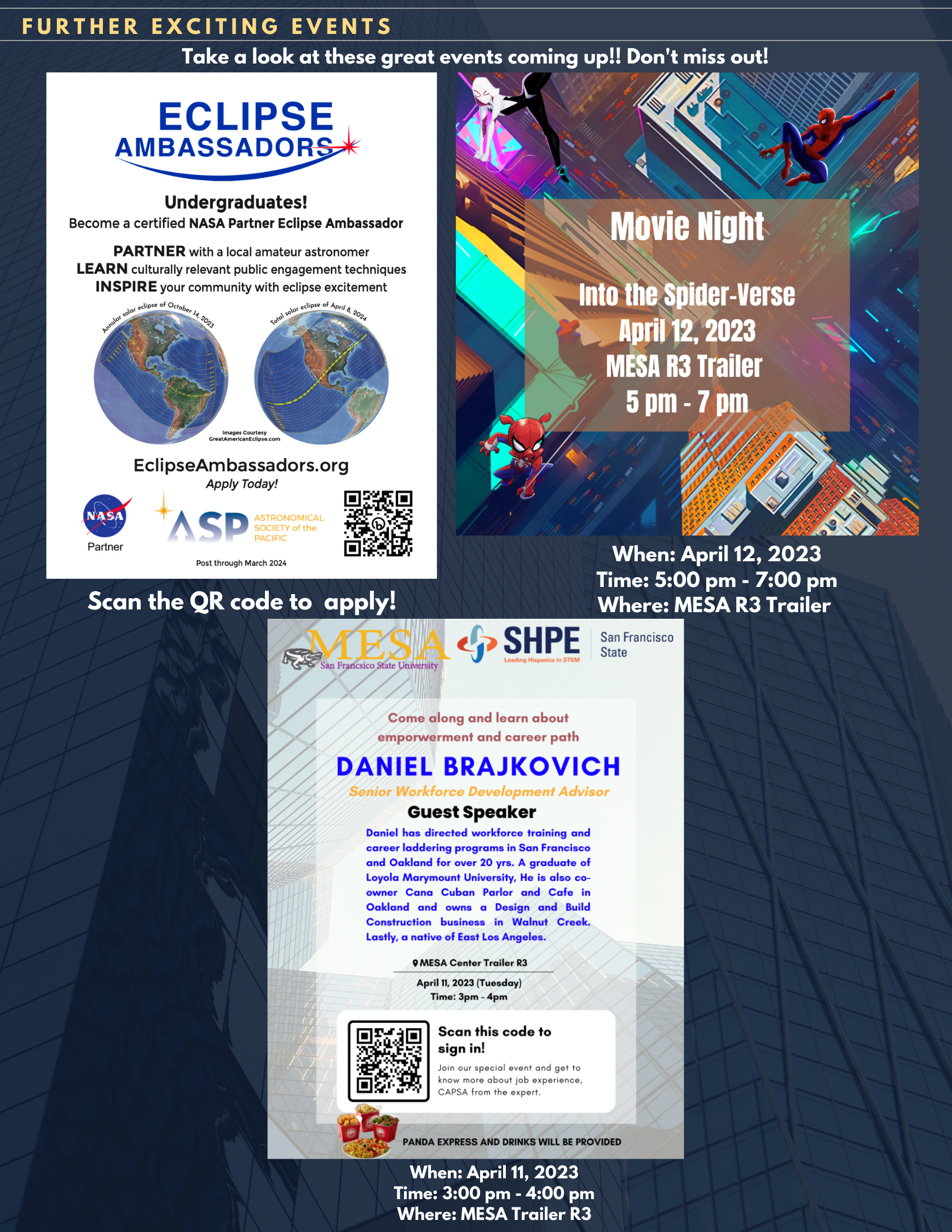
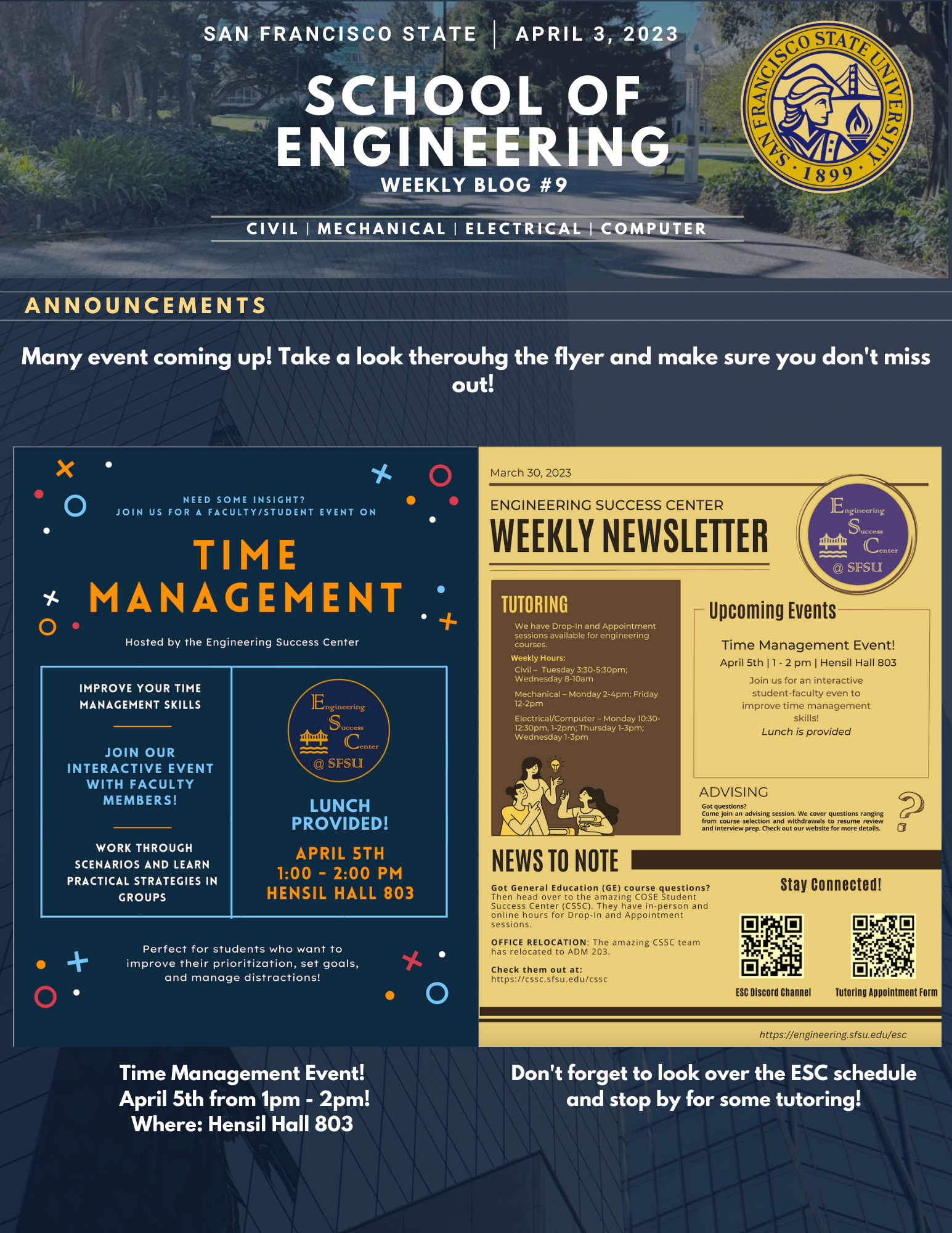
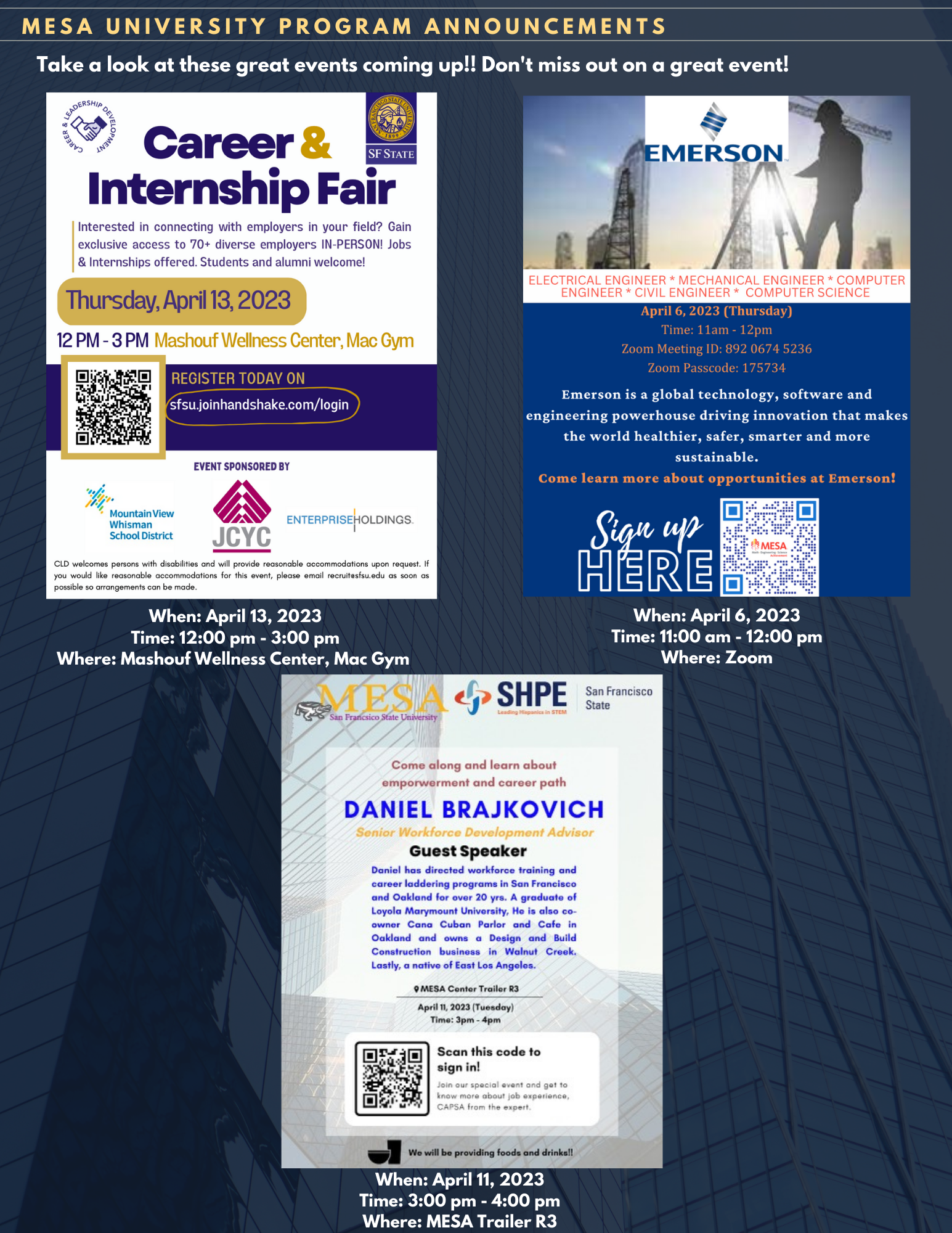
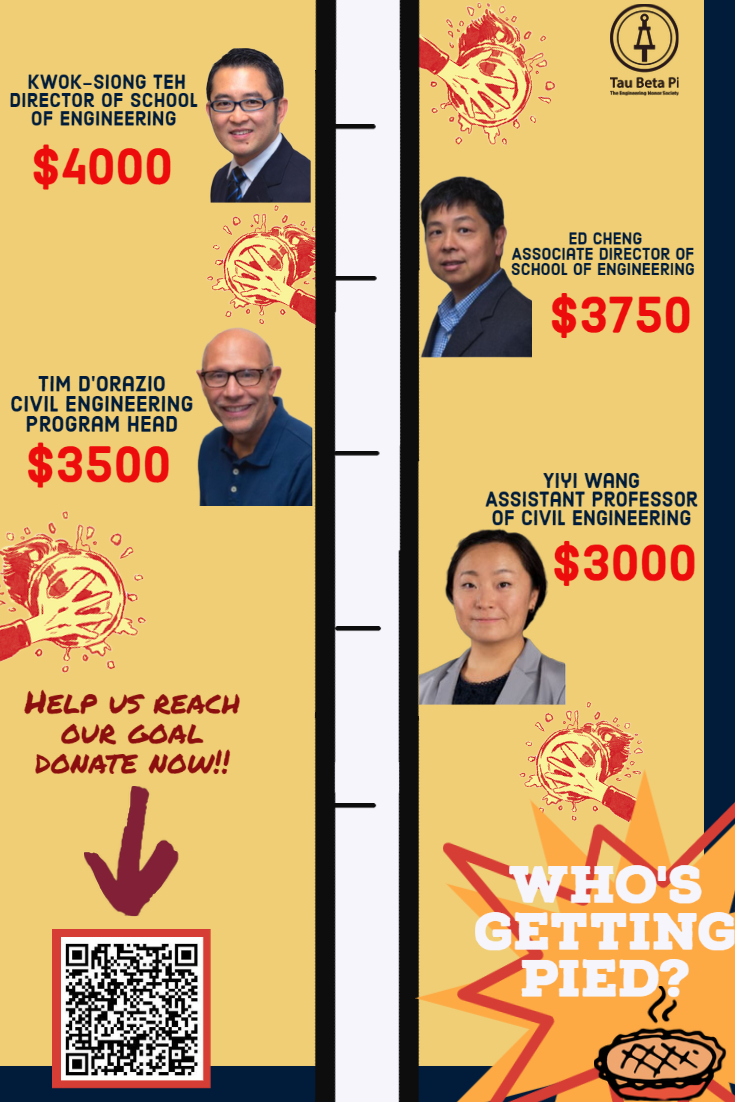
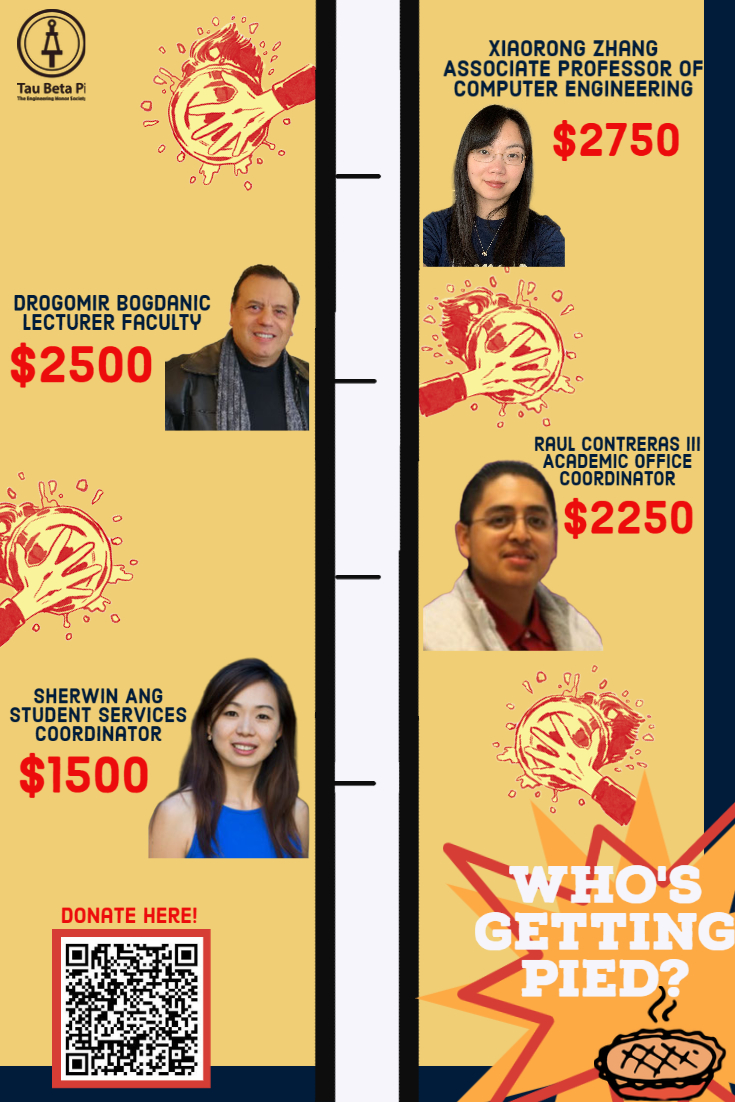
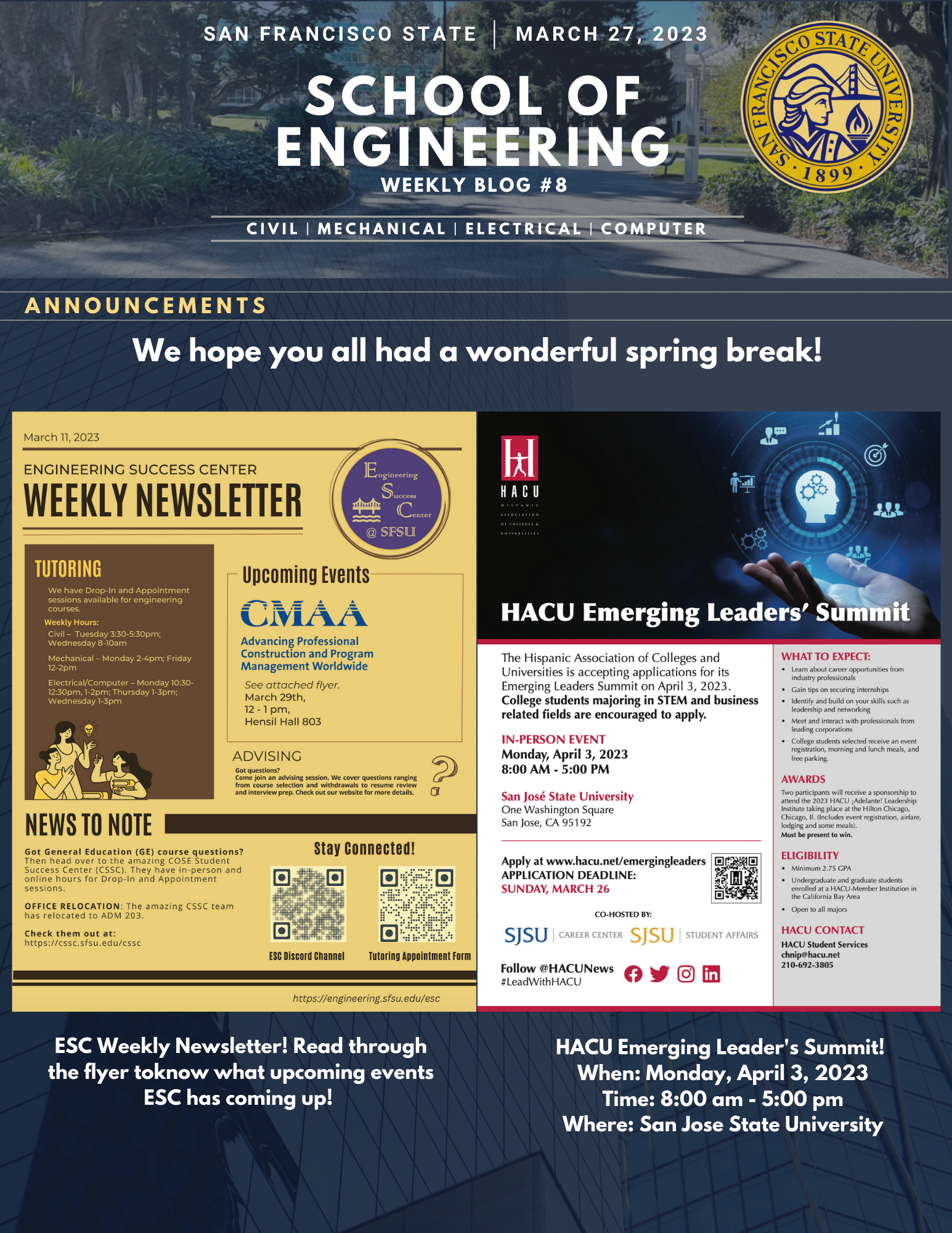
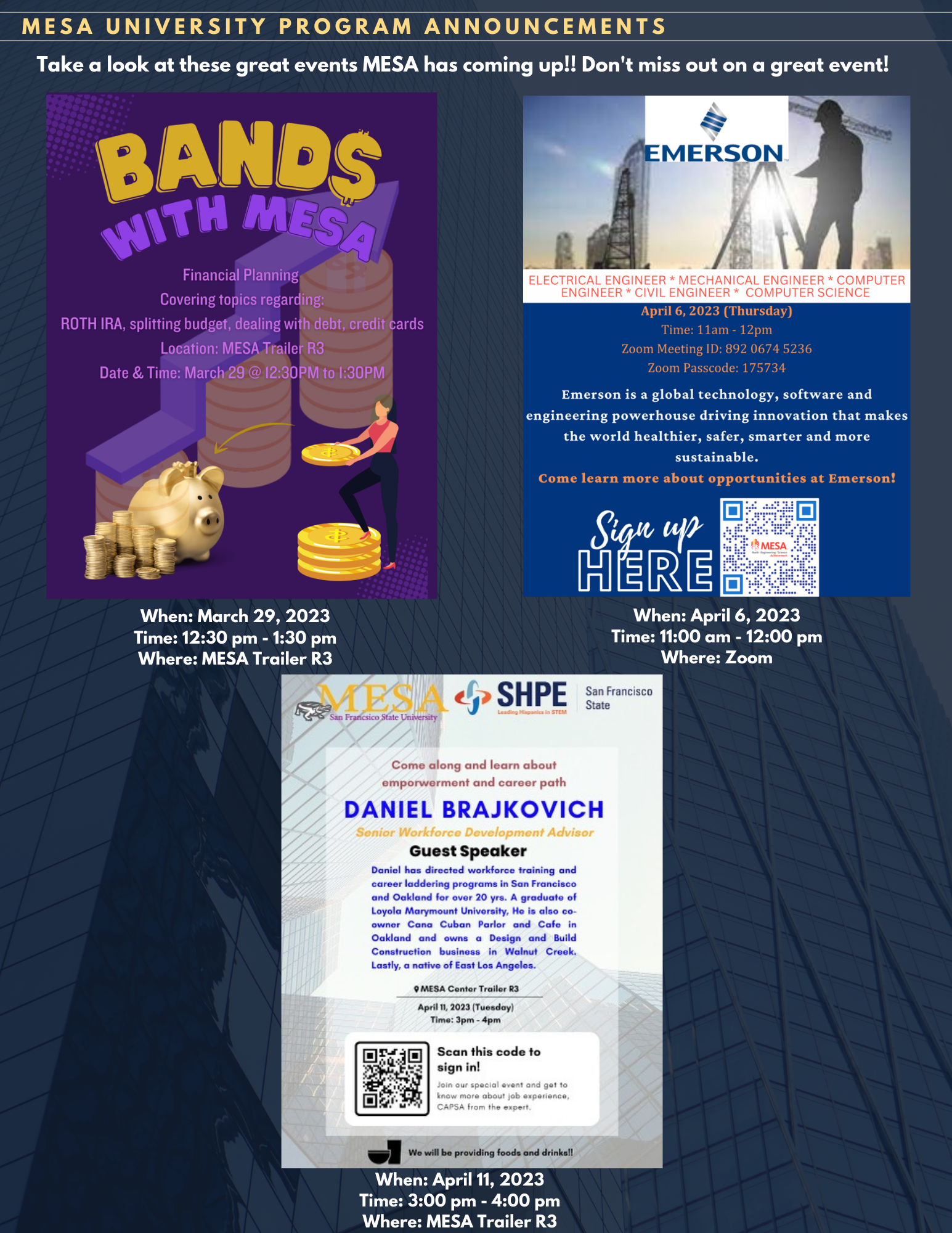
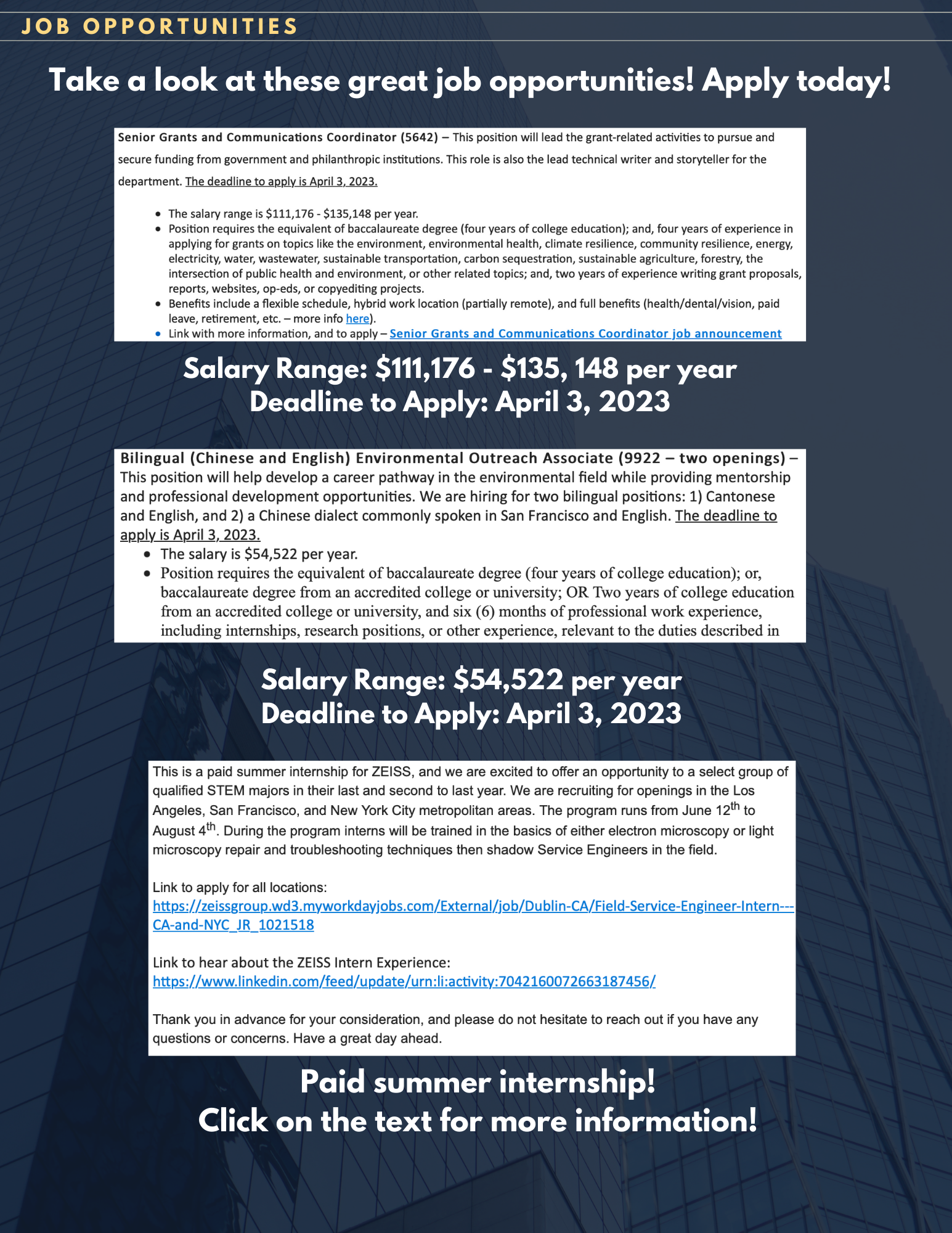
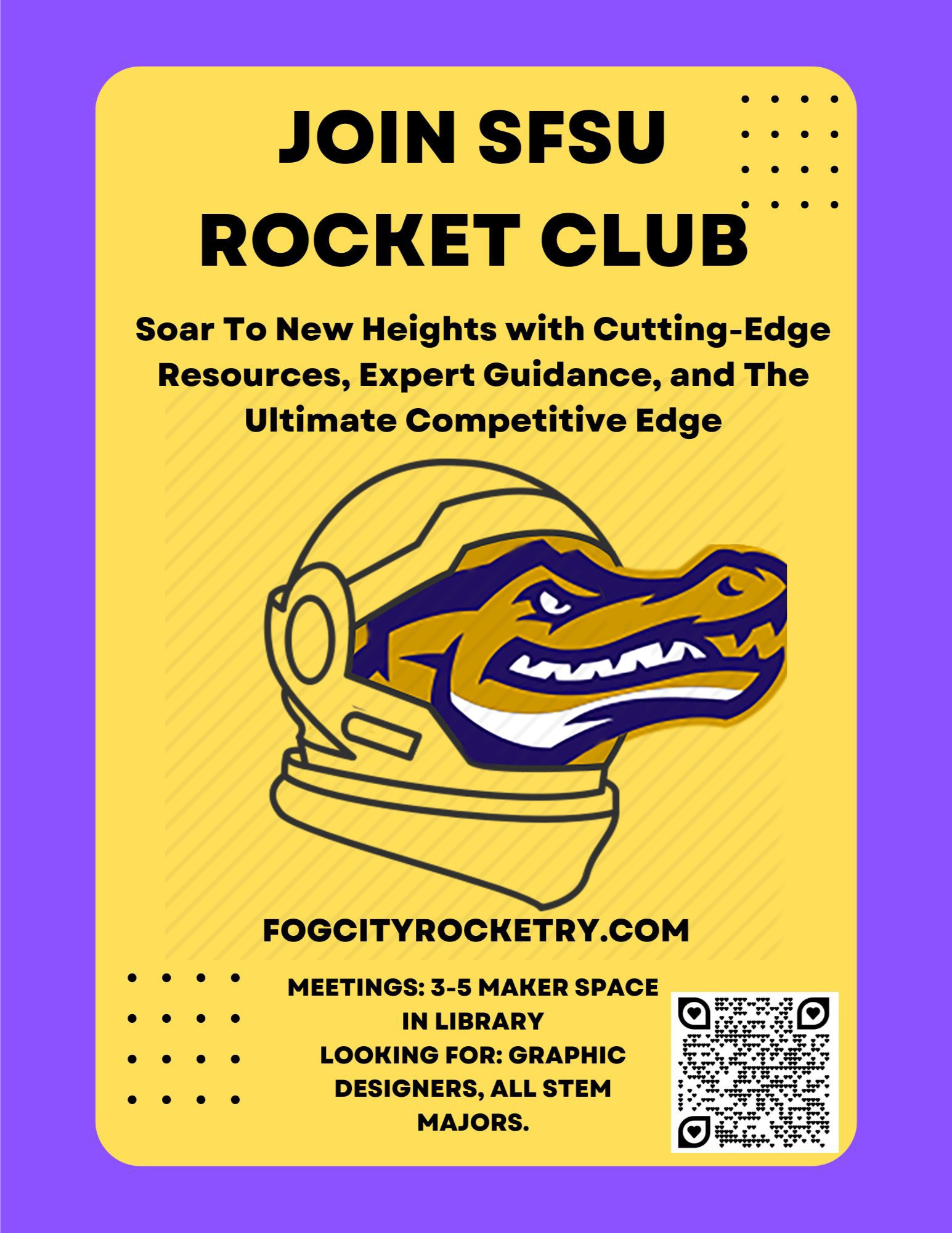
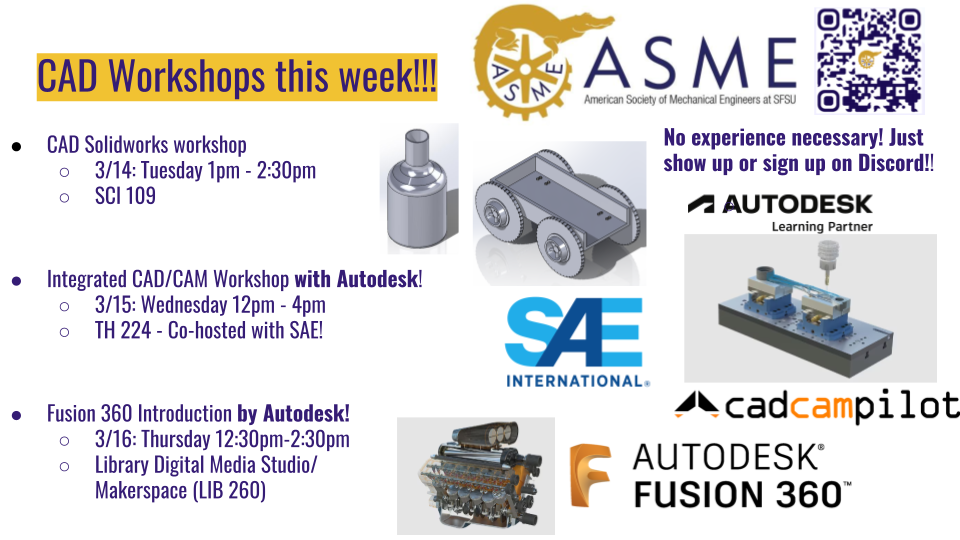
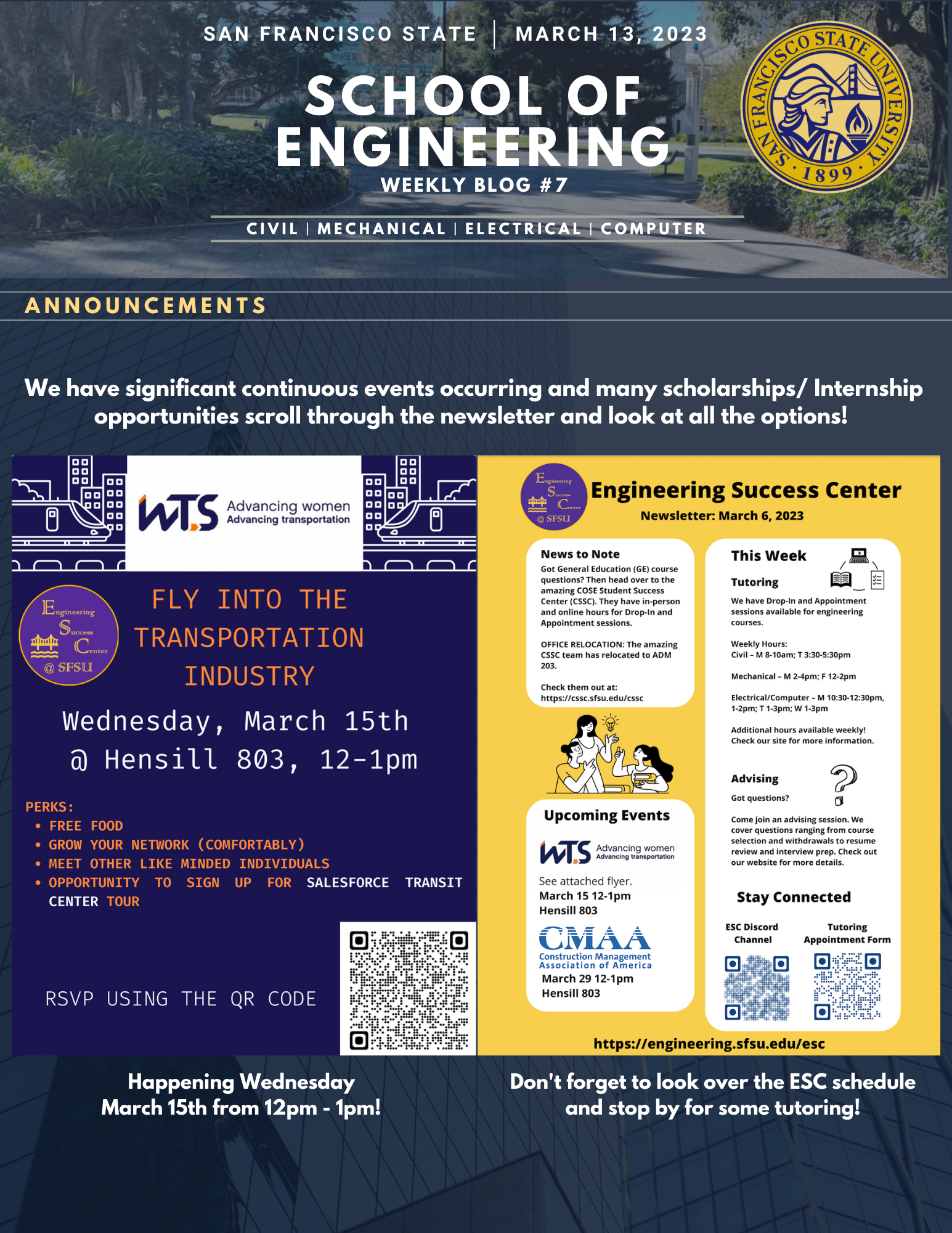
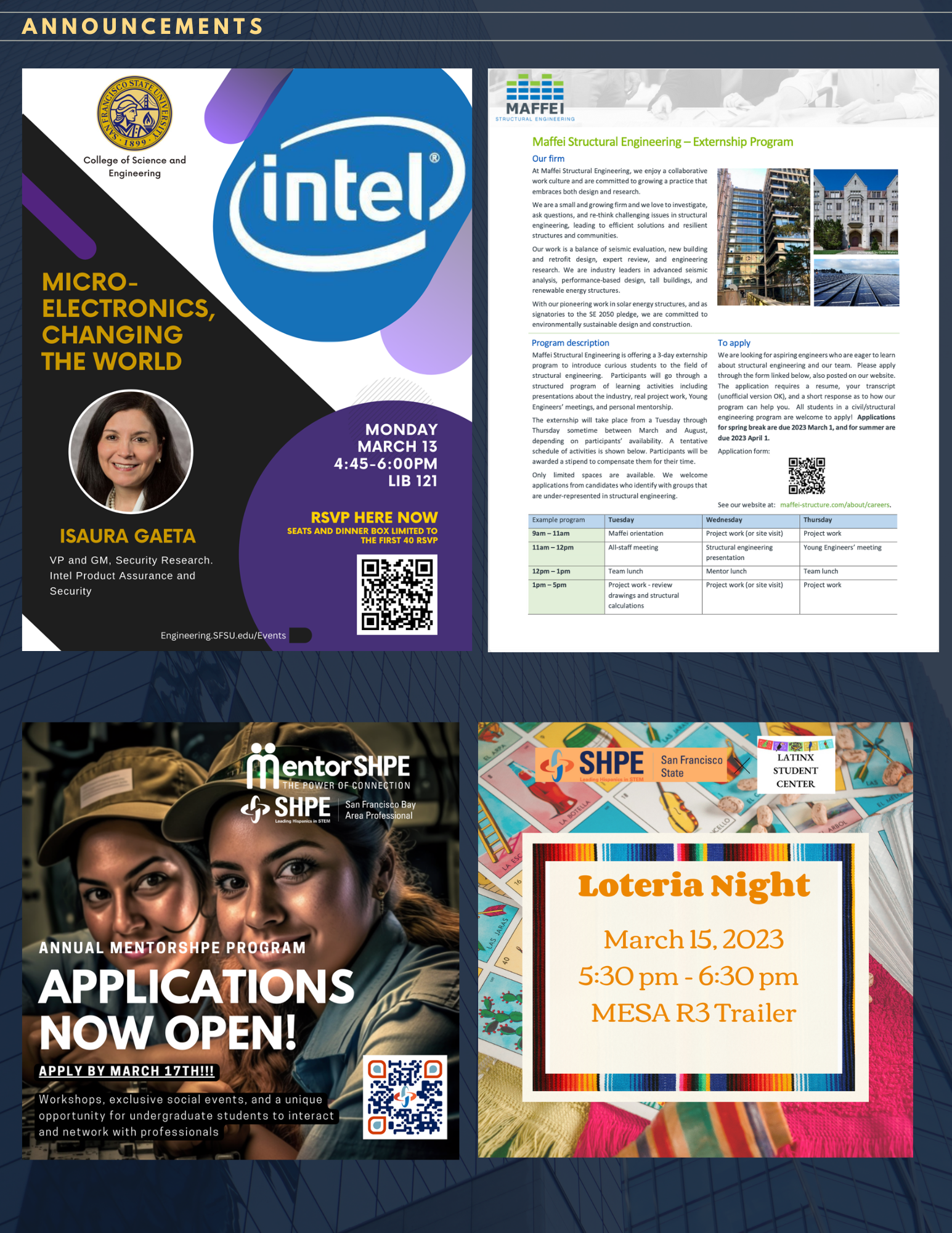
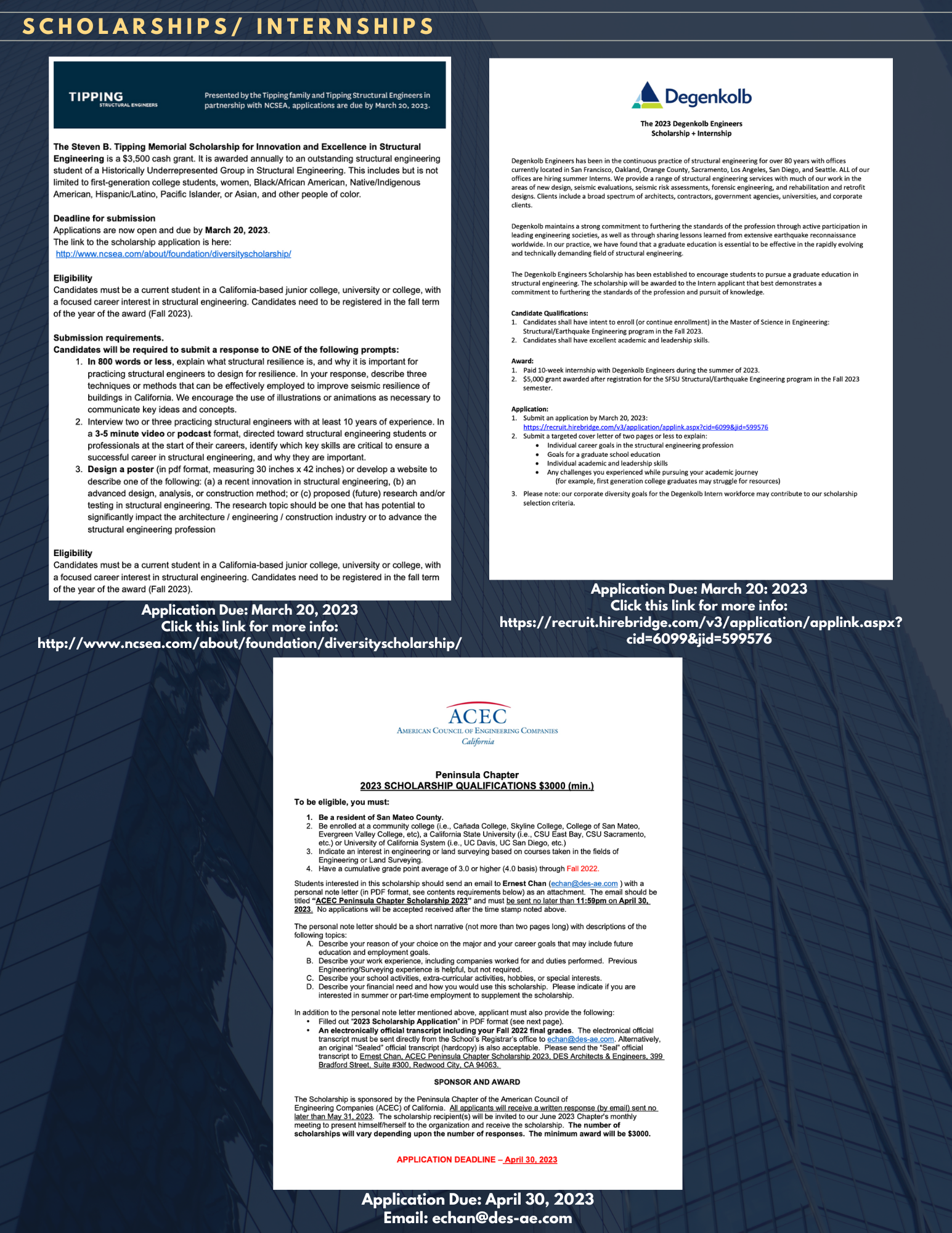
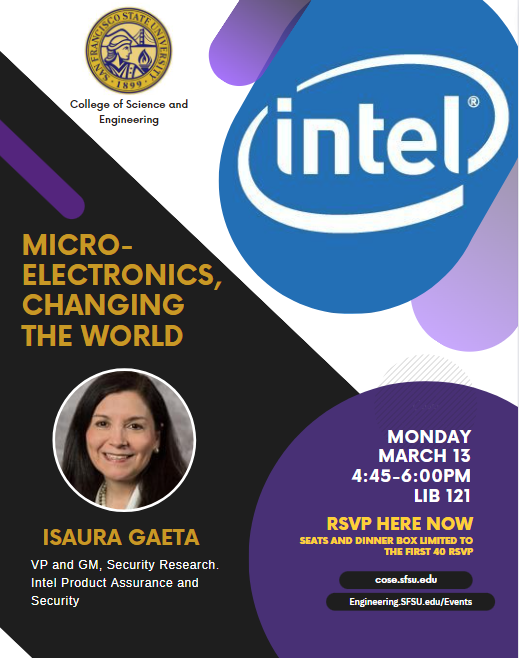
Microelectronics- Changing the World
A talk by Intel VP Isaura Gaeta
Today, March 13, 2023, 4:45-6:00pm at LIB121
Speaker Bio:
Isaura leads an engineering team focused on hardware security research, including penetration testing, physical attack mechanisms, and outreach to academia. Previously she was GM of systems engineering in the Platform Engineering Group and was responsible for optimizing engineering execution capacity, quality management systems, and operational excellence for a global engineering organization of 18k people. A 30-year veteran of Intel, Gaeta spent the first two decades of her Intel career developing various semiconductor processing technologies. Her work during that period led to two patents and five Intel Achievement Awards, the company’s highest recognition. Gaeta holds bachelor’s and master’s degrees in electrical engineering from Stanford University. She founded Intel’s Network of Executive Women for the Latin America region and was the chair of Intel’s Hispanic Leadership Council. Outside of Intel, she serves on the Hispanic Foundation of Silicon Valley board. The Hispanic IT Executive Council has recognized her six times among the top 100 Hispanic professionals in the IT industry.
RSVP to Attend. Seats and Dinner box are limited to the first 40 attendees.
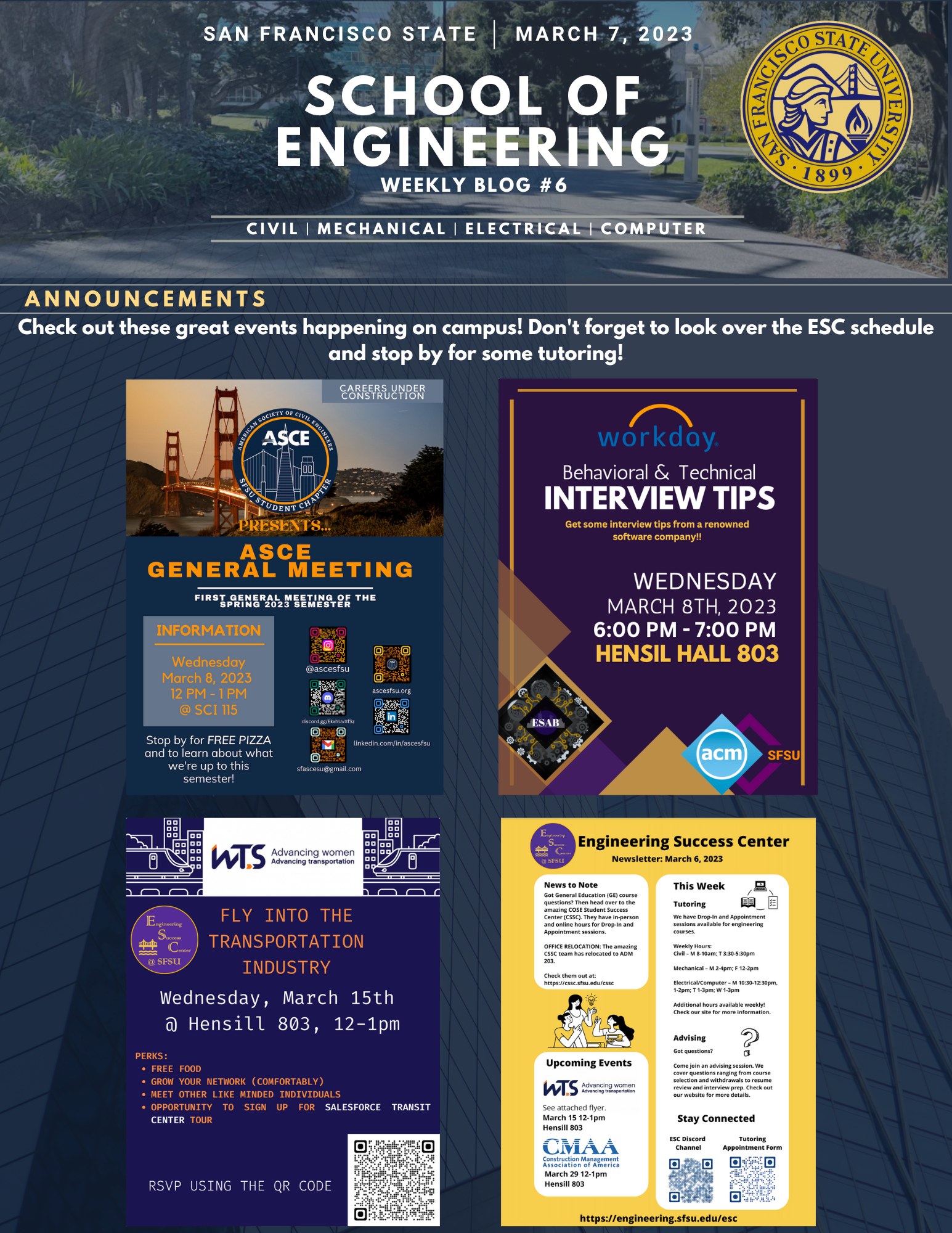
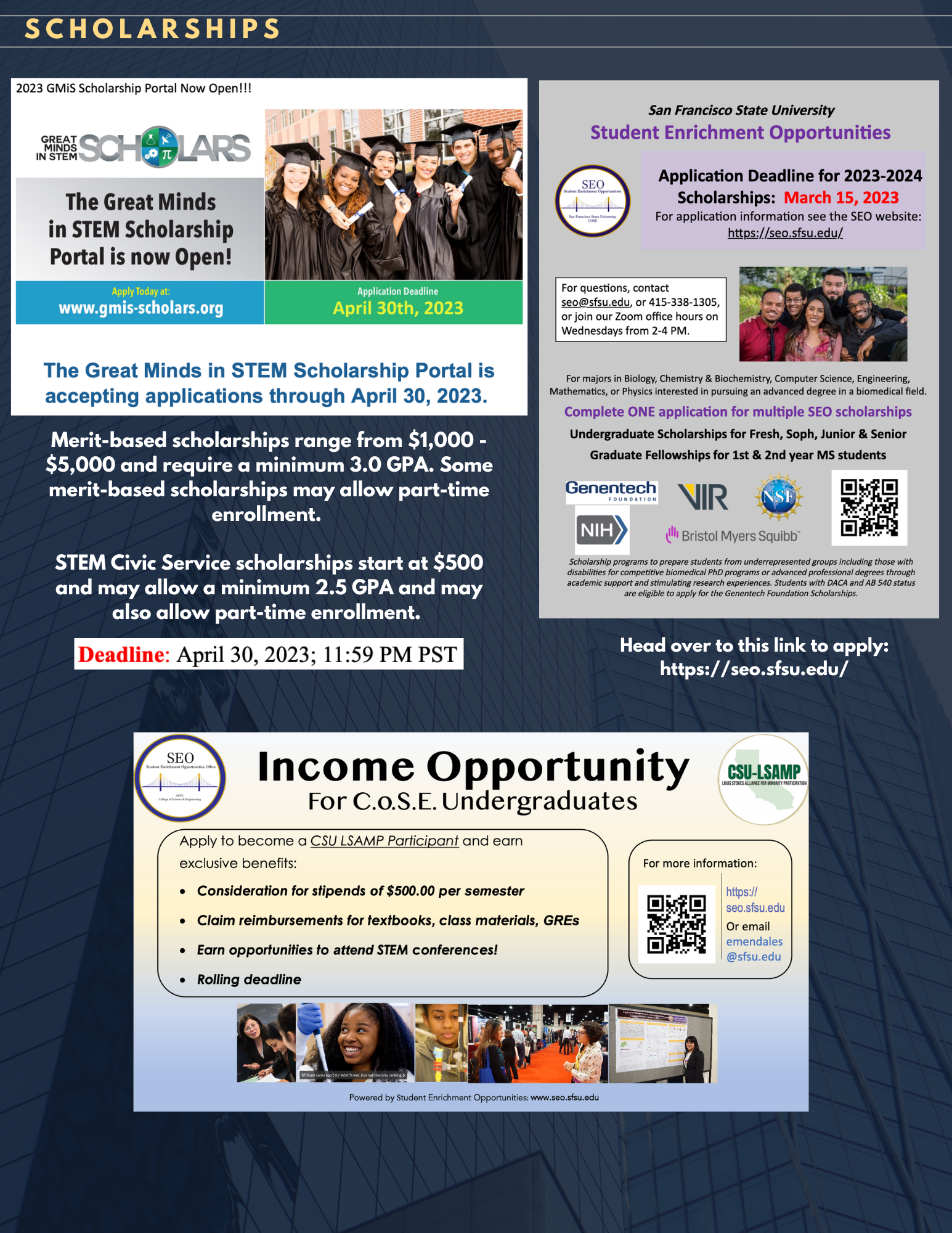
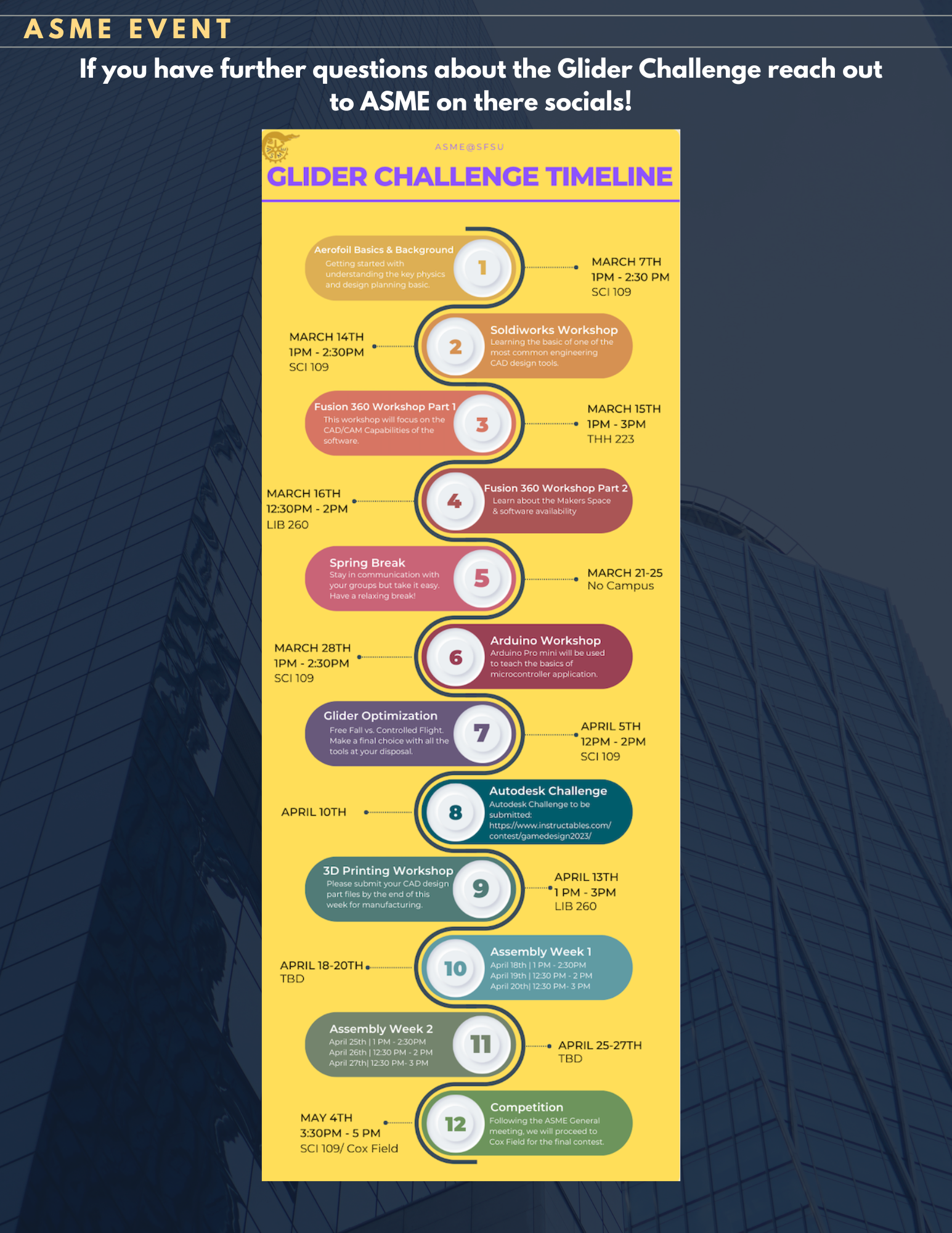
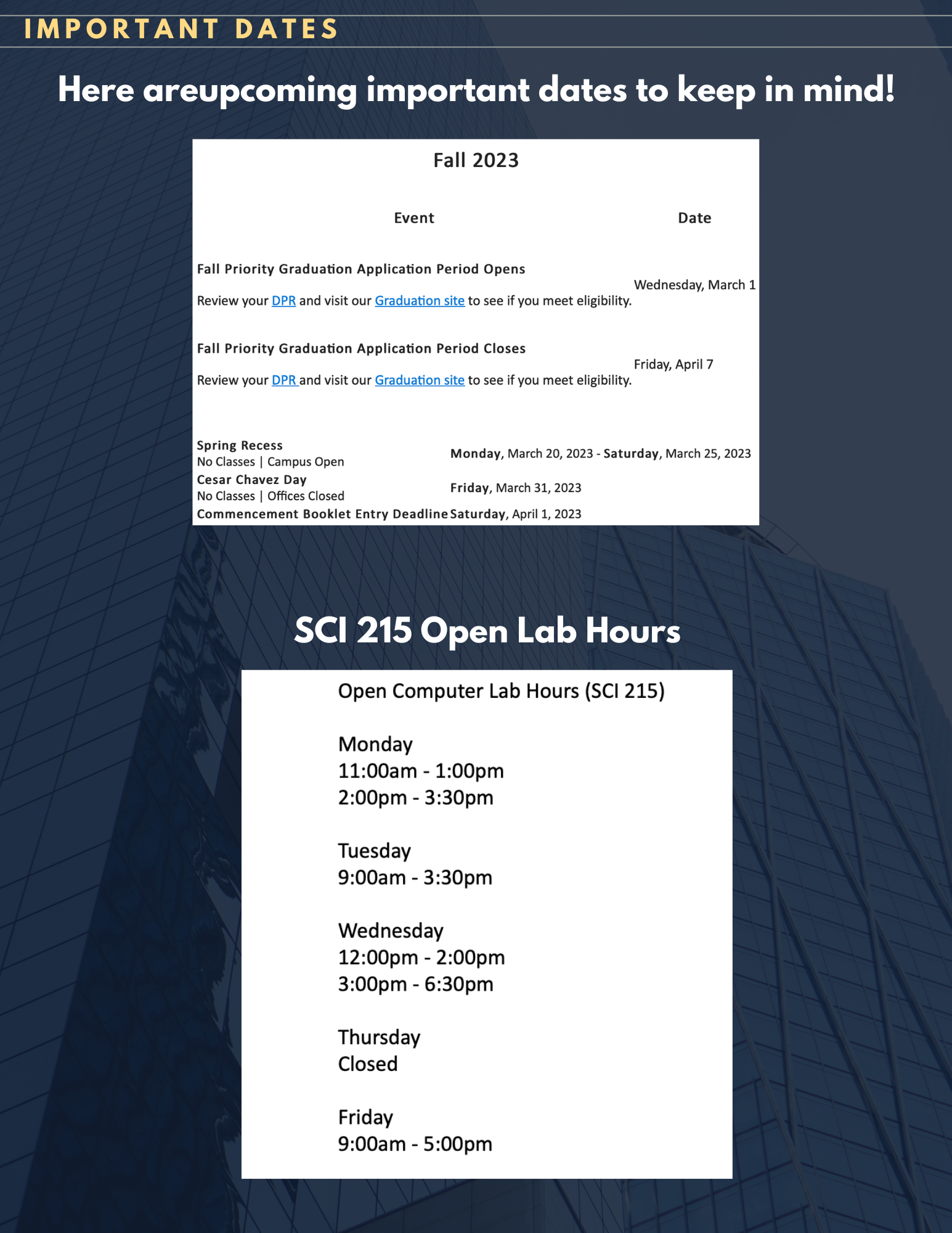
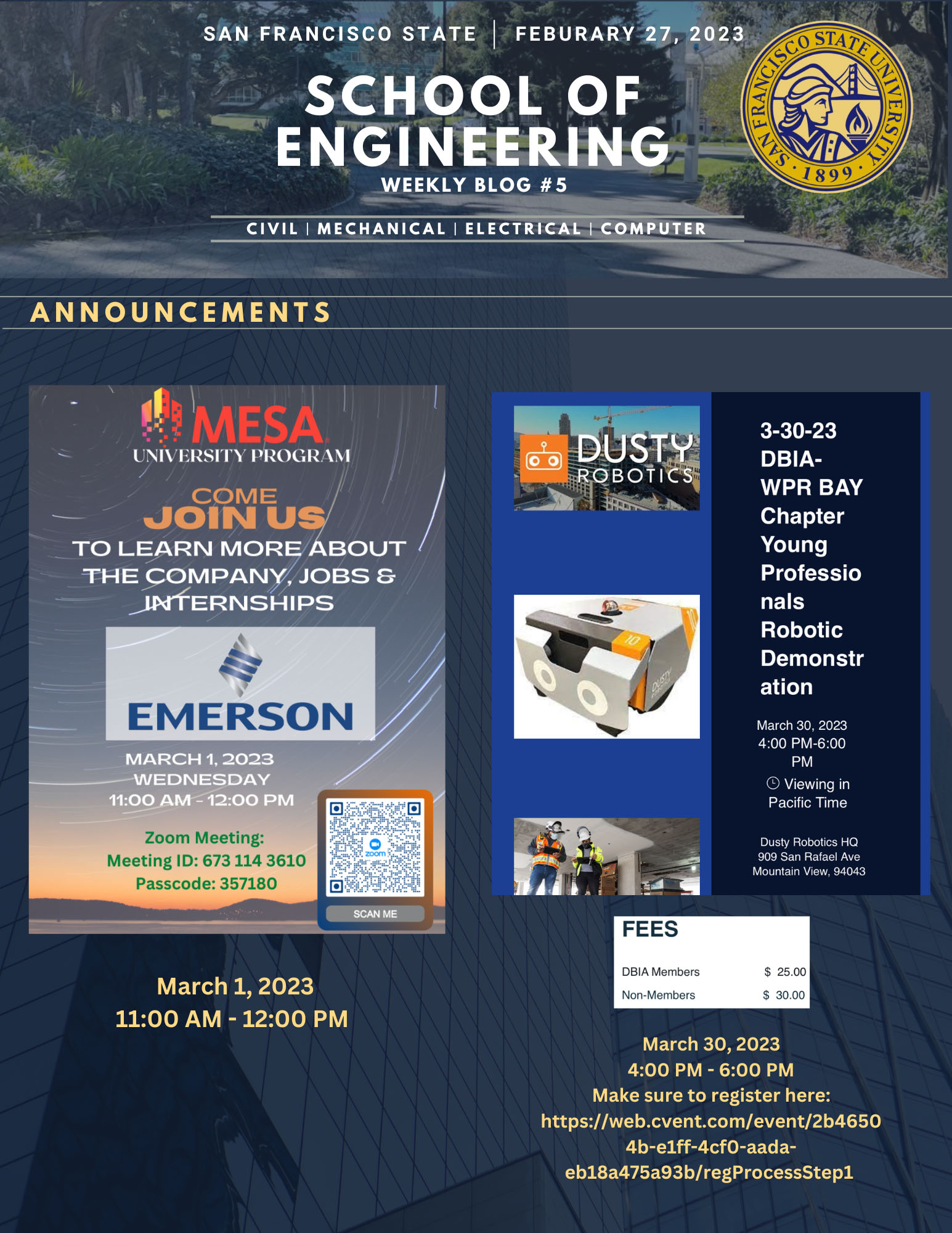
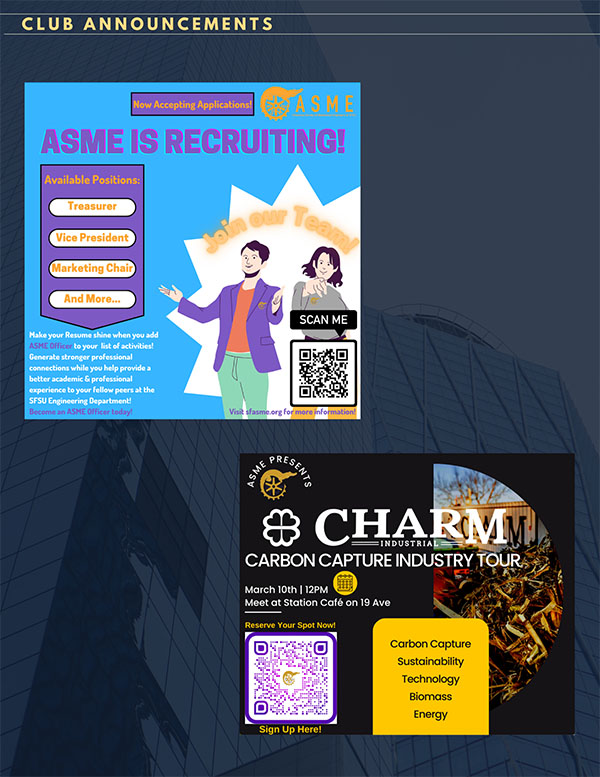
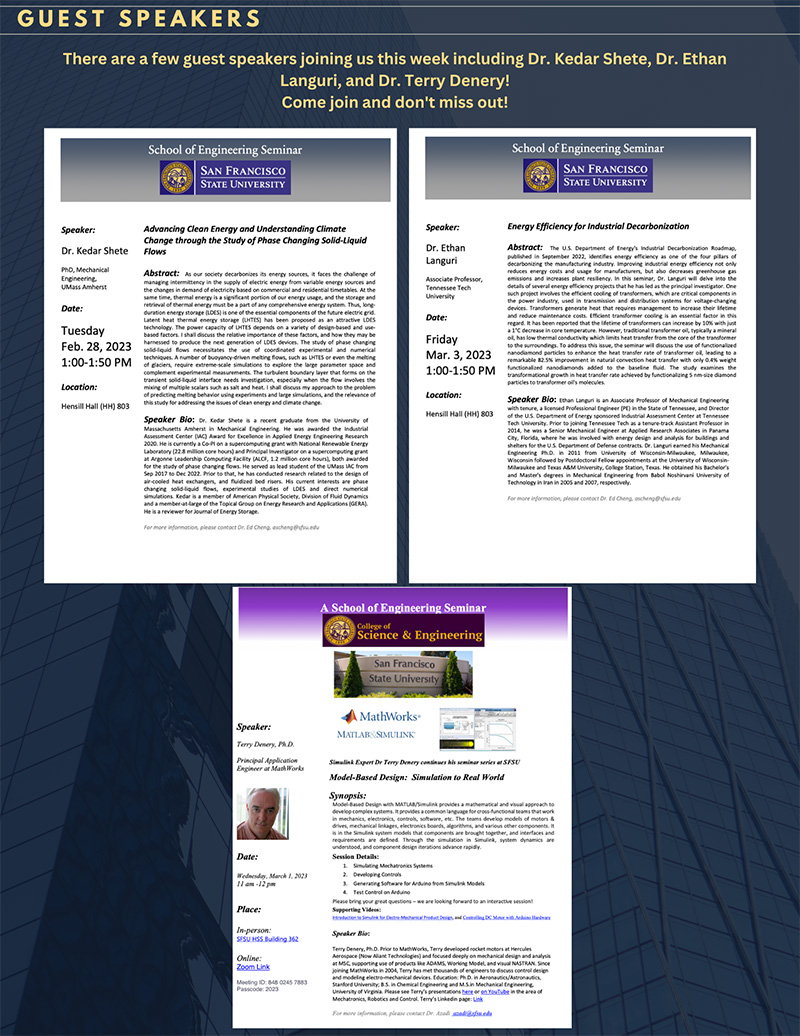
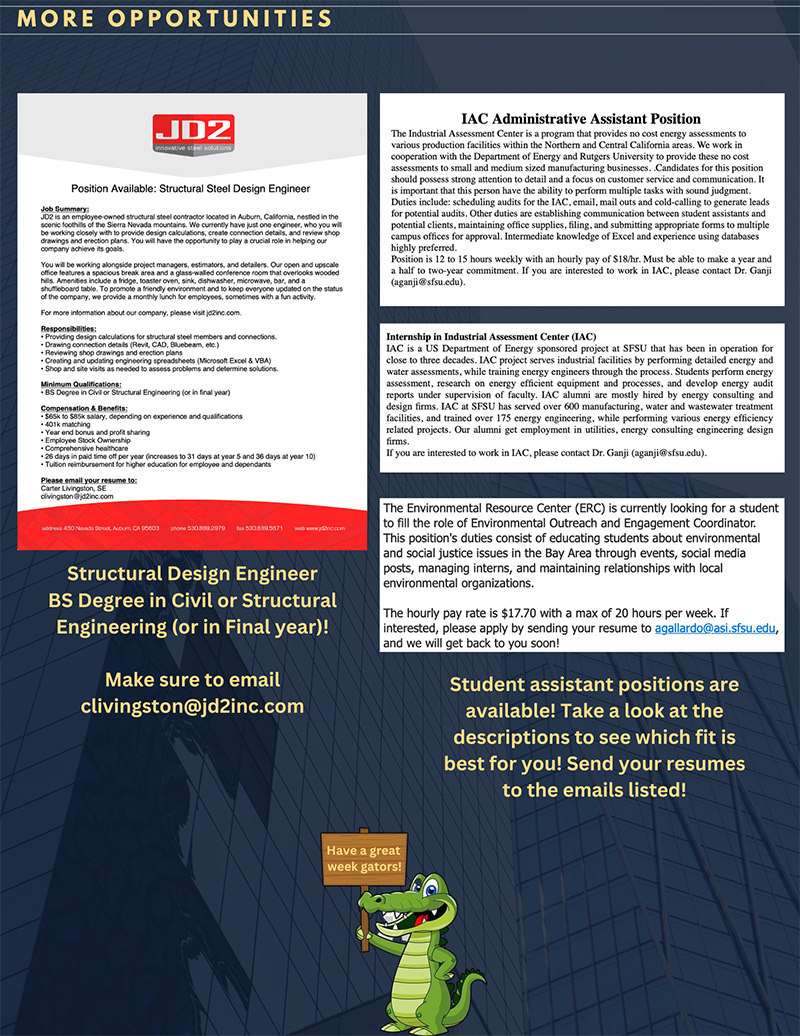
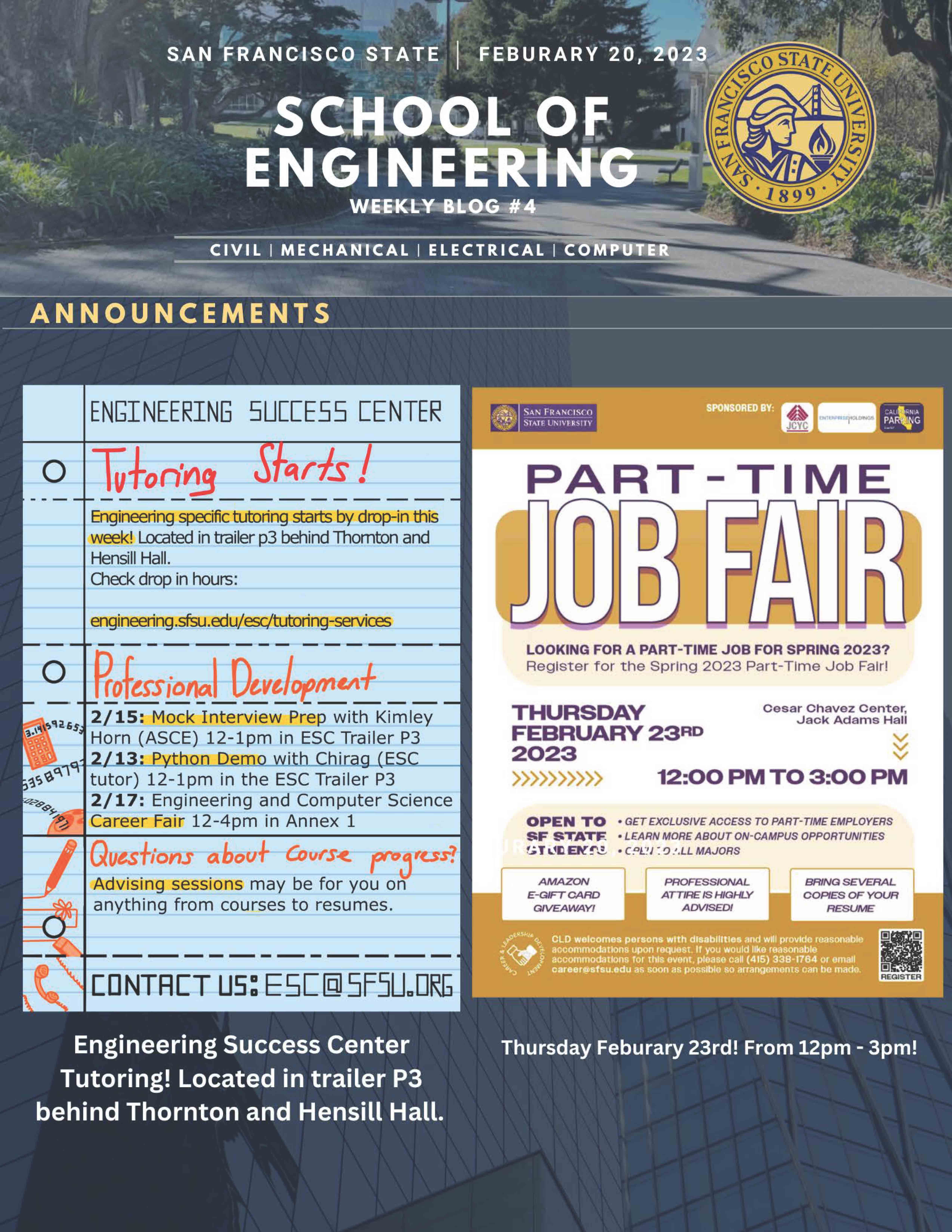
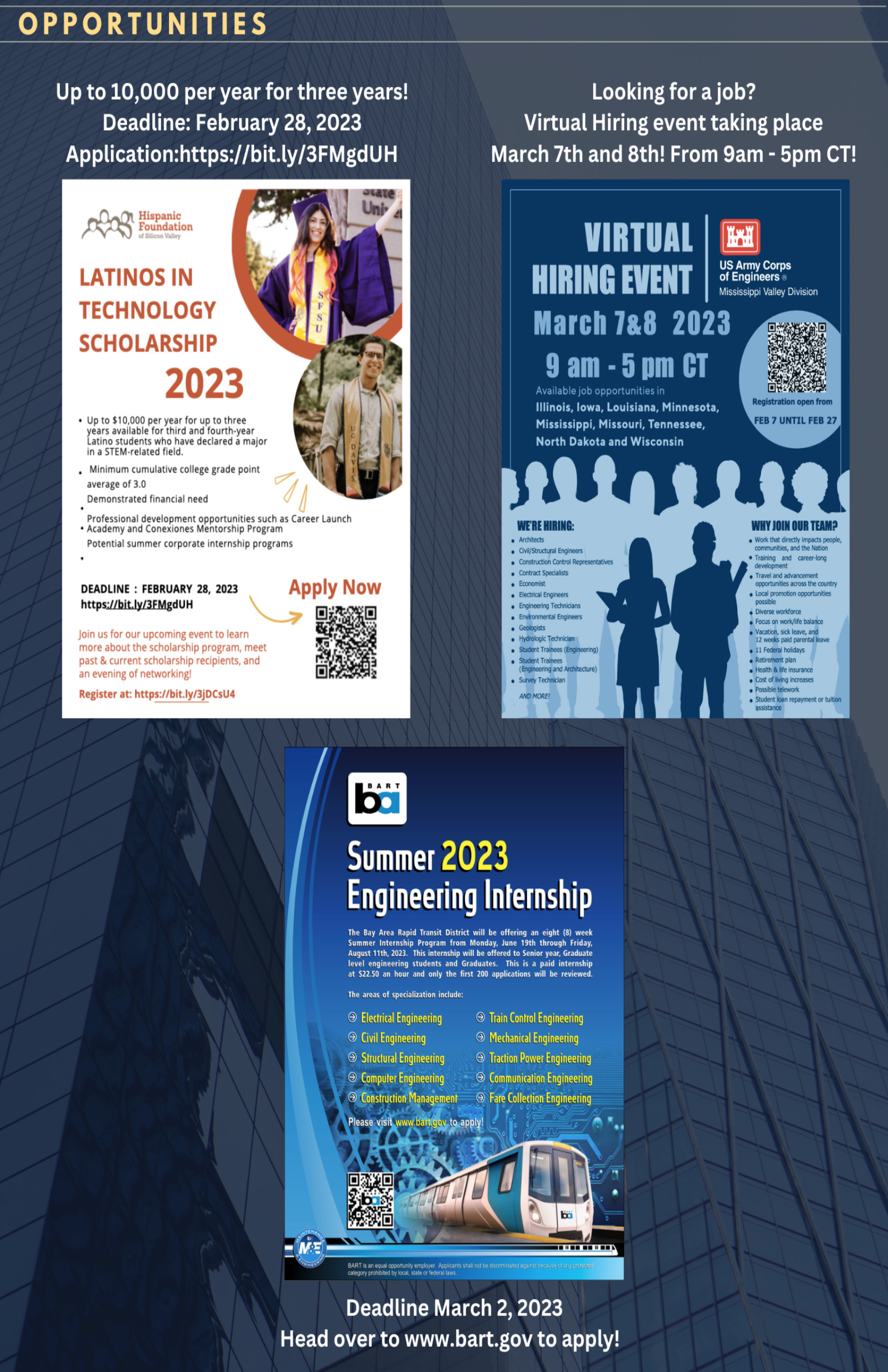
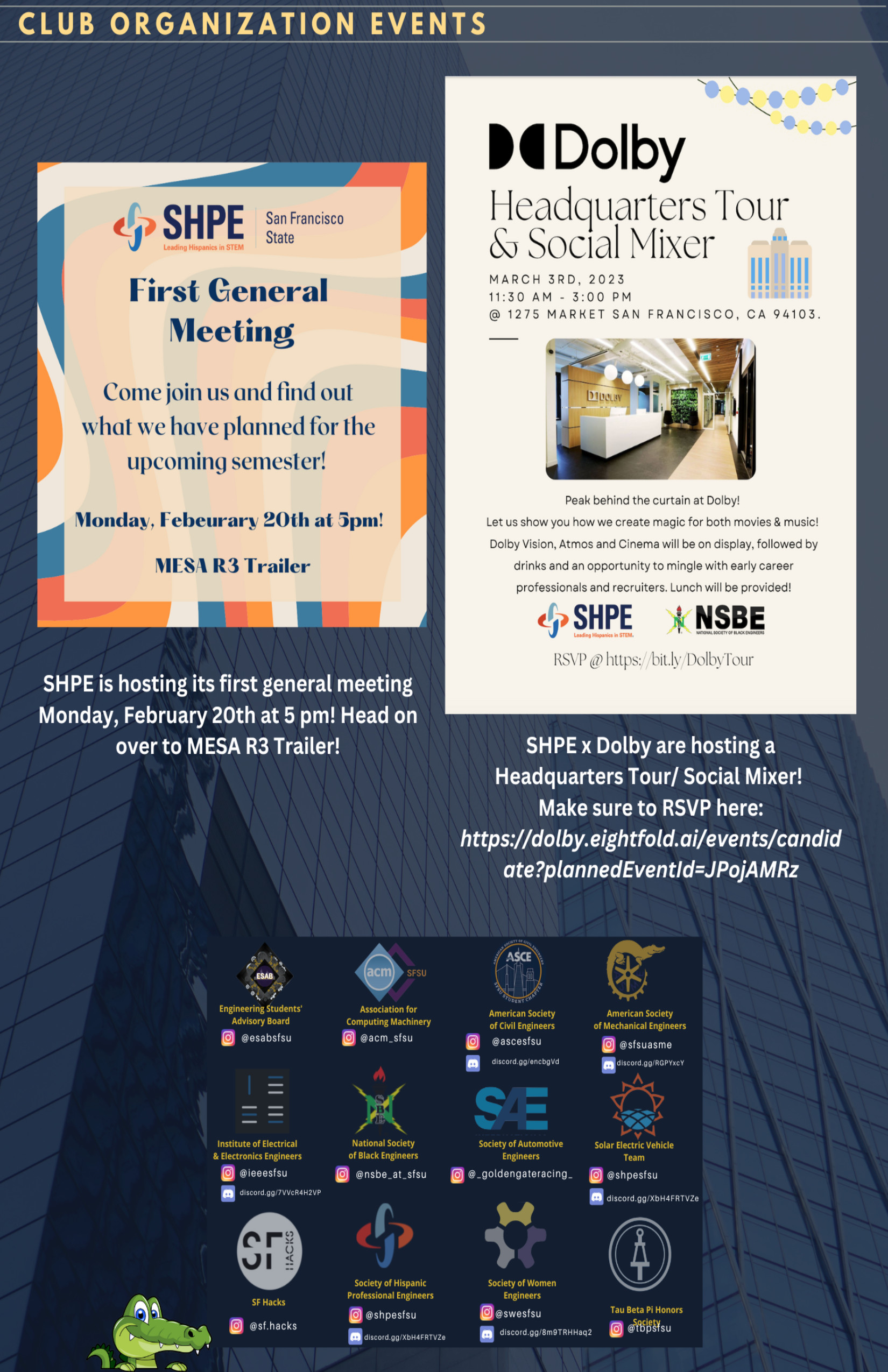
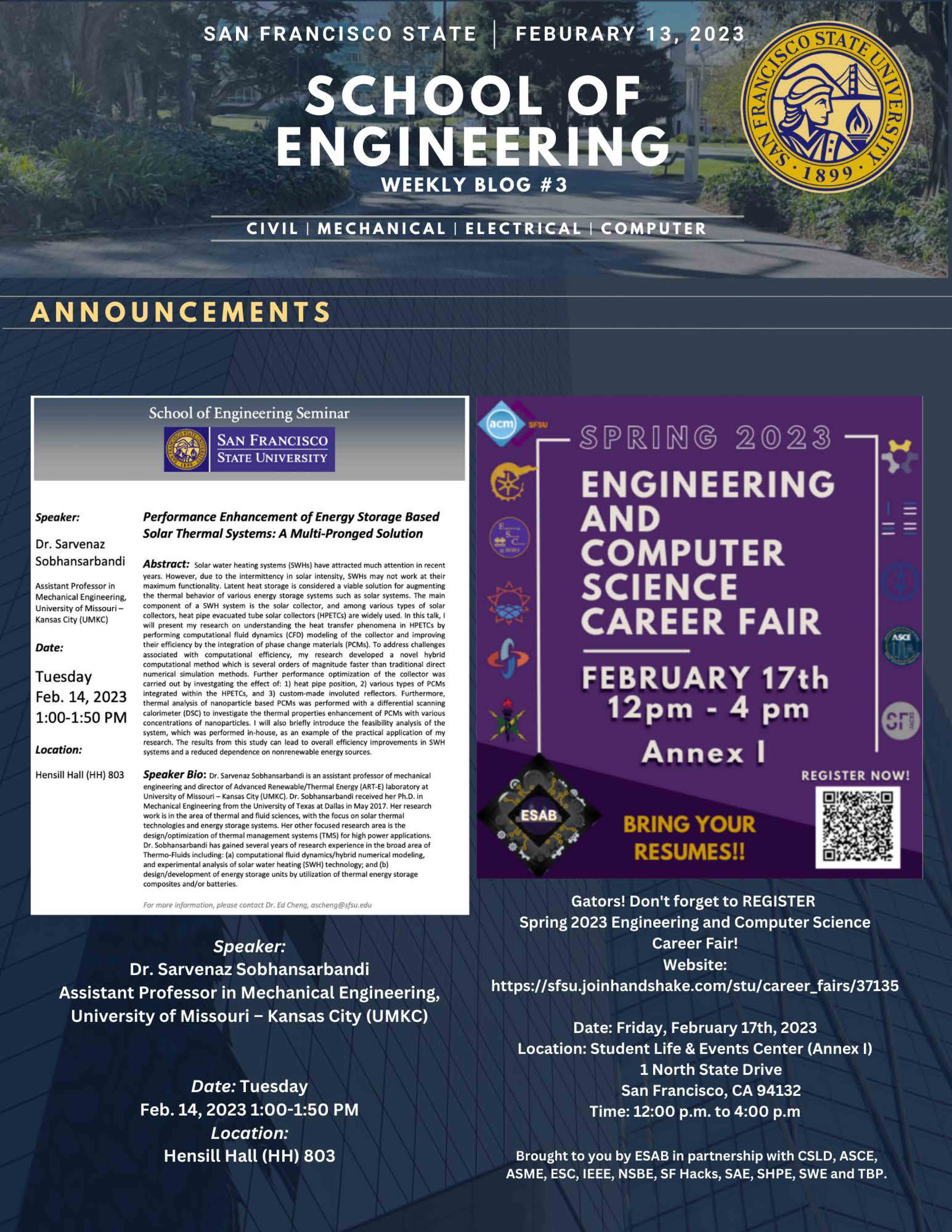
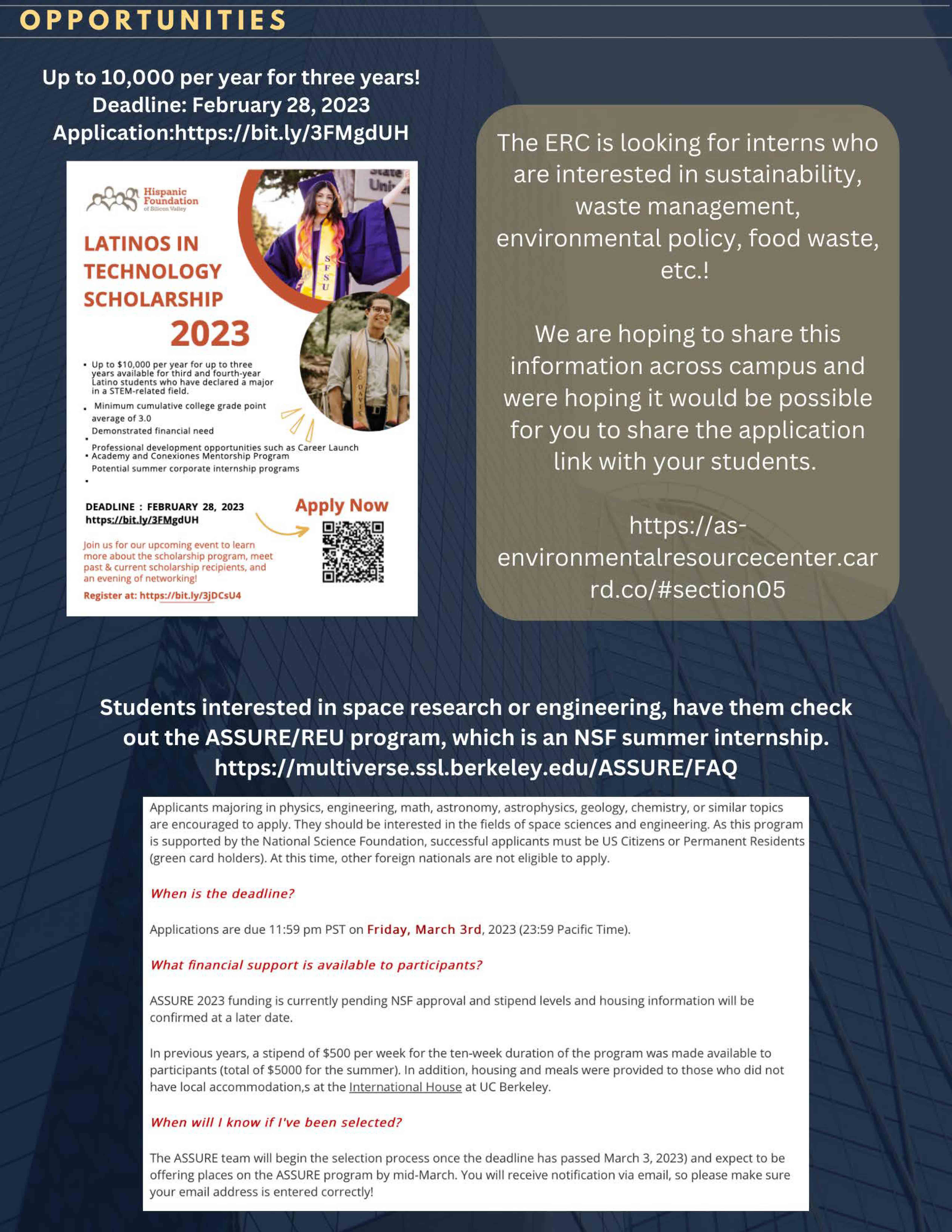
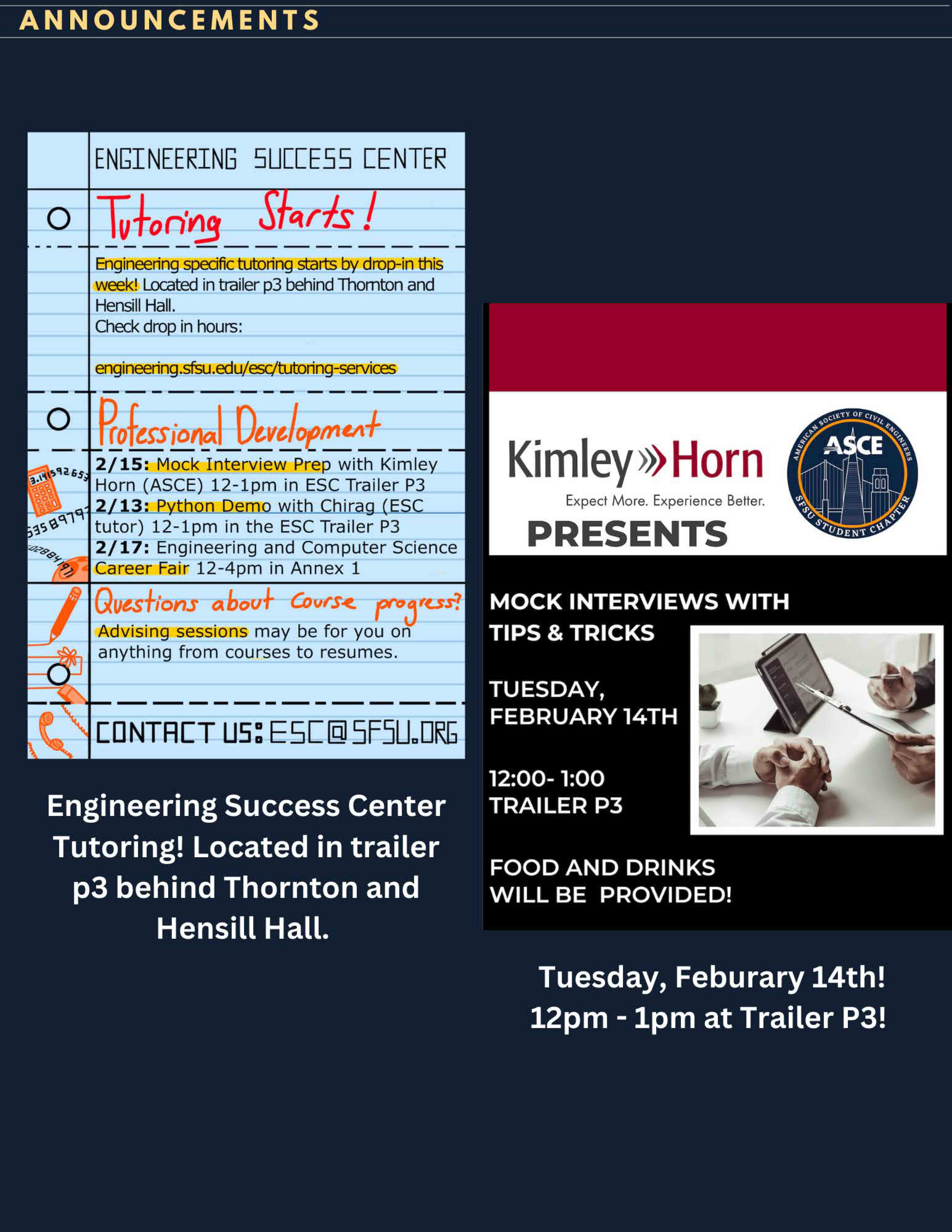
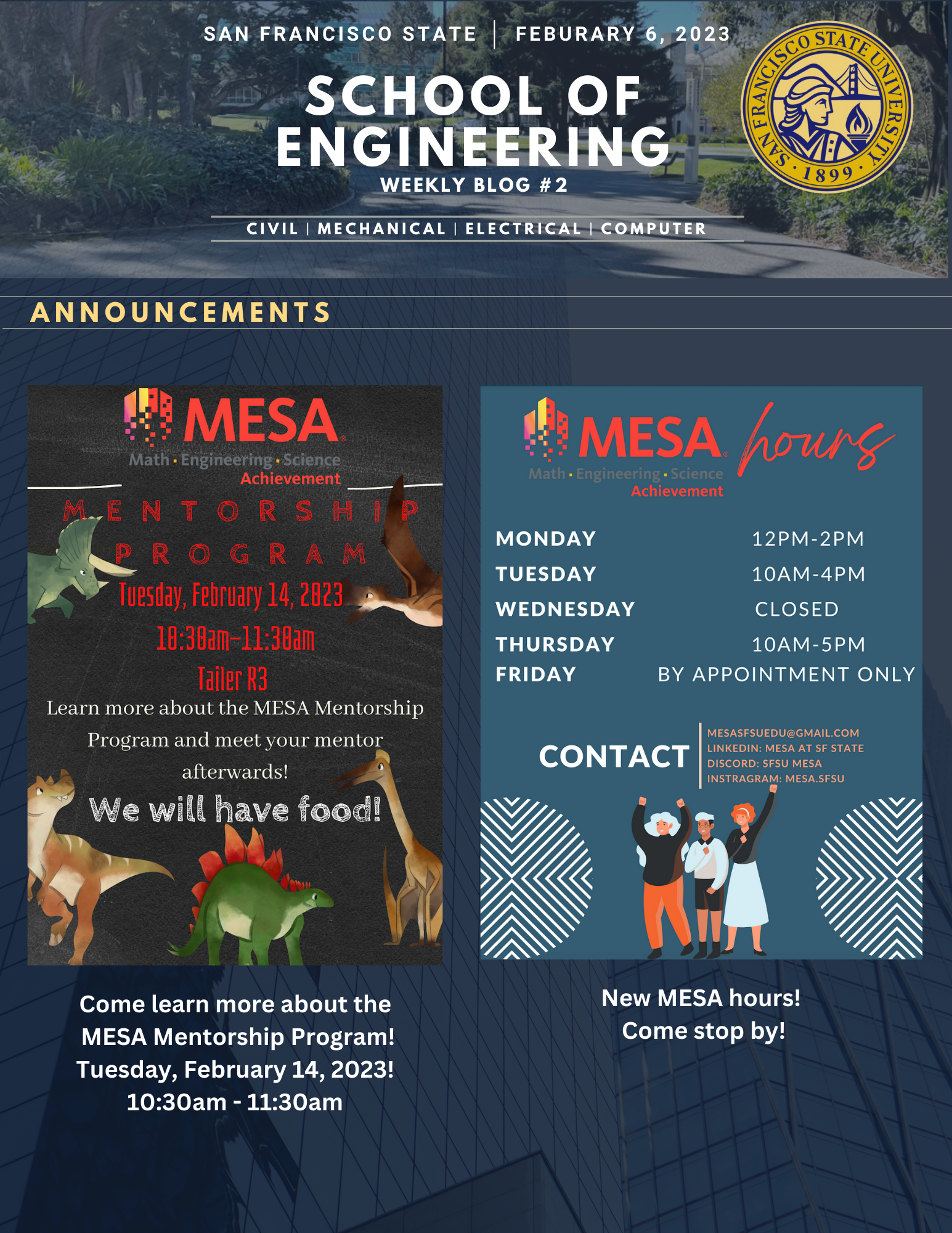
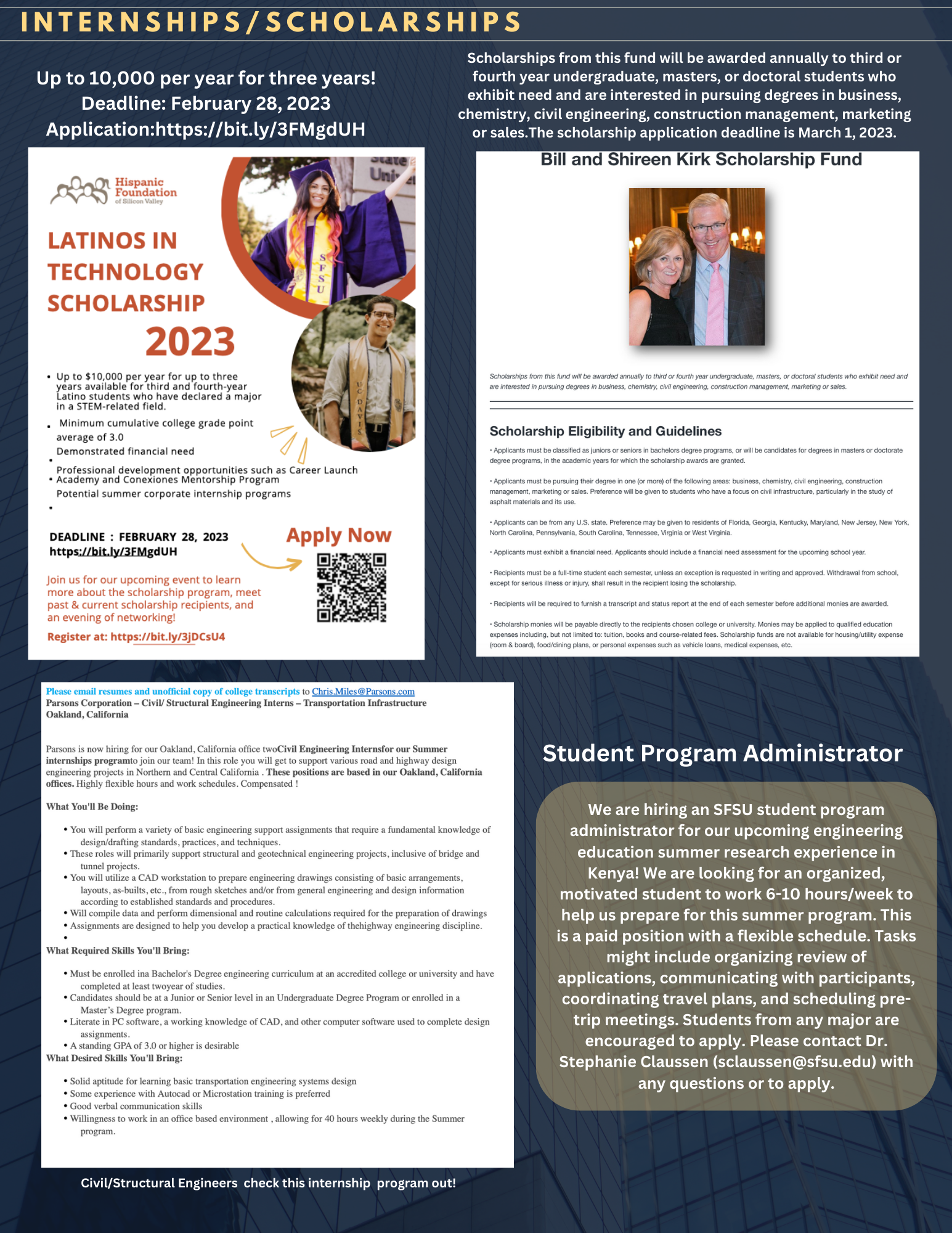
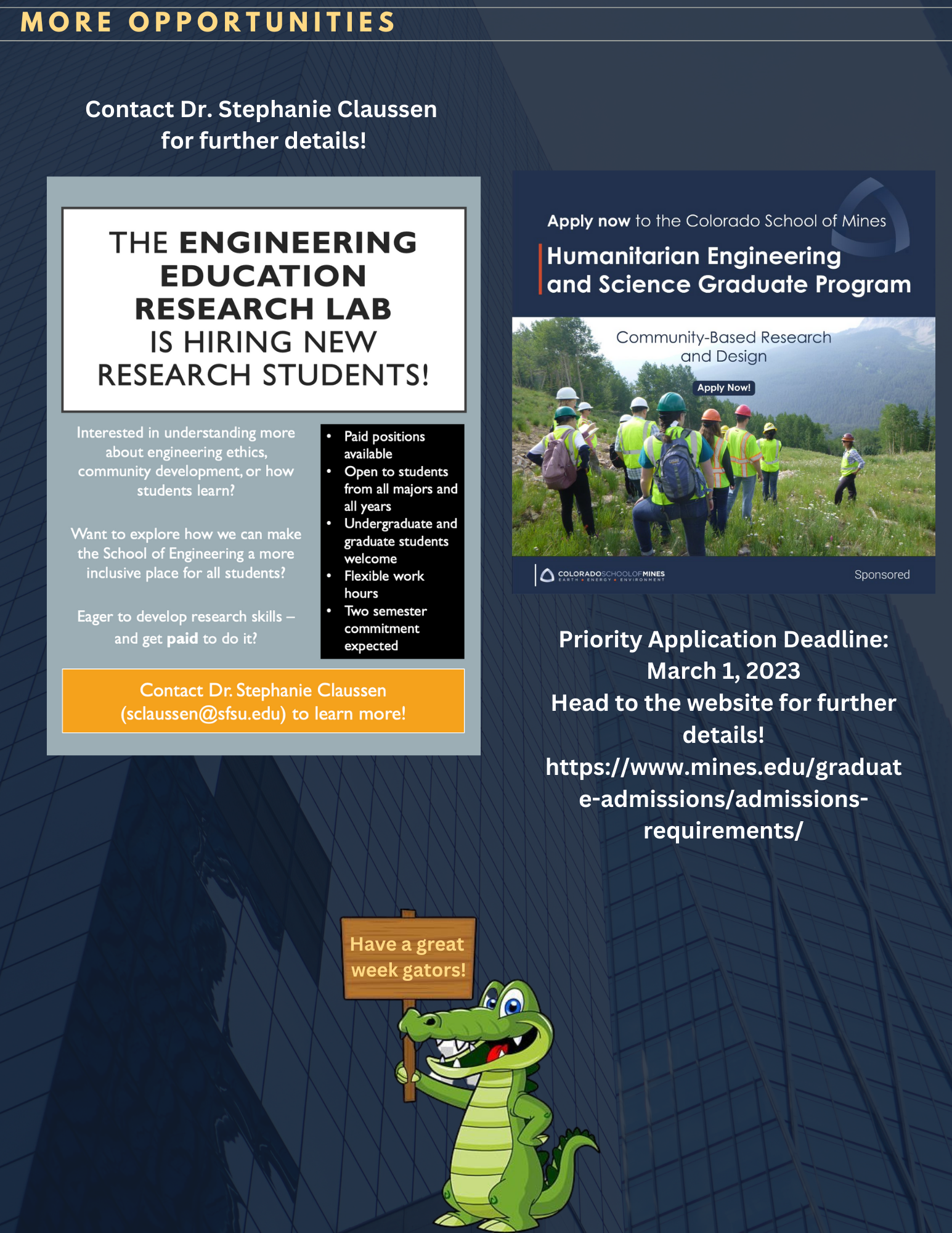
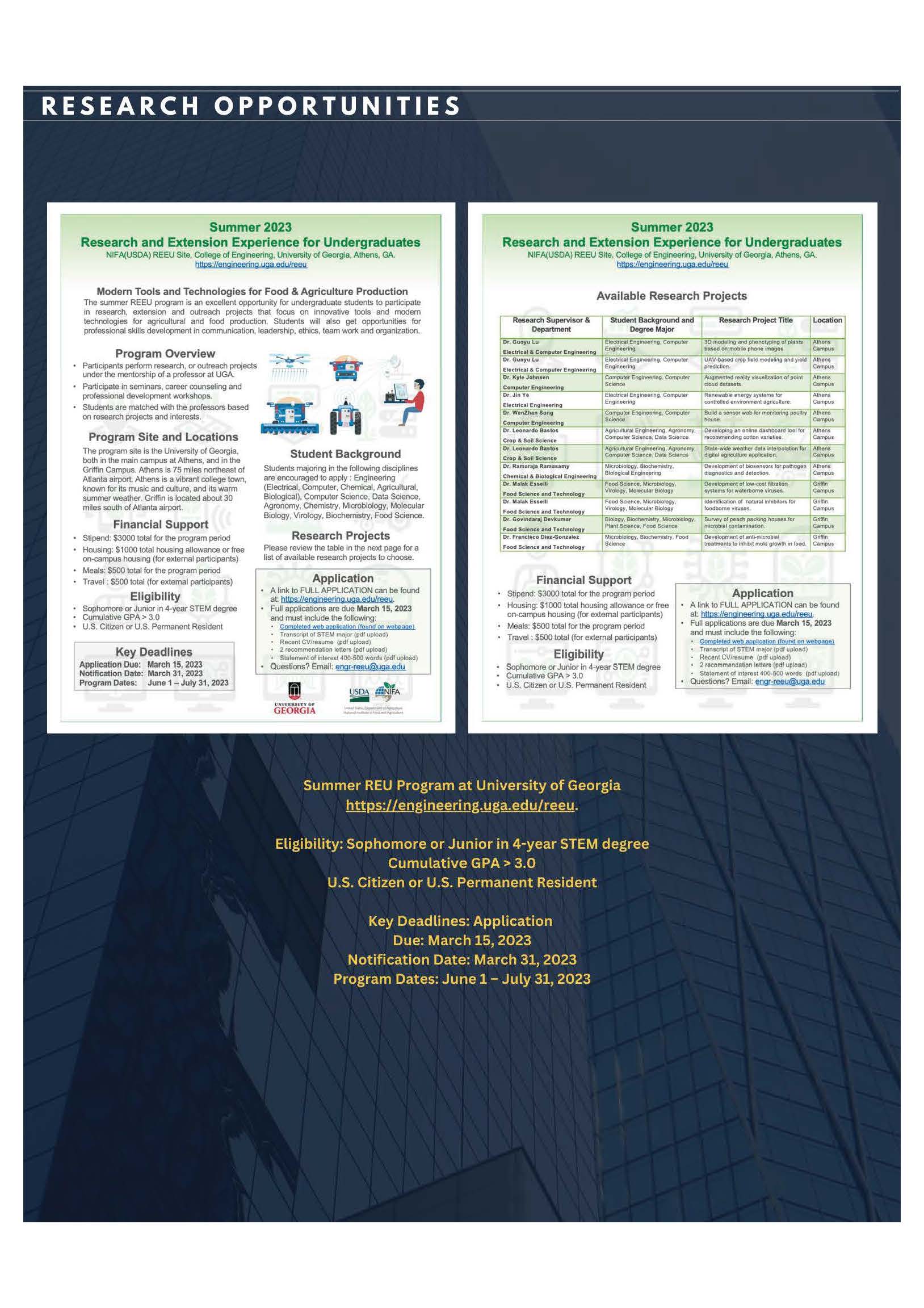
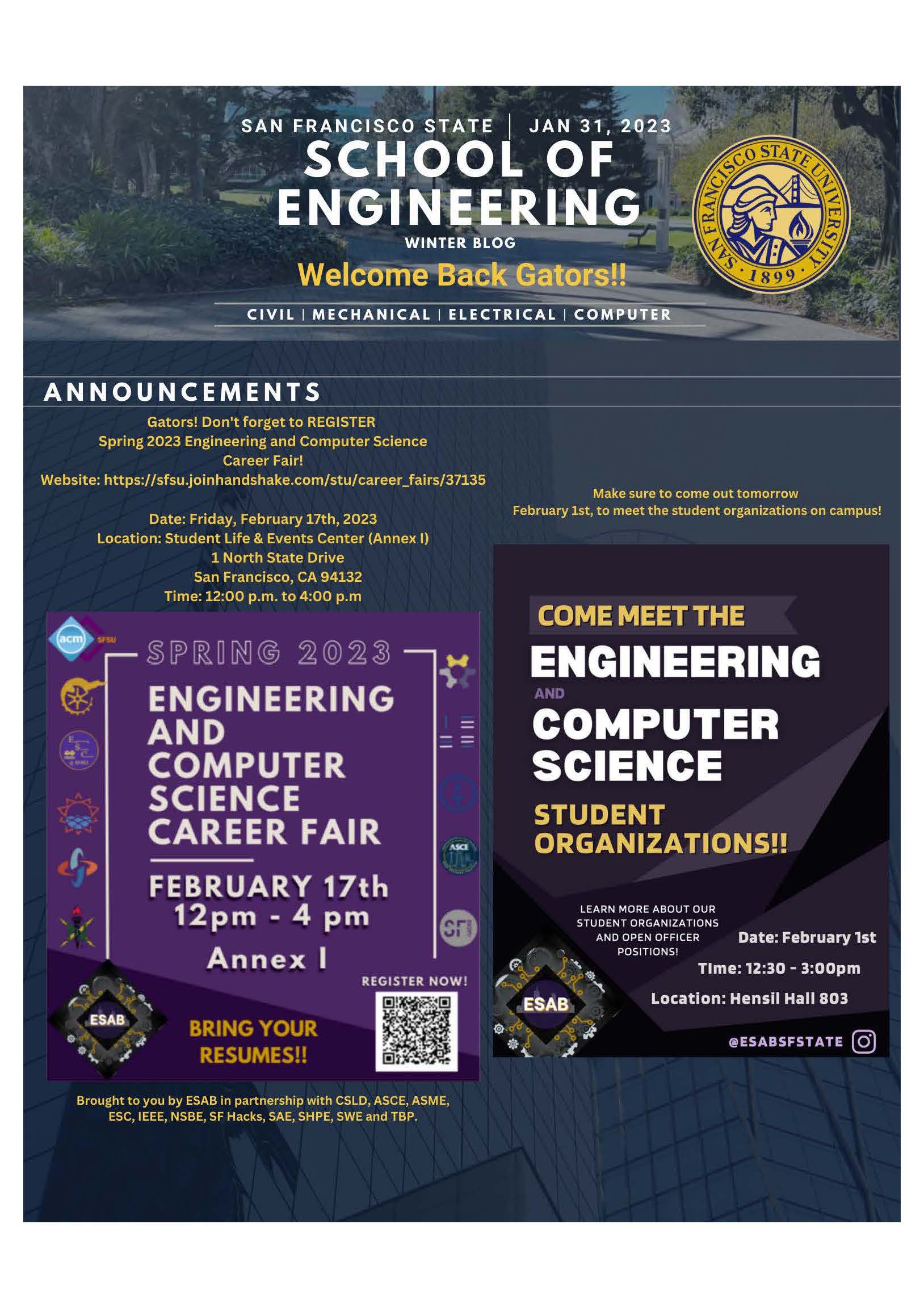
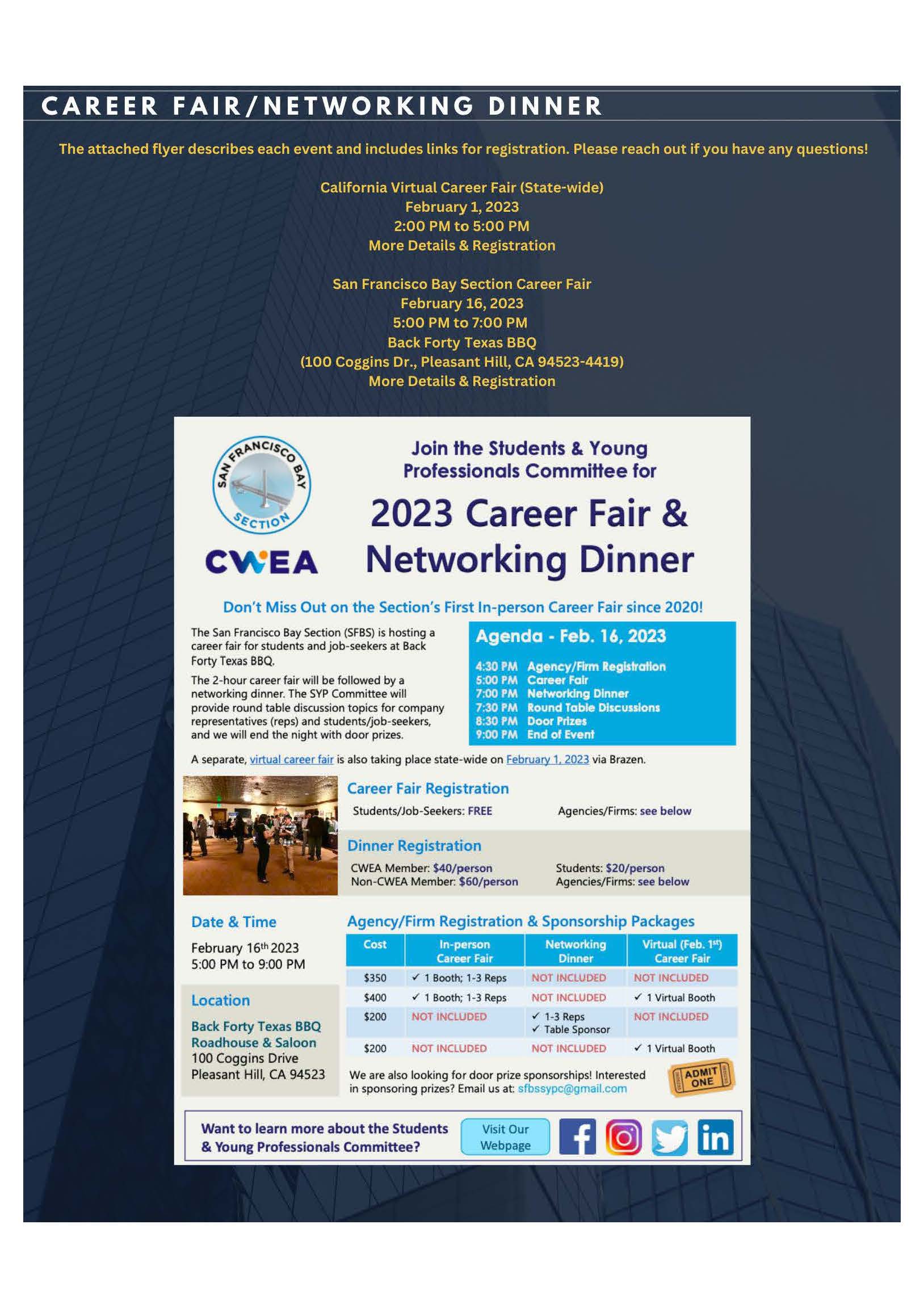
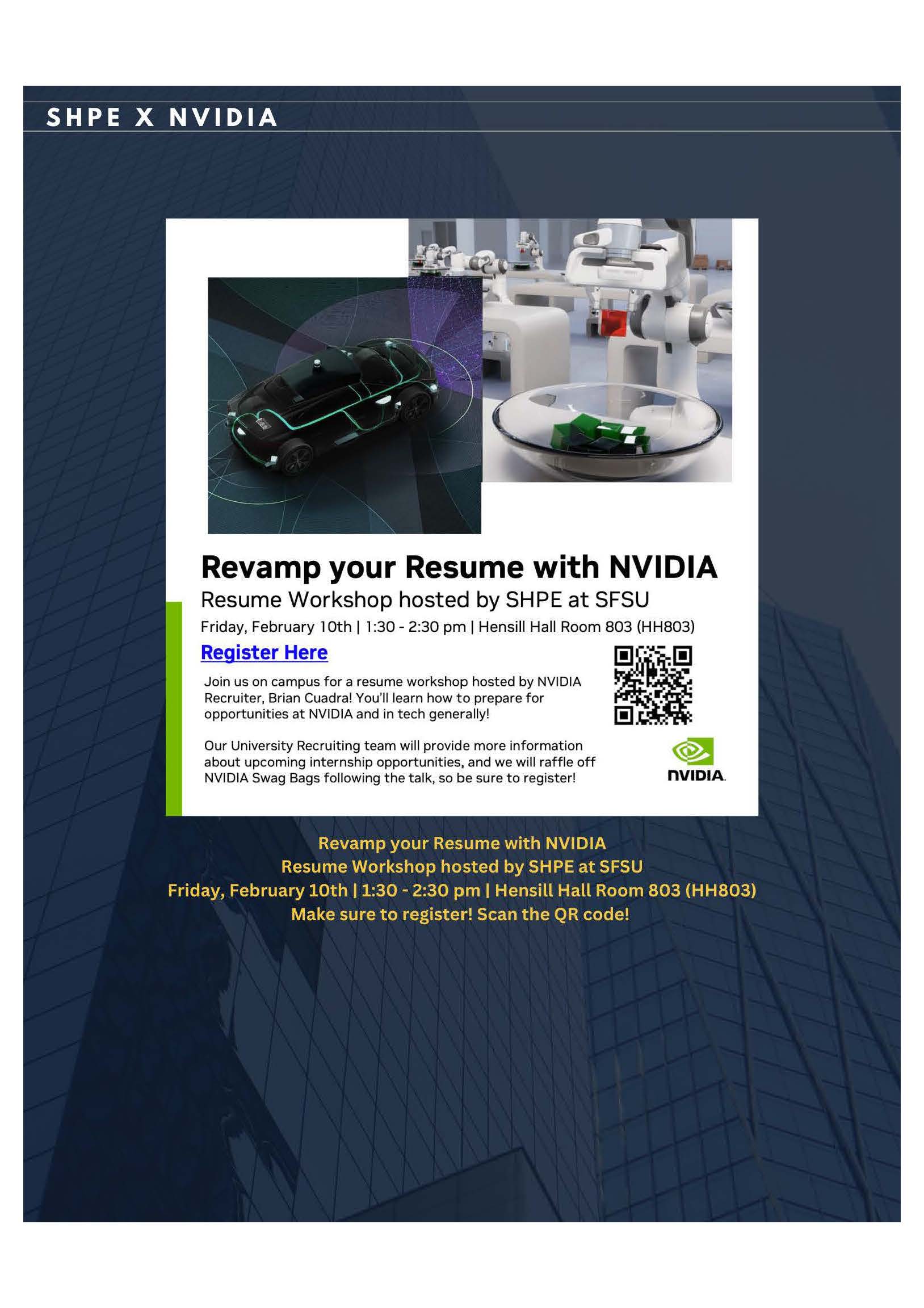
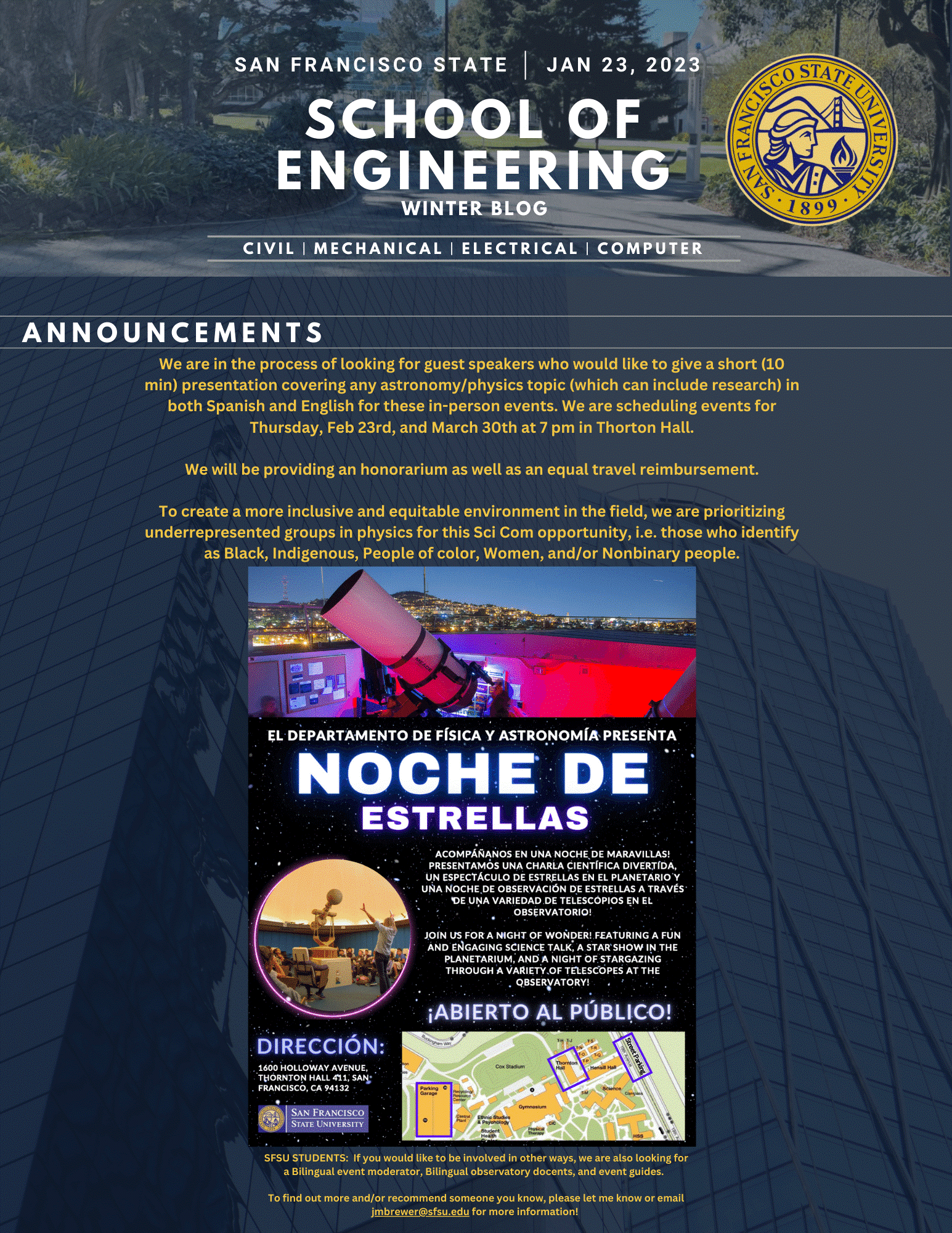
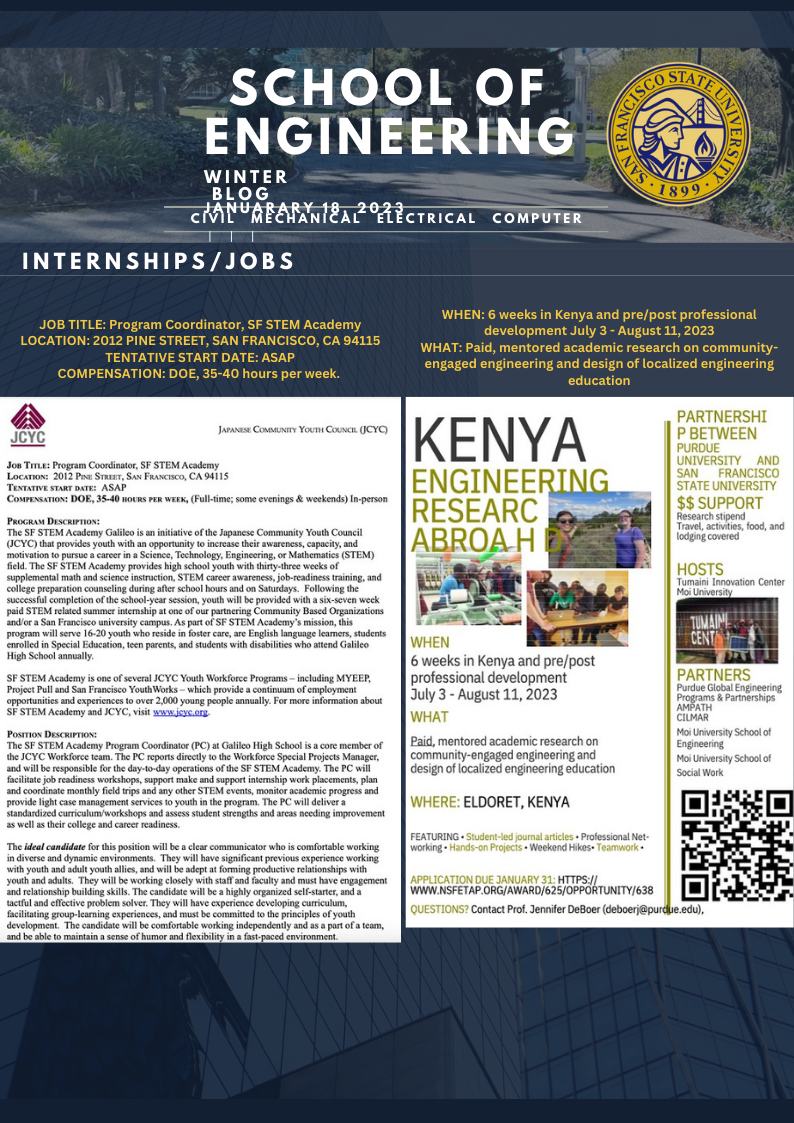
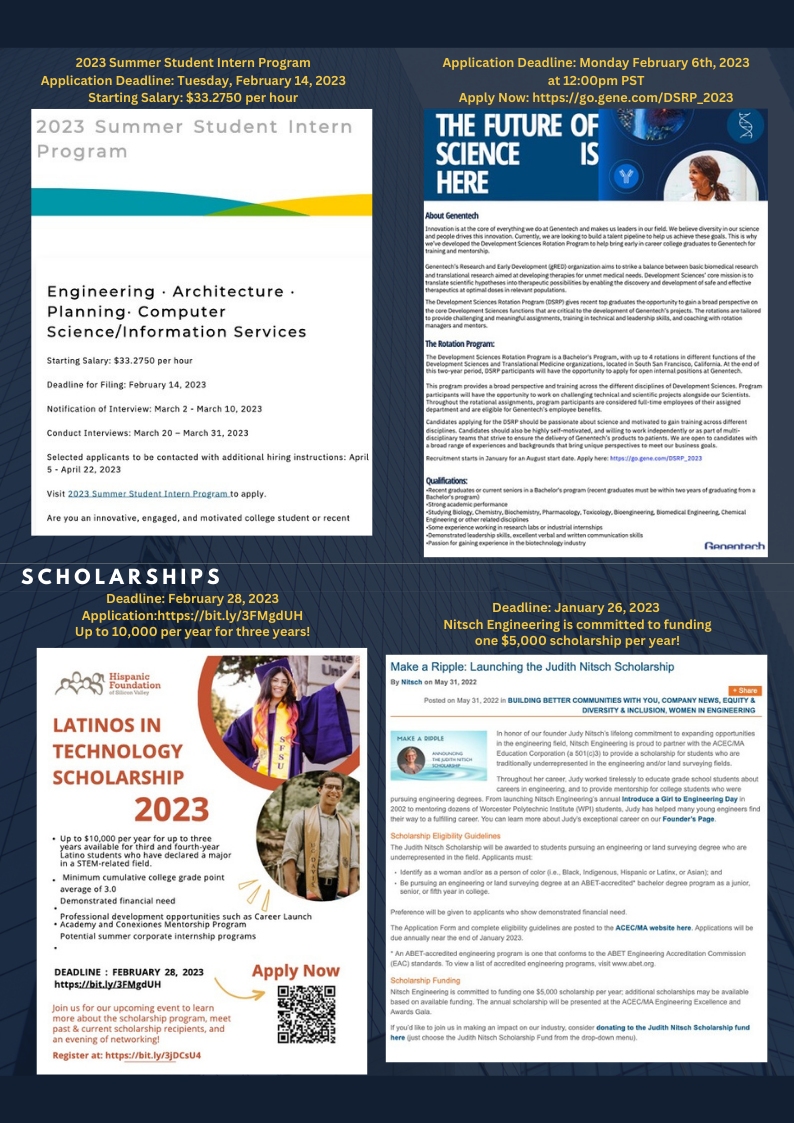
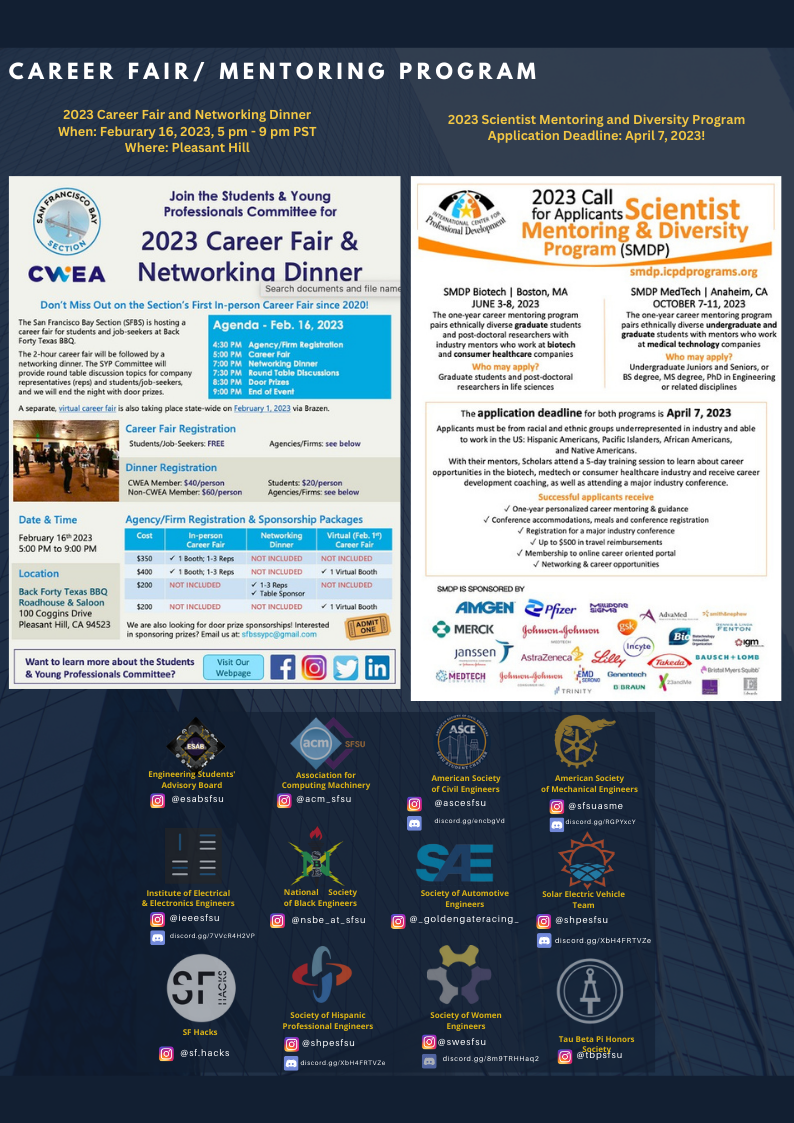